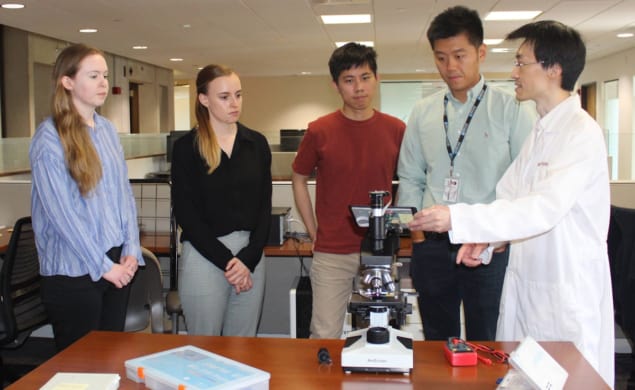
Colorectal cancer (CRC) is the second most common cause of cancer death in the United States, leading to about 53,000 deaths each year. CRC is a complex and multifaceted disease that poses significant challenges in diagnosis, prognosis and treatment selection. In a study reported in Nature Communications, Kun-Hsing Yu and his team at Harvard Medical School developed the Multi-omics Multi-cohort Assessment (MOMA) system, a machine learning framework for the prediction of prognosis in colorectal cancer patients.
The researchers showed that histopathology images can be used as reliable predictors of multi-omic aberrations: genomic, epigenomic, transcriptomic and proteomic abnormalities. “These findings hold great promise for improving patient outcomes and changing the way we approach colon cancer treatment,” says Yu.
Current methods for assessing CRC prognosis include a combination of histopathological, genomic and clinical data analysis, with histopathologic examination remaining the gold standard for diagnosis. Treatment selection for CRC is based on histology subtypes and genetic variations. Inter-rater variations in histopathological diagnosis have been reported, however, and the genomic analysis process can take days or even weeks to complete, in addition to not being available in all clinics in developing countries. Such limitations may prevent CRC patients from receiving timely and appropriate treatments.
Yu and his research team recognized the inability of human analysis of histopathology images to unlock valuable information about the disease. MOMA uses advanced image analysis techniques and machine learning (ML) algorithms to bridge the gap between the visual inspection of tumours and the molecular aberrations found in tumour cells. The ML framework successfully predicts the prognoses of early-stage colorectal cancer patients and identifies the genomics and proteomics status of cancer samples using histopathology images.
The researchers developed a deep-learning model that can extract features and patterns from digitized histopathology images, enabling the identification of important cell features associated with certain conditions. They trained their model using information obtained from 1888 colorectal cancer patients from diverse populations.
In tests on unseen images, the histopathology-based model showed remarkable accuracy in predicting a wide variety of mutations, including genomic alterations and transcriptomic variations. In addition, the AI model predicted patients’ survival outcomes, pointing to the potential of histopathology image features as prognostic indices.
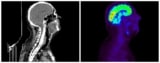
‘Multifaceted radiomics’ predicts cancer metastasis risk
The team suggests that the ML model could also facilitate the development of personalized medicine, with the integration of histopathology images and quantitative omics data opening exciting avenues for personalized cancer treatments. This approach holds great promise for optimizing treatment options, minimizing side effects and improving overall patient outcomes.
With the development of sophisticated algorithms and image processing techniques, AI tools for histopathology image analyses can be integrated into routine clinical practice. In the future, oncologists, pathologists and other healthcare professionals could use this valuable tool to improve diagnostic accuracy, predict response to treatment and tailor treatments for colorectal cancer patients.