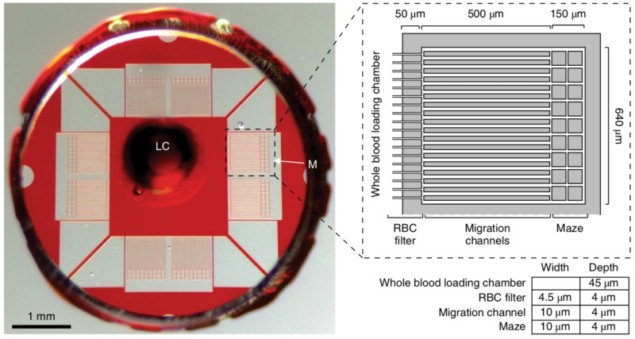
Requiring just a drop of blood, American researchers have designed a microfluidic assay that can diagnose sepsis, a life-threatening condition responsible for 250,000 deaths annually in the US. By using a new microfluidic device to measure the motility of neutrophils, the most common type of white blood cells in humans, and applying a machine learning algorithm, they were able to diagnose sepsis with 97% sensitivity and 98% specificity (Nature Biomed. Eng. 2 207).
Sepsis is an autoimmune reaction to infections that can eventually lead to inflammation and even organ failure. It affects one million patients each year in the US and about 30% of them are misdiagnosed due to poor sensitivity and specificity – the respective proportions of true-positive and true-negative test outcomes – of available tests.
Several biomarkers have been identified as possible targets for screening tests, but none has demonstrated the speed and accuracy required in clinical practice. Daniel Irimia and his team at Massachusetts General Hospital, Harvard Medical School and Shriners Hospital for Children have recently shown that neutrophils display a specific motility pattern in patients affected by sepsis, and that tracking their behaviour could lead to better diagnosis of the condition.
Training a microfluidic device
Building on these findings, the researchers designed a microfluidic assay that allowed the analysis of neutrophils’ behaviour and the diagnosis of sepsis based on a machine learning algorithm. A drop of blood is first collected and filtered by the assay to extract only the white blood cells. About 96% of cells entering the assay are hence neutrophils, which then migrate through multiple channels to one of the eight mazes located at the periphery of the device. Throughout the process, the neutrophil motility velocity and their patterns are tracked and characterized following a scoring system derived by the machine learning algorithm.
The training set for this algorithm was composed of 72 blood samples collected in 23 patients, with and without sepsis, at different times during their stay in the intensive care unit of Massachusetts General Hospital. An initial set of 13 variables was monitored and the algorithm reduced it to five, all characterizing the motility behaviour of the neutrophils. A scoring system with a threshold sepsis score was then derived to discriminate patients with and without sepsis.
High specificity and sensitivity
The researchers then tested the device prospectively in a double-blind study including 19 patients, 10 of whom had sepsis. Following the same approach as with the training set, blood samples were collected at various stages of a patient’s stay in the intensive care unit. The device correctly characterized all but one of the 79 blood samples, yielding a 96.3% sensitivity and 100% specificity for the validation set.
Such accuracy highlights the fundamental role that neutrophils play during sepsis and raises hopes that soon a ready-to-use tool will allow clinicians to quickly diagnose sepsis, putting an end to the unnecessary prescription of antibiotics that makes viruses more resistant to treatments. But to be translated to clinical practice, the duration of the test will have to be significantly reduced from the 6.5 hours currently required to provide a result. This is all the more important as it is estimated that the risk of death from sepsis increases by 8% for every hour that diagnoses is delayed.