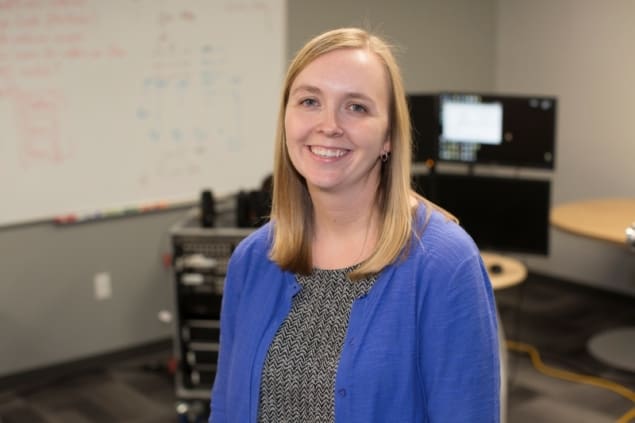
Intracortical brain-computer interfaces (BCIs), which use microelectrode arrays to record action potentials from neurons, could enable people with motor disabilities to communicate and interact with the world around them. BCIs rely upon a consistent relationship between neural firing rates and desired movement commands. Intracortical recordings, however, can be unstable, leading to challenges with maintaining BCI performance.
To address this challenge, a research team from the University of Pittsburgh has performed a detailed study to quantify the length of time that action potentials from the same neuron, or group of neurons, can be recorded from motor cortex (J. Neural. Eng. 15 046016).
“Many researchers in this field agree that intracortical recordings are unstable, but very few have quantified this,” explained Jennifer Collinger from the University’s Rehab Neural Engineering Labs. “We had the opportunity to quantify stability in human intracortical recordings, which anecdotally, some researchers have suggested might be more unstable than recordings from non-human primates.”
Data classification
Collinger and colleagues examined two subjects with tetraplegia who had implanted intracortical microelectrode arrays. They recorded neural data from Subject 1 during 287 BCI sessions over 33 months, and from Subject 2 in 51 sessions over six months.
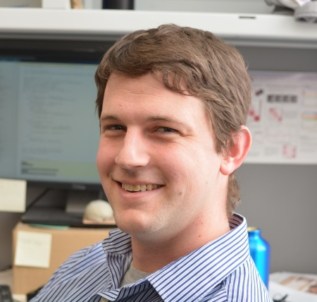
For all sessions, when the measured signal crossed a pre-defined threshold, a 1.6 ms snippet of the signal was saved. The researchers then sorted the snippets into “units” – collections of events with similar waveform shapes recorded from a single electrode. These units could be single-neuron action potentials, multi-neuron waveforms, or a collection of indistinct low-amplitude threshold crossings.
The team classified each unit according to its waveform shape, mean firing rate, autocorrelation and cross-correlation with other units’ firing; they then determined changes in these parameters between test sessions. They quantified unit stability over time using two methods: iterative-comparison, where each session is compared to the next; and direct-comparison, which compares each session to all following sessions.
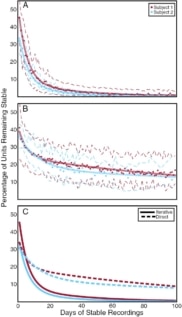
All sessions demonstrated a sharp initial decline in the number of stable units, plus a small group of long-lasting stable units. Fitting the data with a two-term exponential enabled the researchers to categorize each unit as unstable, moderately stable or highly stable. They note that this is a coarse classification and that future continuous wireless recordings could provide more detailed temporal profiles.
Using the more conservative iterative-comparison method, 47.1% of units recorded from Subject 1 were unstable, 44.7% showed moderate stability (with 16.3% of these becoming unstable each day) and 8.2% showed high stability (2.6% becoming unstable each day). Subject 2 had 61.4% unstable units, 32% with moderate stability (15.3% becoming unstable each day) and 6.6% with high stability (3.7% becoming unstable each day).
The direct-comparison approach characterized a larger percentage of units as highly stable: 19.7% and 12.5% for Subjects 1 and 2, respectively. For both subjects, nearly 20% of units were classified as moderately stable.
Single day stability
The team also examined stability within a single day, collecting data every hour for seven hours. For both subjects, 72-80% of units were stable across two consecutive recordings. The iterative-comparison approach estimated that stable units were lost at a rate of 8.7% and 12.5% per hour, for Subjects 1 and 2, respectively, while direct-comparison estimated decreases of 3.6% and 5.8% per hour.
To provide a rough estimate of overall unit stability, the researchers combined data from the between- and within-day experiments. Using iterative-comparison, approximately 25% of units were stable on the order of hours, 47.5% on the order of days and 7.5% on the order of weeks. With direct-comparison, approximately 45% of units were stable for hours, 17.5% for days, and 12.5% for weeks.
Predictive characteristics
The researchers also investigated whether any particular unit characteristics could distinguish stable from unstable units. They observed that unstable units generally had lower noise levels, lower peak-to-peak voltages, lower firing rates, less firing rate consistency and lower model-free tuning than units that were stable for at least one day.

Using a regression model to predict unit stability revealed that higher firing rates were most predictive of prolonged stability, with higher peak-to-peak voltage and model-free tuning values also correlated with longer stability. These characteristics could be used to identify units that would provide more stable long-term recordings, with the aim of creating more reliable intracortical BCIs.
One important criterion for a clinical BCI is to minimize device recalibration, for example by identifying stable units in real time and only using these for BCI control. “We have not tested this explicitly, but we have a good idea of which unit properties are common for highly stable units, so this would provide a starting point for creating a stable BCI decoder,” Collinger explained.
The researchers are now developing approaches to minimize recalibrations. Their analysis predicts that a 96-channel array would provide at least 15 stable units for between nine and 29 days. “We are also interested in how task-context might impact the functional stability of intracortical recordings,” said Collinger. “For example, we might record from the same neuron on two different days, but if it responds differently when the task conditions change, our BCI system will view it as unstable.”