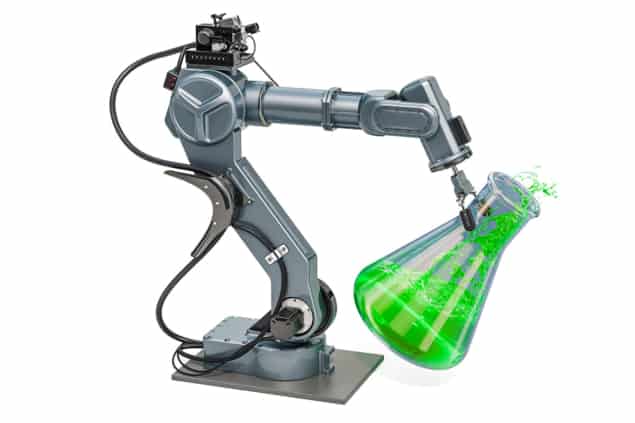
As our global energy demand increases, the need for sustainable routes to sources of energy and industrial chemicals becomes more urgent. Catalysis – the process by which a chemical increases the rate of a reaction – is the key to unlocking such routes. Research has therefore focused on development of new catalytic materials like photocatalysts, nanocatalysts, and electrocatalysts.
But what, exactly, makes a chemical compound a good catalyst?
Scott Denmark’s group at the University of Illinois hopes to answer that question using computer science. In their new paper in Science (DOI: 10.1126/science.aau5631), the group shows the power of information science in chemistry – a field called chemoinformatics – in predicting a compound’s catalytic activity.
“Experimental catalysis design allows researchers to qualitatively recognize patterns in catalyst structures to improve catalyst selectivity and efficiency,” says Andrew Zahrt, first-author of the paper and PhD student in chemistry. “This is an empirical method that does not always have or lead to mechanistic understanding of chemical reactions.”
Having this mechanistic understanding is key for catalysis, as a subtle change in a molecule’s structure – corresponding to an energy difference of about 1 kcal/mol between structures – can lead to large, measurable changes in catalyst performance. The ability to predict these changes can alleviate the burden of having to synthesize and test hundreds of molecules to optimize a given chemical system.
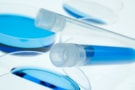
Robotics platform automates chemical synthesis
A new workflow for chemists
Their code first produces an in silico library that provides a collection of synthetically available catalysts for a particular system. Their code then calculates chemical descriptors for the catalysts and uses the results of those calculations to determine a subset of catalysts – called a “universal training set” – to examine experimentally. Zahrt describes the process:
“We calculate a conformer distribution and put it in a common grid comparable across all catalysts. The numbers themselves that we use are occupancy grid points in each point of space,” he said. “It’s like taking an image map, and taking an average occupancy to capture their flexibility – we want to answer the question: how much time does this group spend near an active site?”
The system eliminates the need for any prior mechanistic information about the system, as the system examines the structure of the catalysts to understand their properties. The group has been able to experimentally verify their findings and is now working on looking at larger material libraries. Currently, Zahrt says their progress is limited by the calculation of the initial conformer distribution; the code takes about one month to run for their library of 800 materials.
“We’re comparing very similar molecules. All we’re doing is changing subunits, but this can have dramatic changes,” Zahrt says. “This is definitely a model system, this is definitely a proof of concept stage.”
More details can be found in Science.