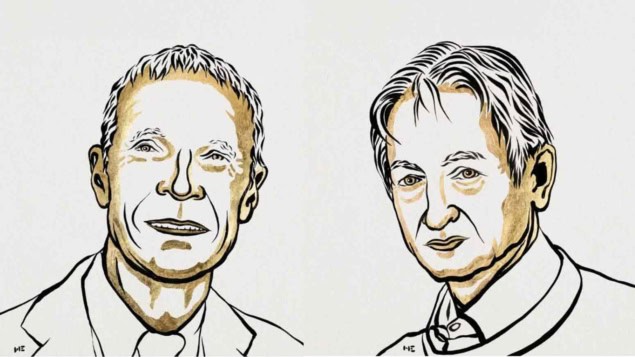
John Hopfield and Geoffrey Hinton share the 2024 Nobel Prize for Physics for their “foundational discoveries and inventions that enable machine learning and artificial neural networks”. Known to some as the “godfather of artificial intelligence (AI)”, Hinton, 76, is currently based at the University of Toronto in Canada. Hopfield, 91, is at Princeton University in the US.
Ellen Moons from Karlstad University, who chairs the Nobel Committee for Physics, said at today’s announcement in Stockholm: “This year’s laureates used fundamental concepts from statistical physics to design artificial neural networks that function as associative memories and find patterns in large data sets. These artificial neural networks have been used to advance research across physics topics as diverse as particle physics, materials science and astrophysics.”
Speaking on the telephone after the prize was announced, Hinton said, “I’m flabbergasted. I had no idea this would happen. I’m very surprised”. He added that machine learning and artificial intelligence will have a huge influence on society that will be comparable to the industrial revolution. However, he pointed out that there could be danger ahead because “we have no experience dealing with things that are smarter than us.”
“Two kinds of regret”
Hinton admitted that he does have some regrets about his work in the field. “There’s two kinds of regret. There’s regrets where you feel guilty because you did something you knew you shouldn’t have done. And then there are regrets where you did something that you would do again in the same circumstance but it may in the end not turn out well. That second kind of regret I have. I am worried the overall consequence of this might be systems more intelligent than us that eventually take control.”
Hinton spoke to the Nobel press conference from the West Coast of the US, where it was about 3 a.m. “I’m in a cheap hotel in California that doesn’t have a very good Internet connection. I was going to get an MRI scan today but I think I’ll have to cancel it.”
Hopfield began his career as a condensed-matter physicist before making the shift to neuroscience. In a 2014 perspective article for the journal Physical Biology called “Two cultures? Experiences at the physics–biology interface”, Hopfield wrote, “Mathematical theory had great predictive power in physics, but very little in biology. As a result, mathematics is considered the language of the physics paradigm, a language in which most biologists could remain illiterate.” Hopfield saw this as an opportunity because the physics paradigm “brings refreshing attitudes and a different choice of problems to the interface”. However, he was not without his critics in the biology community and wrote that one must have “have a thick skin”.
In the early 1980s, Hopfield developed his eponymous network, which can be used to store patterns and then retrieve them using incomplete information. This is called associative memory and an analogue in human cognition would be recalling a word when you only know the context and maybe the first letter or two.
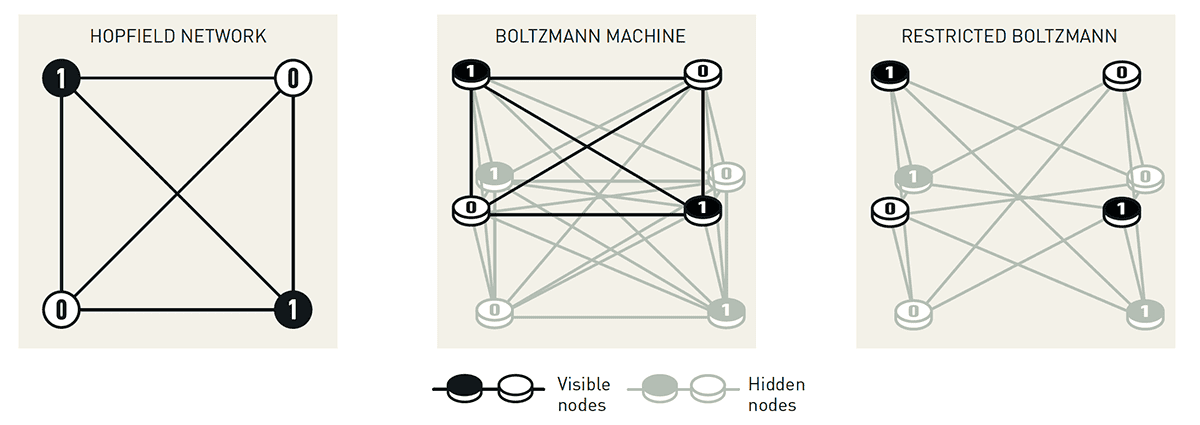
A Hopfield network is layer of neurons (or nodes) that are all connected together such that the state, 0 or 1, of each node is affected by the states of its neighbours (see above). This is similar to how magnetic materials are modelled by physicists – and a Hopfield network is reminiscent of a spin glass.
When an image is fed into the network, the strengths of the connections between nodes are adjusted and the image is stored in a low-energy state. This minimization process is essentially learning. When an imperfect version of the same image is input, it is subject to an energy-minimization process that will flip the values of some of the nodes until the two images resemble each other. What is more, several images can be stored in a Hopfield network, which can usually differentiate between all of them. Later networks used nodes that could take on more than two values, allowing more complex images to be stored and retrieved. As the networks improved, evermore subtle differences between images could be detected.
A little later on in the 1980s, Hinton was exploring how algorithms could be used to process patterns in the same way as the human brain. Using a simple Hopfield network as a starting point, he and a colleague borrowed ideas from statistical physics to develop a Boltzmann machine. It is so named because it works in analogy to the Boltzmann equation, which says that some states are more probable than others based on the energy of a system.
A Boltzmann machine typically has two connected layers of nodes – a visible layer that is the interface for inputting and outputting information, and a hidden layer. A Boltzmann machine can be generative – if it is trained on a set of similar images, it can produce a new and original image that is similar. The machine can also learn to categorise images. It was realized that the performance of a Boltzmann machine could be enhanced by eliminating connections between some nodes, creating “restricted Boltzmann machines”.
Hopfield networks and Boltzmann machines laid the foundations for the development of later machine learning and artificial-intelligence technologies – some of which we use today.
A life in science
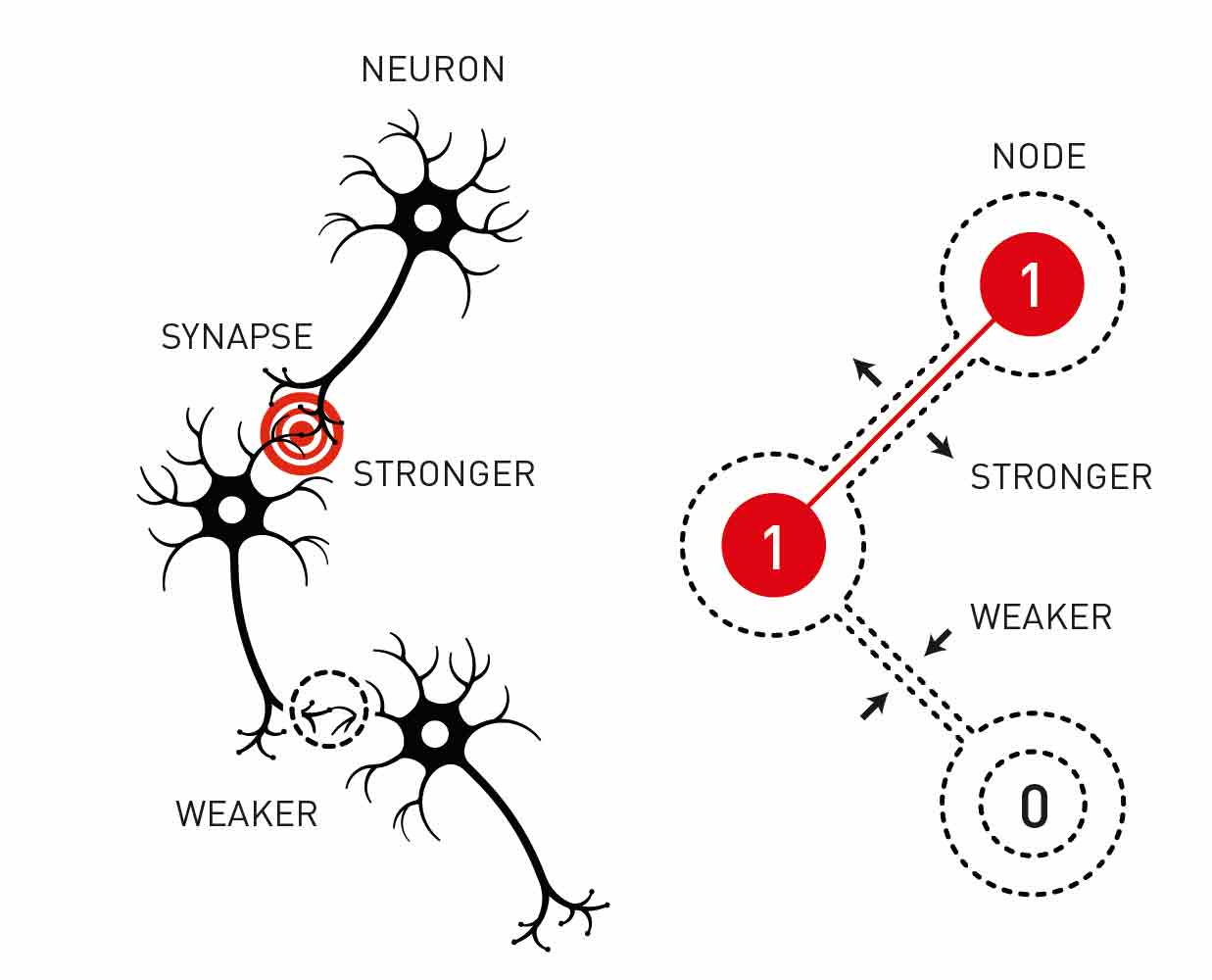
Born on 6 December 1947 in London, UK, Hinton graduated with a degree in experimental psychology in 1970 from Cambridge University before doing a PhD on AI at the University of Edinburgh, which he completed in 1975. After a spell at the University of Sussex, Hinton moved to the University of California, San Diego, in 1978, before going toCarnegie-Mellon University in 1982 and Toronto in 1987.
After becoming a founding director of the Gatsby Computational Neuroscience Unit at University College London in 1998, Hinton returned to Toronto in 2001 where he has remained since. From 2014, Hinton divided his time between Toronto and Google but then resigned from Google in 2023 “to freely speak out about the risks of AI.”
Elected as a Fellow of the Royal Society in 1998, Hinton has won many other awards including the inaugural David E Rumelhart Prize in 2001 for the application of the backpropagation algorithm and Boltzmann machines. He also won the Royal Society’s James Clerk Maxwell Medal in 2016 and the Turing Award from the Association for Computing Machinery in 2018.
Hopfield was born on 15 July 1933 in Chicago, Illinois. After receiving a degree in 1954 from Swarthmore College in 1958 he completed a PhD in physics at Cornell University. Hopfield then spent two years at Bell Labs before moving to the University of California, Berkeley, in 1961.
In 1964 Hopfield went to Princeton University and then in 1980 moved to the California Institute of Technology. He returned to Princeton in 1997 where he remained for the rest of his career.
As well as the Nobel prize, Hopfield won the 2001 Dirac Medal and Prize from the International Center for Theoretical Physics as well the Albert Einstein World Award of Science in 2005. He also served as president of the American Physical Society in 2006.
- Two papers written by this year’s physics laureates in journals published by IOP Publishing, which publishes Physics World, can be read here.
- The Institute of Physics, which publishes Physics World, is running a survey gauging the views of the physics community on AI and physics till the end of this month. Click here to take part.
SmarAct proudly supports Physics World‘s Nobel Prize coverage, advancing breakthroughs in science and technology through high-precision positioning, metrology and automation. Discover how SmarAct shapes the future of innovation at smaract.com.