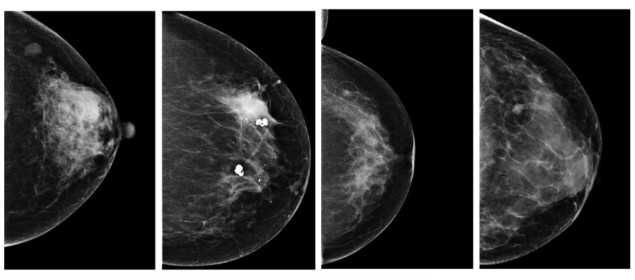
A comparison of three commercially available artificial intelligence (AI) systems for breast cancer detection has found that the best of them performs as well as a human radiologist. Researchers applied the algorithms to a database of mammograms captured during routine cancer screening of nearly 9000 women in Sweden. The results suggest that AI systems could relieve some of the burden that screening programmes impose on radiologists. They might also reduce the number of cancers that slip through such programmes undetected.
Population-wide screening campaigns can cut breast-cancer mortality drastically by catching tumours before they grow and spread. Many of these programmes employ a “double-reader” approach, in which each mammogram is assessed independently by two radiologists. This increases the procedure’s sensitivity – meaning that more breast abnormalities are caught – but it can strain clinical resources. AI-based systems might alleviate some of this strain – if their effectiveness can be proved.
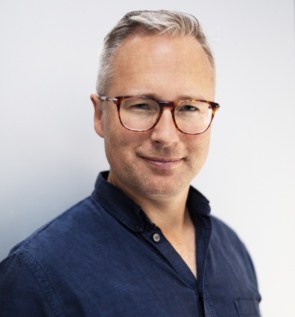
“The motivation behind our study was curiosity about how good AI algorithms had become in relation to screening mammography,” says Fredrik Strand at Karolinska Institutet in Stockholm. “I work in the breast radiology department, and have heard many companies market their systems but it was not possible to understand exactly how good they were.”
The companies behind the algorithms that the team tested chose to keep their identities hidden. Each system is a variation on an artificial neural network, differing in details such as their architecture, the image pre-processing they apply and how they were trained.
The researchers fed the algorithms with unprocessed mammographic images from the Swedish Cohort of Screen-Age Women dataset. The sample included 739 women who had been diagnosed with breast cancer less than 12 months after screening, and 8066 women who had received no diagnosis of breast cancer within 24 months. Also included in the dataset, but not accessible to the algorithms, were the binary “normal/abnormal” decisions made by the first and second human readers for each image.
The three AI algorithms rate each mammogram on a scale of 0 to 1, where 1 corresponds to maximum confidence that an abnormality is present. To translate this approach into the binary system used by radiologists, Strand and colleagues chose a threshold for each AI algorithm so that the binary decisions assumed a specificity (the proportion of negative cases classified correctly) of 96.6%, equivalent to the average specificity of the first readers. This meant that only mammograms that scored above the threshold value for each algorithm were classed as abnormal cases. The ground truth to which they were compared comprised all cancers detected at screening or within 12 months thereafter.
Under this system, the researchers found that the three algorithms, AI-1, AI-2 and AI-3, achieved sensitivities of 81.9%, 67.0% and 67.4%, respectively. In comparison, the first and second readers averaged 77.4% and 80.1%. Some of the abnormal cases identified by the algorithms were in patients whose images the human readers had classified as normal, but who then received a cancer diagnosis clinically (outside of the screening programme) less than a year after the examination.
This suggests that AI algorithms could help correct false negatives, particularly when used within schemes based on single-reader screening. Strand and colleagues showed that this was the case by measuring the performance of combinations of human and AI readers: pairing AI-1 with an average human first reader, for example, increased the number of cancers detected during screening by 8%. However, this came with a 77% rise in the overall number of abnormal assessments (including both true and false positives). The researchers say that the decision to use a single human reader or high-performing AI algorithm, or a human–AI hybrid system, would therefore need to be made after a careful cost–benefit analysis.
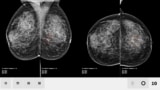
Artificial intelligence versus 101 radiologists
As the field advances, we can expect the performance of AI algorithms to improve. “I have no idea how effective they might become, but I do know that there are several avenues for improvement,” says Strand. “One option is to analyse all four images from an examination as one entity, which would allow better correlation between the two views of each breast. Another is to compare to prior images in order to better identify what has changed, as cancer is something that should grow over the years.”
Full details of the research are published in JAMA Oncology.