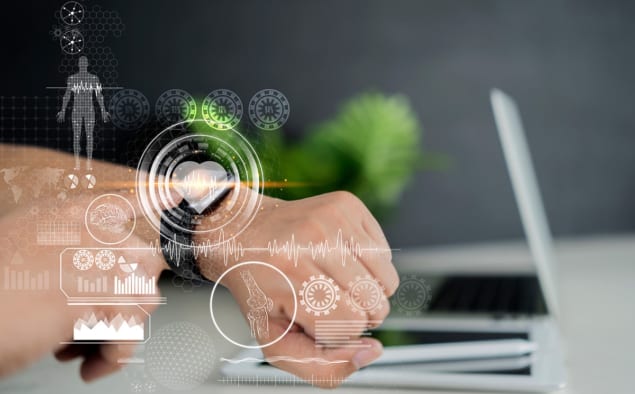
A new type of transistor could transform personal health monitoring by enabling artificial intelligence (AI) to analyse data inside wearable devices – without relying on cloud computing for help.
The “mixed-kernel heterojunction” (MKH) transistors were developed by researchers at Northwestern University, along with colleagues from the University of Southern California (USC). Unlike conventional transistors, they are not silicon based. Instead, their p-type material is semiconducting carbon nanotubes, while the n-type material comprises a monolayer of chemical vapour deposition-grown molybdenum disulphide.
The researchers used their MKH transistors to create a nanoelectronic AI system that can implement personalized machine learning (ML) algorithms, known as kernels. They showed that the device can classify large datasets – for example, identifying different types of arrhythmia (irregular heartbeats) in electrocardiograms (ECGs) – with high accuracy.
Categorizing data in this way using conventional electronics would require at least 100 silicon-based transistors. By contrast, just two of Northwestern’s MKH transistors are needed. Not only does this dramatically reduce the hardware footprint, it also requires far less power, enabling the system to be incorporated into consumer electronics such as smart watches.
“Our devices are 100-fold more energy efficient than conventional silicon electronics for ML classification such as detecting arrhythmia in electrocardiogram data. Because our devices are so efficient, they can be implemented directly with the sensors in wearable electronics, thus allowing ML classification to occur directly on wearable devices,” says Mark Hersam, from Northwestern’s McCormick School of Engineering, who co-led the project along with Han Wang from USC and Vinod Sangwan at Northwestern.
Fitting this MKH transistor-based electronics into smart watches and fitness trackers would also eliminate the need for remote processing of such data in power-hungry cloud data centres.
“AI and ML algorithms are energy-inefficient when implemented using conventional silicon electronics,” Hersam tells Physics World. “This energy inefficiency gets worse as the amount of data increases, which implies that AI/ML is on an unsustainable path towards consuming a significant fraction of the grid’s energy. We are hoping to solve this problem by developing new electronic devices that can more efficiently implement AI/ML.”
In a study reported in Nature Electronics, the researchers showed that their device could identify with 95% accuracy six different types of heartbeat – normal rhythm and five arrhythmias including atrial premature beat and premature ventricular contraction – from a dataset of 10,000 ECG tests.
Such precise results were achieved because the new transistors can “faithfully mimic mixed-kernel support vector machines for enhanced ML classification accuracy,” Hersam explains. Mixed-kernel support vector machines are a type of AI that can classify complicated, multi-dimensional datasets such as biological data with many different features. This makes it ideal for analysing ECGs and other personal health data.
In addition, the ability to reconfigure these MKH transistors by strongly modulating the current flow through them enables the AI accuracy to be optimized for each specific patient. This opens the way for real-time personalized results that could improve health outcomes by enabling earlier medical intervention when problems arise. Users would also benefit from enhanced security for their sensitive health information because there is no need to pass their data to and from the cloud for analysis.
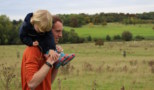
Heartbeat detection in smartwatch signals wins Physiological Measurement best paper award
The team now aims to develop mass production methods and expand the range of potential uses for their transistors.
“Our MKH transistors have been demonstrated at the lab scale. Large-scale deployment in wearable devices will require the development of scalable manufacturing methods, which could occur in a few years with suitable investment. In the future, we will be working on scalable manufacturing of our MKH transistors. In addition, we will be generalizing our MKH transistors for other AI/ML applications,” says Hersam.