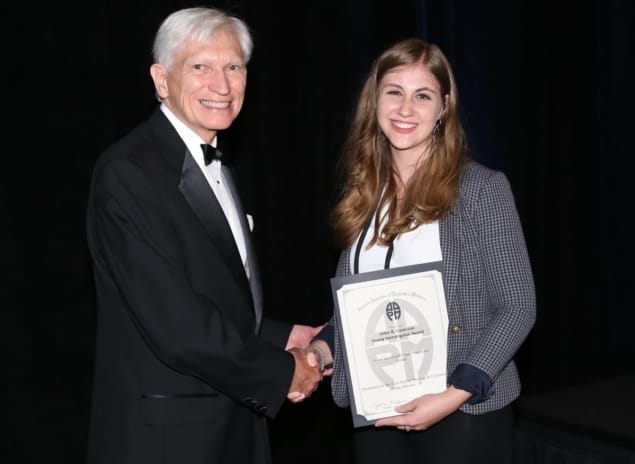
Each year, the AAPM Annual Meeting holds a Young Investigator competition. The 10 submitted abstracts scored the highest by the reviewers were presented by the finalists in a dedicated symposium, held in honour of University of Wisconsin professor emeritus John R Cameron. The top three winners of the competition received their awards at a ceremony later that day. Here’s what the winners had to say.
Ultrasound guidance improves brachytherapy accuracy
The winner of the 2019 John R Cameron Young Investigator award was Jessica Rodgers from the Robarts Research Institute. Rodgers is developing a 3D ultrasound needle-guidance system to help improve high dose-rate (HDR) interstitial gynaecologic brachytherapy.
Gynaecologic cancers have diverse presentation, Rodgers explained, occur in challenging anatomic locations and exhibit highly variable disease geometry. One treatment option for such cancers is HDR interstitial brachytherapy, in which needles are inserted into the tumour and then a radioactive source is positioned within the needle channels to precisely irradiate the tumour target.
Accurate placement of the needles is essential to avoid delivering excess dose to nearby organs-at-risk (OARs), such as the bladder or rectum. Currently, guidance is achieved using an initial MR image, followed by post-insertion CT or MRI to verify needle placement. What’s needed, Rodgers told the audience, is a way to visualize the needles while they are being placed. Such intra-operative guidance, using 3D ultrasound, for example, should improve implant quality and reduce risk to OARs.
To account for the variability of gynaecologic cancer geometry and patient anatomy, Rodgers and colleagues have created an ultrasound device with three scanning modes: side-fire transrectal ultrasound (TRUS) with 170° probe rotation, side-fire transvaginal ultrasound (TVUS) with 360° rotation, and end-fire TVUS.
They tested the TRUS mode in five patients and the 360° TVUS mode in six patients, with 8–10 needles placed per patient. Comparing the ultrasound images to post-insertion CT images revealed mean needle positional differences of 3.8 and 2.4 mm, and mean angular differences of 3° and 2°, for TRUS and TVUS, respectively.
Rodgers noted that while the needle and patient anatomy were clearly visible in the ultrasound images, the needle tips could not be seen in deep insertions. This may be achieved, however, using the end-fire mode. In a proof-of-concept phantom study, the team combined end-fire and side-fire TVUS to visualize the placement of six needles. Here, the mean maximum difference from the CT images was 1.9 mm and the mean angular difference was 1.5°.
The next challenge is rapid needle localization, for which Rodgers is developing an automatic needle segmentation algorithm. In tests on a TVUS image, the algorithm took about 11 s to identify all needles with a mean positional difference of 0.8 mm from manual segmentation and an angular difference of 0.4°.
“3D ultrasound may provide an accessible and versatile approach for accurately visualizing needles and OARs intra-operatively, with automatic needle segmentation having the potential to improve the clinical utility,” Rodgers concluded.
Modelling lymphocyte dose helps optimize radiation treatments
The runner up Young Investigator was Abdelkhalek Hammi from Massachusetts General Hospital/Harvard Medical School. Hammi described a method for modelling the dose delivered to lymphocytes during intracranial radiation therapy.

Lymphocytes are white blood cells that form part of the body’s immune system. Irradiation of these calls is thought to lead to lymphopenia – an abnormally low level of lymphocytes in the blood. Hammi’s goal is to perform dynamic modelling of blood flow and radiation delivery to estimate the dose to circulating lymphocytes. Such work could increase our understanding of radiotherapy-induced lymphopenia and help optimize treatments to reduce lymphocyte depletion.
To achieve this, he used MRI to extract macroscopic brain vasculature data and develop a computational model of intracranial blood flow, extending this with a generic vessel model to create more than 1000 pathways through the brain. To simulate lymphocyte irradiation, the model explicitly tracks the motion of over 250,000 individual “blood particles” through the brain and radiation field and calculates the dose to each one.
Hammi simulated the rest of the human body using a statistical approach containing 24 organs. The entire blood flow model encompassed more than 22 million particles. He applied the models to compare dose to circulating blood from a 6-field intensity-modulated radiotherapy (IMRT) plan and a 3-field passive scattering proton therapy plan, both delivering 60 Gy in 30 fractions. He noted that the computation time was less than 10 s.
The mean delivered dose was 0.06 Gy for proton therapy and 0.13 Gy for IMRT, with maximum doses of 0.32 and 0.57 Gy, respectively. “We could see that the dose delivered to blood is really small compared with the dose to the target,” Hammi explained. “But the dose to blood in the proton plan is half of that received with IMRT.”
“This is the first explicit 4D blood flow model including recirculation to estimate radiation dose received by the circulating blood pool,” Hammi concluded, noting that his model accounts for blood flow throughout the entire body.
Deep learning helps spare the heart during radiotherapy
The third-place award this year went to Eric Morris, from Wayne State University and Henry Ford Cancer Institute. Morris presented his work on the use of deep learning to segment cardiac structures.

Radiation to the heart is potentially fatal for cancer patients, Morris told the audience. Sensitive cardiac substructures are linked to cardiac toxicity and avoiding these could improve patient outcome after radiotherapy. Unfortunately, there’s currently no accurate way to segment these structures as they are not visible in standard CT images. As such, they are not considered in radiation therapy planning.
MR images could provide more information, but MRI is not a standard part of treatment planning in all clinics. Instead, Morris developed a deep learning system that learns to delineate 12 cardiac substructures from cardiac MR images registered to treatment planning CTs, but requires only non-contrast CT inputs.
Morris trained the system using T2-weighted MRIs and CT images from 25 breast cancer patients. To prevent erroneous outputs, he implemented a post-processing step using conditional random fields (CRFs). He then assessed the system using 11 independent test patient CTs. In these test cases, “CRFs improved the segmentation results for all 12 substructures,” said Morris. “This gives us confidence that relevant features are maintained during post-processing.”
Prediction versus ground-truth evaluation revealed that the deep learning model accurately segmented all structures with a mean distance-to-agreement (MDA) of less than 2 mm. Nine structures had a Dice similarity coefficient (DSC) of over 0.75, including the heart chambers (0.87) and great vessels (0.85). Pulmonary veins were delineated with a DSC of 0.71 and coronary arteries with a DSC of 0.50.
Morris also compared results for the 11 test CTs with a previously developed multi-atlas method. The deep learning technique improved MDA by about 1.4 mm over the multi-atlas results, and increased DSC by 3–7% for the chambers and 23–35% for coronary arteries. “Deep learning provided a statistical improvement over multi-atlas for all substructures,” he emphasized, adding that his model takes about 14 seconds to perform segmentation, compared with around 10 minutes for the multi-atlas approach.
“Our deep learning model offers widespread applicability for efficient and accurate cardiac substructure segmentation on non-contrast enhanced treatment planning CTs,” Morris concluded. “This may yield stronger associations with outcome than standard-of-care dose evaluation using whole-heart metrics.”