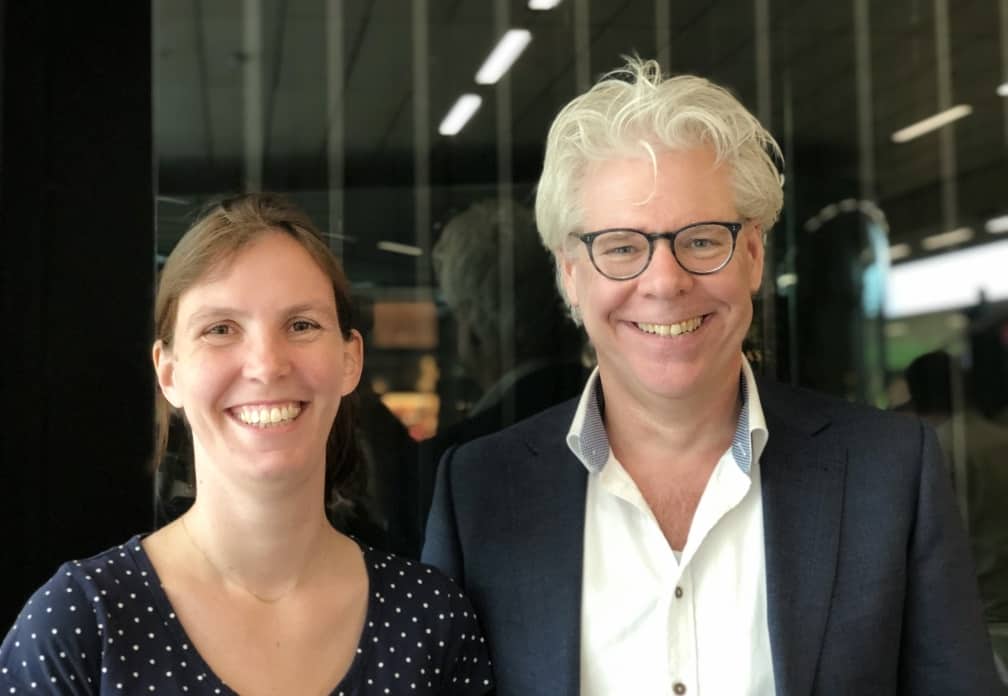
When patients suffer cardiac arrest, more than half of those who remain comatose never regain consciousness. Early and accurate prediction of neurological outcome is hence key when deciding whether to treat or not. Researchers from the Netherlands have trained an algorithm to read electroencephalograms (EEGs) and predict outcomes. They found that the algorithm’s results matched those of trained specialists (Crit. Care Med. 10.1097/CCM.0000000000003854).
Conventional methods to predict outcomes include applying an electrical signal to the wrist of a patient in a coma and checking for correlated brain activity. Failure to do so indicates very low odds of seeing the patient awakening one day. Another method consists of trained specialists analysing EEG traces – recordings of the brain electrical activity – as specific features can give hints of the outcome. For example, a flat EEG is associated with a poor outcome while a continuous EEG is often an indicator of a more favourable result.
The importance of the first 12 to 24 hours
Visual analysis of EEGs does not, however, capture their integral richness and is prone to intra- and inter-observer variability. EEGs also require highly trained analysts, who might not always be available to provide a prognosis within the paramount 12 to 24 hr window.
To remedy this, Marleen Tjepkema from the University of Twente decided to train deep convolutional neural networks (CNNs) to analyse the EEG and provide an outcome prediction. The CNN uses the ECG trace as an input that goes through a set of connected filters and mathematical functions that can extract specific features, in a similar way to neurons in the brain.
In the study, Tjepkema and her team examined 10 s EEG readings from 18 different channels, collected 12 and 24 hr after a cardiac arrest. Five nearby hospitals were involved: two centres provided the training, cross-validation and internal validation datasets, the other three supplied the data that would serve for external validation. In total, 661 patients were used to train the algorithm and 234 patients provided the validation dataset. All patients, apart from 31 lost to follow-up, were checked six months after the samples were recorded, to determine their cerebral performance category (CPC) score that defines their outcome as good or poor.
Matching trained specialists
In the training datasets, sensitivities for the prediction of poor outcomes were 42% and 57%, at 12 and 24 hr after cardiac arrest, respectively. Another important metric is the false positive rate (FPR), the prediction of one outcome when the opposite ends up happening. In both cases, the FPR for poor outcomes was 0%. Positive outcomes could be predicted with sensitivities of 48% and 33%, at 12 and 24 hr after cardiac arrest, respectively, both with an FPR of 5%.
In the validation dataset, 12 hr after cardiac arrest, the algorithm predicted poor outcomes with a 58% sensitivity and a 0% FPR, while it reliably predicted good outcomes with a 48% sensitivity and an FPR of 5%. Interestingly, predictions were less accurate 24 hr after cardiac arrest.

EEG can look deep into the brain
Using the complete dataset, six trained specialists performed visual assessment based on features associated with specific outcomes. Synchronous burst suppression on all channels was associated with a poor outcome, with a sensitivity of 37% at 12 hr and 25% at 24 hr (FPR of 0% in both cases). Conversely, a continuous EEG pattern correlated to positive outcome (sensitivity of 50% at a 9% FPR after 12 hr; sensitivity of 68% at a 20% FPR after 24 hr).
With these promising results, the researchers have shown that machine learning algorithms provide diagnostic information with similar, if not better, accuracy to human experts. Their focus is now shifting to understanding the patterns that the CNNs associate with specific outcomes, in the hope that this will provide new insights into the brain activity of comatose patients.