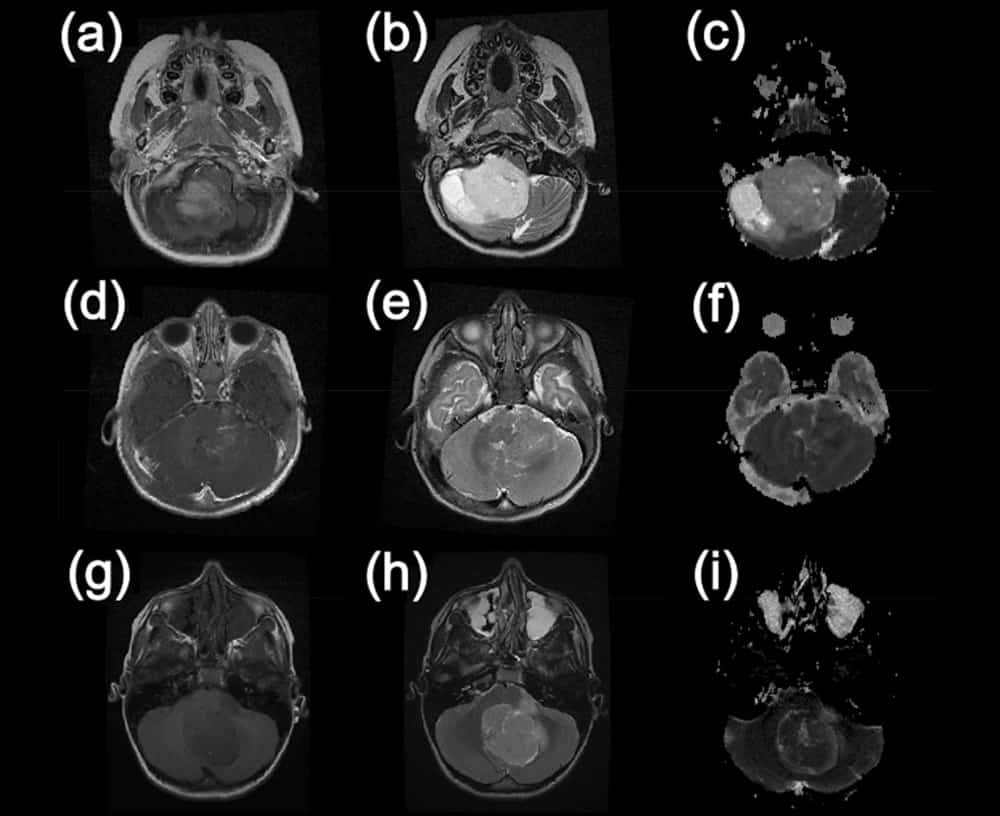
Brain and spinal cord tumours are the second most common cancers in children, making up about 26% of all childhood cancers. Many of these tumours are found in a region of the brain called posterior fossa, with the most common site being the cerebellum. There are three main types of such brain tumours – ependymoma, medulloblastoma and pilocytic astrocytoma – and as the treatment and outlook for each is different, accurate identification of tumour type is important to help improve surgical planning.
The most widespread method used in current clinical practice to characterize these tumours is through acquiring brain MRIs, which are then assessed by radiologists. However, this qualitative analysis is often challenging, due to the overlapping characteristics of these three types of cancers. This makes diagnosis difficult without the added confirmation of biopsy. One possible solution is to use diffusion-weighted imaging, which measures the random motion of water molecules in tissues, revealing details of the tissue microarchitecture. This more advanced MR technique can provide quantitative information regarding the tumour, in the form of apparent diffusion coefficient (ADC) maps, with the aim of improving diagnosis.
AI-based paediatric tumour classification…
A UK-based multi-centre study, led by the University of Birmingham and including researchers from the University of Warwick, focused on analysing ADC maps of paediatric brain tumours of the posterior fossa. The aim was to accurately identify the tumour type, without the need for biopsy. To achieve this, the group employed machine learning techniques and showed that it is possible to discriminate between the three most common types of paediatric posterior fossa brain tumour. They report their results in Scientific Reports.

Andrew Peet from the University of Birmingham explains: “When a child comes to hospital with symptoms that could mean they have a brain tumour, that initial scan is such a difficult time for the family and understandably they want answers as soon as possible. Here, we have combined readily available scans with artificial intelligence to provide high levels of diagnostic accuracy that can start to give some answers.”
…in a large-scale multi-centre study
The study involved 117 patients from five primary treatment centres across the UK (Nottingham, Newcastle, Great Ormond Street Children’s Hospital London, Alder Hey Liverpool and Birmingham Children’s Hospital), with MR images provided by 12 different hospitals (including the local hospitals where the children had their first scans) and a total of 18 different scanners. The images were analysed by a paediatric neuroimaging expert who manually drew regions-of-interest (ROIs) around the tumours. The researchers then extracted ADC values from the tumour ROIs and extracted a range of metrics from the ADC histogram.
The group used these features as input to two machine learning classifiers, a linear model called naïve Bayes (NB) and a non-linear model called random forest (RF), and trained them to noninvasively discriminate between the three most common types of paediatric posterior fossa brain tumours. The RF method achieved the best overall classification accuracy of 86.3%, while the NB classifier had the highest classification rates for ependymomas of 80.8%.
The authors report that these accuracies are not as high as previously seen in other studies. However, they note that their study is “much larger than the aforementioned studies with a more heterogeneous data input with regards to hospitals, scanners and acquisition protocols”. In fact, “previous studies using these techniques have largely been limited to single expert centres,” adds Peet. “Showing that they can work across such a large number of hospitals opens the door to many children benefitting from rapid noninvasive diagnosis of their brain tumour. These are very exciting times and we are working hard now to start making these artificial intelligence techniques widely available.”
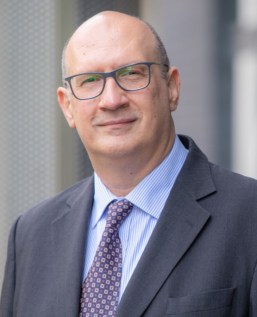
“Using AI and advance magnetic resonance imaging characteristics such as ADC values from diffusion-weighted images can potentially help distinguish, in a noninvasive way, between the main three different types of paediatric tumours in the posterior fossa,” explains co-author Theo Arvanitis from the University of Warwick.
“If this advanced imaging technique, combined with AI technology, can be routinely enrolled into hospitals, it means that childhood brain tumours can be characterized and classified more efficiently, and in turn means that treatments can be pursued in a quicker manner with favourable outcomes for children suffering from the disease,” Arvanitis adds.