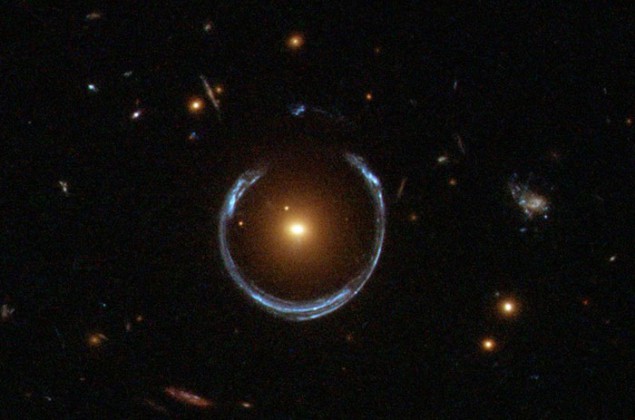
A new and much faster way of analysing the gravitational lensing of light has been developed by physicists at Stanford University in the US. The technique is 10 million times faster than conventional methods and uses artificial neural networks. It could make it possible for astronomers to rapidly analyse images of tens of thousands of gravitational lenses that are expected to be discovered by the next generation of telescopes.
Gravitational lensing occurs when light from a distant object is bent by the gravitational field of a galaxy or other massive object that the light passes on its journey to Earth. This leads to the distortion of the image of the distant object – often as multiple images of the distant object or a ring of light. Careful analysis of the distortion can reveal important information about the distribution of matter and dark matter in the universe.
Just a few seconds
Analysing gravitational lenses is a time-consuming process that involves comparing the observed image to a large number of computer simulations to find a match. This can take several months for a single image. Using artificial neural networks, Yashar Hezaveh, Laurence Perreault Levasseur and Philip Marshall say they can perform the same analysis in just a few seconds.
Artificial neural networks simulate the human brain, which comprises neurons that are connected in a network by synapses. Like their living counterparts, these artificial networks are very good at learning how to do a task when given many examples of how the job should be done.
Training day
The trio trained their neural networks by inputting half a million simulated images of gravitational lenses – each corresponding to specific lens properties and matter distribution. This process took about a day, but once completed the system was able to analyse new lenses almost instantaneously, say the researchers.
“The neural networks we tested – three publicly available neural nets and one that we developed ourselves – were able to determine the properties of each lens, including how its mass was distributed and how much it magnified the image of the background galaxy,” explains Hezaveh.
What’s more, the analysis requires no special computing facilities and the team says it can be done on a PC or even on a smartphone.
Tens of thousands
Writing in Nature, the researchers point out that the technique could be indispensable for dealing with the plethora of lensing images that are expected from the next generation of telescopes such as the Large Synoptic Survey Telescope (LSST). Scheduled for completion in 2019, the Chile-based LSST will have a 3.2-gigapixel camera and should increase the number of known strong gravitational lenses from a few hundred today to tens of thousands.
“We won’t have enough people to analyse all these data in a timely manner with the traditional methods,” says Perreault Levasseur. “Neural networks will help us identify interesting objects and analyse them quickly. This will give us more time to ask the right questions about the universe.”