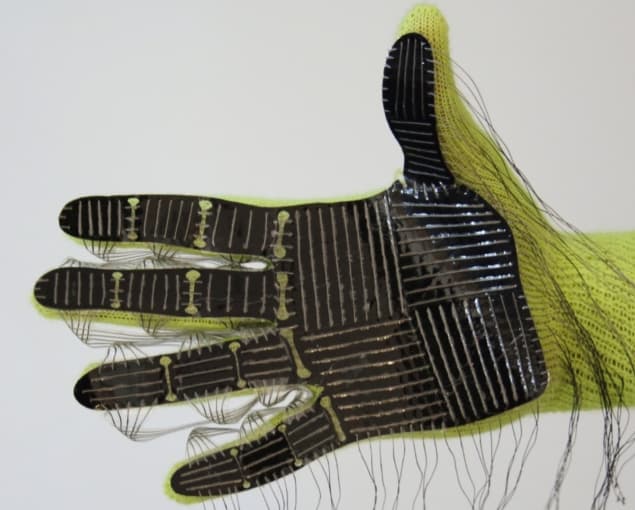
A glove that measures how a person grasps, feels and weighs objects has been unveiled by researchers at the Massachusetts Institute of Technology in the US. The low-cost device has hundreds of pressure sensors, which produce a sequence of high-resolution “pressure maps” when a wearer manipulates an object. The maps are fed into an artificial-intelligence system, which can recognize objects being held in the glove.
As well as providing important information about how the hand works, the research could also help with the design of prosthetic hands, robotic graspers and human-machine interfaces.
Created by Subramanian Sundaram and colleagues, the glove is made from a fabric that is coated with a polymer with an electrical resistance that changes when pressure is applied to it. The fingers, thumb and palm are crisscrossed by 64 conducting threads creating 548 junctions where the conductivity of the polymer – and hence the local pressure on the glove – can be measured. The data output of the glove is a 32×32 pixel “pressure map” that records the pressure at each pixel on grey scale of about 150 gradations. Data are acquired at a rate of 7.3 frames per second and the glove cost about $10 to make and readout electronics cost and additional $100.
Tactile database
The glove was used to acquire a “tactile database” of 135,000 pressure maps. This was done by having the wearer manipulate 26 everyday objects in one hand in sessions that lasted 3-5 min. The items included a pair of scissors, a roll of tape, a mug and a drinks can.
To identify objects, the team created a convolutional neural network (CNN), which is a type of artificial-intelligence system that is used to classify images. The CNN was designed to mimic how a person can identify an object by grasping in several different ways. Holding a roll of tape across its full diameter as well as grasping it by its much thinner ring, for example.
“Humans can identify and handle objects well because we have tactile feedback. As we touch objects, we feel around and realize what they are. Robots don’t have that rich feedback,” says Sundaram.
When evaluating an object, the CNN first groups similar pressure maps together into clusters – with each cluster corresponding to a specific grasp of the object. Then, a representative map from each of the clusters is selected to create a set of maps that are associated with a specific object. Finally, the CNN is trained to recognize a manipulated object by comparing the object’s pressure maps to the sets of representative maps.
Pressure drop
To estimate the weight of an object, the team created a separate data set of 11,600 pressure maps that were acquired while objects where picked-up, held and then dropped. In this case the CNN was trained to calculate the weight of the object from the pressure required to hold it.
According to the team, the system is able to recognize objects being manipulated with an accuracy of 76% and determine their weights to within about 60 g.
The glove also provided insights into how different parts of the hand work together to perform certain tasks. They found that when a subject uses the middle joint of their index finger, for example, they rarely use their thumb. Conversely, they found that using the tips of the index and middle fingers always corresponds to thumb usage. “We quantifiably show, for the first time, that, if I’m using one part of my hand, how likely I am to use another part of my hand,” says Sundaram.
The research could help engineers mimic the function of the hand to create better prostheses and robotic graspers. Covering mechanical hands with tactile gloves could give the devices a sense of touch and allow them to operate in a more life-like manner. In addition, computer-vision algorithms could be adapted for use with the glove, leading to new technologies involving tactile sensing.
The glove is described in Nature.