Matt Hodgson reviews Why Machines Learn: The Elegant Maths Behind Modern AI by Anil Ananthaswamy
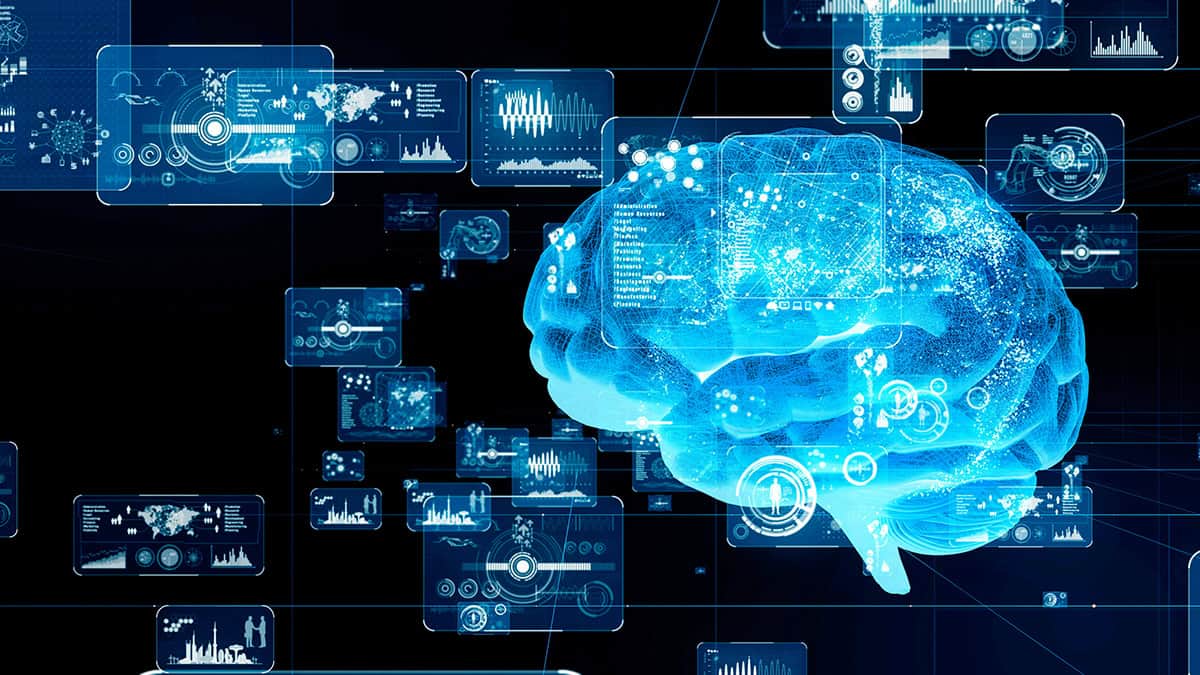
Artificial intelligence (AI) is rapidly becoming an integral part of our society. In fact, as I write this article, I have access to several large language models, each capable of proofreading and editing my text. While the use of AI can be controversial – who’s to say I really wrote this article? – it may soon be so commonplace that mentioning it will be as redundant as noting the use of a word processor. Just to be clear though, this review is all my own work.
It’s still early days for AI, but it has the potential to impact every aspect of our lives, from politics to education and from healthcare to business. As AI is used more widely, it’s not just computer scientists who need to understand how machines think and come to conclusions. Society as a whole must have a basic appreciation and understanding of how AI works to make informed decisions.
Why Machines Learn, by the award-winning science writer Anil Ananthaswamy, takes the reader on an entertaining journey into the mind of a machine. Ananthaswamy draws inspiration from the subject of his book; much like training a neural network, he uses well-designed examples to build the reader’s understanding step by step.
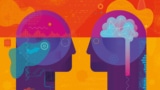
The Turing Test 2.0
Whereas AI is a general term that covers human-like qualities such as reasoning and adaptive intelligence, the author’s focus is on the subfield of AI known as “machine learning”, which is all about how we extract knowledge from data. Starting with the fundamentals, Ananthaswamy carefully constructs a comprehensive picture of how machines learn complex relationships.
Anyone who thinks that AI is a modern invention, or that the road to today’s technology has been smooth, will be shocked to learn that the pursuit of a “learning machine” began in the 1940s with McCulloch and Pitts’ model of a biological neuron. Funding for this now billion-dollar industry has sometimes also been meagre. What’s more, the concepts underpinning modern AI have their roots in diverse and unexpected areas, from the idle curiosities of academics to cholera outbreaks and even game shows.
Ananthaswamy’s background in electronics and computer engineering is evident throughout this book, for example in how he introduces several technical and mathematical concepts needed to grasp the power and limitations of machine learning. He begins with the US psychologist Frank Rosenblatt’s development in the late 1950s of the “perceptron” – a basic, single-layer learning algorithm with a binary output (“yes” or “no”). The author then shows how decades of innovation have led to deep neural networks with billions of neurons, such as ChatGPT, capable of giving nuanced and insightful responses.
Unusually for a popular-science book, Why Machines Learn includes quite a lot of mathematics and equations
Unusually for a popular-science book, Why Machines Learn includes quite a lot of mathematics and equations as it explores how vectors, linear algebra, calculus, optimization theory, statistics and probability can be employed to engineer a synthetic brain. Given the complexity of these topics, Ananthaswamy is careful to provide frequent recaps of key concepts so that readers less familiar with these ideas don’t become lost or overwhelmed.
Fortunately, the author has the uncanny ability to answer questions with simple and illuminating examples just as they arise in the reader’s mind. Although this might seem to diminish some of the magic, it’s inspiring to see how such a powerful system can be constructed from deceptively simple components.
Gaming the system
For topics such as probability, this pedagogical approach is essential for anyone without a strong background in mathematics. For instance, the infamous Monty Hall problem is so counterintuitive that even some of the world’s most renowned mathematicians struggled to accept its solution. Indeed, as Ananthaswamy notes, Paul Erdős – one of the most prolific mathematicians of the 20th century – “reacted as if he’d been stung by a bee” when confronted with the answer.
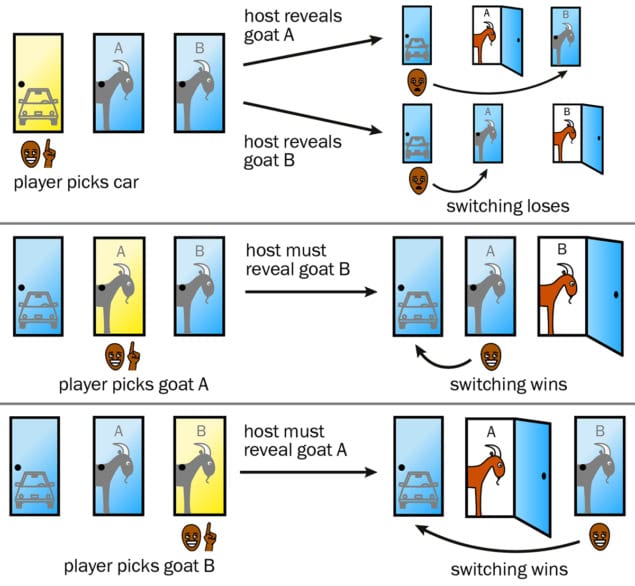
The dilemma is based on the classic US TV game show Let’s Make a Deal, hosted by Hall, in which you, the contestant, have a choice of three doors. Behind one is a brand-new sports car, while the other two doors conceal goats. After you pick a door (but don’t get to see what’s behind it) the host opens one of the other two doors to reveal a goat. The host then offers you the chance to switch your choice to the remaining, unopened door.
To maximize your chances of winning the car, you should always switch. It’s counter-intuitive and controversial. Surely, if you’ve got two doors to pick from, then the odds of getting the car must be 50–50? In other words, why would you bother switching if there’s a car behind one door and a goat behind the other? The book goes on to explain how the logic behind this decision is a cornerstone of machine learning; bypassing human intuition, which is often flawed.
Ananthaswamy shows that machine learning is a powerful method of analysis. He first demonstrates how data can be represented in an abstract, high-dimensional space, before explaining how collapsing the number of dimensions allows patterns in the data to be found. With the assistance of some elegant linear algebra, this process can be engineered so that the data is categorized most effectively; highlighting strong correlations, which leads to more reliable performance.
Cautious embrace
In our data-driven world, this remarkable capability of AI is something that should be embraced. However, like any data-analysis method, machine learning is prone to biases and errors. Why Machines Learn gives the reader an awareness and understanding of these shortcomings, allowing AI to be used more effectively.
This is particularly important as AI becomes mainstream. It’s common for people to mistakenly believe that it’s some all-powerful super brain, capable of completing any task with ease. This misconception can lead to the misuse of AI or the unfair perception of AI as overrated when it falls short of this unrealistic standard. Ananthaswamy gives his readers an appreciation of how machine learning works and, hence, how to use it appropriately, which may help combat the abuse of AI.
It’s evident that machines are far from achieving human-like intelligence
By exploring the fundamental principles of machine learning in such detail, it’s evident that machines are far from achieving human-like intelligence. While the secrets of the human brain remain elusive, Why Machines Learn demystifies the underlying mechanisms behind machine learning, which may possibly lead to a better understanding of the learning process itself and the development of improved AI.
This inevitably requires the reader to confront advanced and counterintuitive concepts in various branches of mathematics and logic, from collapsing dimensions to mind-bending games of chance. For those who invest the time and effort, they will reap the rewards that come from understanding a technology with the potential to revolutionize many aspects of our lives.
- 2024 Penguin/Allen Lane 480pp £30hb/£16.99 ebook