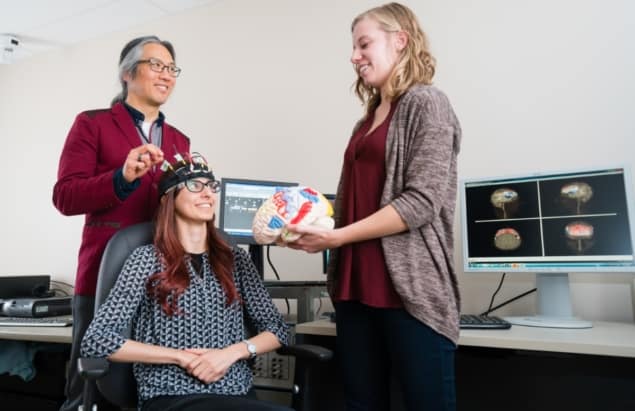
Brain–computer interfaces (BCIs) use cognitive activity to control an external device, enabling individuals with severe motor impairment to communicate. One BCI approach employs near-infrared spectroscopy (NIRS) to detect oxygen levels in the cerebral cortex, known as the haemodynamic signal, which is indicative of neuronal activity. The temporal resolution of this signal, however, is a few seconds, limiting the information transfer rate in NIRS-BCIs.
Another possibility may be to use NIRS to measure the fast optical signal (FOS) that arises from changes in optical scattering through cerebral tissue during neuronal activation. As the FOS response relates directly to neuronal activation (rather than the slow haemodynamic response that follows), its latency is around 100 ms. But despite this higher temporal resolution, the FOS has a low signal-to-noise ratio (SNR) and its reliability has been disputed. A team from Bloorview Research Institute has now investigated the use of the FOS to control a NIRS-BCI (Biomed. Phys. Eng. Express 4 065010).
“As it is believed that the FOS signal is related to neuronal firing, there is potential that evoked responses similar to those measured with electroencephalography [EEG] could be harnessed through optical measurements,” says senior author Tom Chau. “In theory, this could accelerate the response time of optical BCIs. A major limitation, however, is the need for trial averaging, which slows down the effective response time.”
Spotting Einstein
Fifteen study participants took part in three offline data collection sessions, during which they performed a visual oddball task. Each session included three runs of 10 task blocks, with 100 trials per block. In each trial, an image was presented on a screen for 512 ms, with oddball images (a photo of Einstein) interspersed randomly between frequent images of letters (A, B, C, D); 20% of images were oddballs. Fourteen subjects also attended two online sessions composed of one offline run, and two online runs during which they received classification feedback.

Chau and colleagues recorded NIRS measurements over the prefrontal cortex, using laser diode sources and detectors placed in a custom headband on the participant’s forehead. They used five paired 690 nm and 830 nm sources and four detectors, enabling 11 measurement channels of each wavelength. To validate FOS responses, they performed simultaneous EEG measurements of event-related potentials in two sessions, using electrodes on the NIRS headband.
“As there has been some debate about whether optical measurements can detect evoked responses, we used EEG to confirm that indeed we were eliciting an evoked response,” explains Chau. “Also, the EEG signals provided a reference against which we could compare the FOS data.”
Automation algorithm
The team then examined the feasibility of automatically classifying the FOS response in the online sessions, using a classification algorithm trained on data from the four offline sessions. They used temporal and spectral features extracted from DC intensity and phase delay data, at 690 and 830 nm, to classify the FOS response, using a weighted majority vote of support vector machine and linear discriminant analysis classifiers.
Examining averaged responses from 15 trials offline, the researchers found that FOS response could differentiate oddball and frequent images with an average sensitivity, specificity and balanced accuracy (average of sensitivity and specificity) of 63%, 60% and 62%, respectively. They note that these offline FOS classification results were significantly above chance for each participant, confirming that automatic detection of the FOS is possible at above-chance levels.
Individual classifier results were significantly higher for DC intensity feature sets than for phase delay feature sets for nine and 10 participants, at 690 and 830 nm, respectively. Classifiers of 830 nm feature sets also had significantly better results than 690 nm sets for half of the participants. The authors propose that FOS sensitivity is higher at 830 nm because increased absorption at 690 nm reduces the SNR.

The EEG-measured event-related potentials were classified using a similar algorithm, and seen to differentiate images with an average sensitivity, specificity and balanced accuracy of 82%, 72% and 77%, respectively. This confirmed that the prefrontal neuronal response to a visual oddball task could be classified above the level required for effective BCI communication (70%).
Chau and colleagues also classified the FOS 15-trial averages online during the two online sessions. Here, the FOS exhibited an average sensitivity, specificity and balanced accuracy of 64%, 63% and 63%, respectively. The online classification results were also significantly better than chance in both online sessions for seven participants and in one session for five.

The Cybathlon challenge
For this first reported attempt to automatically classify the FOS online, the average online balanced accuracy across all participants was 63±6%. While this accuracy does not meet the required 70% level, the authors suggest that adding measurement channels across the fronto-central and parietal areas of the brain, where a larger FOS response may be found, should improve the SNR.
“Future work will look for ways to improve the strength of the response and thereby reduce the number of trials that need to be averaged,” Chau tells Physics World. “For now, the FOS is not yet ready for deployment in a practical BCI.”