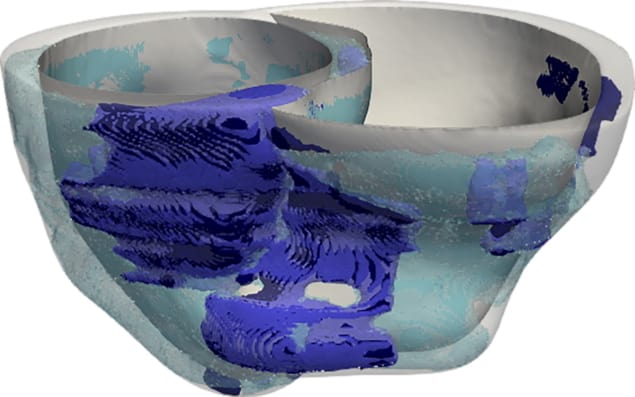
Julie Shade, a biomedical engineering PhD candidate in Natalia Trayanova’s lab at Johns Hopkins University, hopes her research will change how doctors manage cardiac sarcoidosis.
Cardiac sarcoidosis is an inflammatory heart disease in which clusters of white blood cells gather in heart tissue and interfere with the heart’s electrical system. Some patients with cardiac sarcoidosis will have irregular heartbeats called arrythmias that may progress to heart failure or sudden cardiac arrest.
Unfortunately, doctors don’t have precise methods to identify which patients with cardiac sarcoidosis will have an arrythmia. Consequently, only one-third of these individuals receive appropriate care, such as implantation of a defibrillator, and up to a quarter of patients receive inappropriate therapy. In this latest study, results of which are reported in Science Advances, Shade combines data from two types of heart scan with machine learning to create a tool that predicts a patient’s risk of sudden cardiac arrest.
“This is the first study to assess the propensity to electrical disturbances in patients’ hearts using information from multiple imaging modalities,” explains Shade. “When we combined this information with other clinical data in a multivariable classifier, we arrived at an approach that could be used as a clinical decision support tool, helping a clinician assess a patient’s risk of sudden cardiac death due to arrhythmia.”
Modelling electrical signals in cardiac sarcoidosis
Regions of fibrosis and inflammation in the heart – hallmarks of cardiac sarcoidosis – slow down and disturb the electrical signals passing through the heart. To gain insight into how fibrosis and inflammation affect heartbeat, Shade created three-dimensional models of cardiac sarcoidosis patients’ hearts and then measured how electrical conduction in each model becomes disorganized as the result of disease changes in the heart. Researchers could extrapolate these findings to clinical outcomes and a patient’s risk of having an arrythmia.

While Trayanova’s lab had experience modelling cardiac electrical function, modelling electrical signals’ pathways in cardiac sarcoidosis posed unique challenges.
For example, fibrosis is best visualized using magnetic resonance imaging (MRI), while areas of inflammation are seen with positron emission tomography (PET). Shade and the rest of the research team identified areas of fibrosis and inflammation in each heart by identifying thresholds of image intensity in the MR and PET images and checked these regions against radiologists’ reports. Regions of fibrosis and inflammation identified, the researchers registered MR and PET images/volumes using a combination of landmark- and mutual information-based techniques.
Next, regions of fibrosis and inflammation in each personalized model were populated with heart cells with abnormal properties. Then the researchers took an in-depth, point-by-point look at how the fibrosis and inflammation affected heartbeats by poking each modelled heart with a myriad of tiny electrical signals. They validated the results of their models with clinical ablation data.
“The number of patient heart models here – forty-five – developed for the evaluation of arrhythmia likelihood is the largest in any patient-specific heart modelling study to date, and entailed an enormous effort and investment,” says Trayanova, who is senior author on the study. “We worked together with the clinical team, which had added tremendous value to this study.”
Predicting arrythmias using a classifier
Personalized heart models of patients with sarcoidosis can predict, fairly accurately, which heart is likely to develop heartbeat dysfunction. However, they do not account for other patient clinical data, which, in a complex disease like sarcoidosis, might provide additional predictive value. Therefore, once the heart models were built and validated, Shade implemented a supervised classifier to identify which patients are at risk of sudden cardiac death due to arrhythmia. She trained, tested and validated the classifier on the personalized modelling results, as well as additional MRI and PET data and clinical information acquired retrospectively from 45 patients at Johns Hopkins Hospital.
Shade’s classifier (60% sensitivity, 72% specificity, area under the curve 0.754) outperformed traditional clinical metrics (no higher than 38% sensitivity and specificity). Shade notes that while the sample size of their study is small from a machine learning perspective, it’s large from a modelling perspective.
“Our validation results were stronger than the test results. But the test results were not bad,” says Shade. “I think the reason for that [good performance despite small sample size] is that most or many of the features in the machine learning classifier were based on a mechanistic understanding of how a patient’s heart works…We’re not giving [the classifier] features that have no biophysical meaning.”
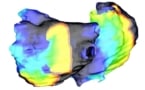
Simulations guide targeted ablation of atrial fibrillation
“The synergistic combination of mechanistic modelling and machine learning that [we developed in] this study is unique,” Trayanova adds. “The inclusion of features from results of mechanistic modelling in a machine learning classifier imparts interpretability to the predictions, exemplifying how concerns over clinical decisions being informed by ‘black box’ algorithms could be overcome.”
Johns Hopkins Medicine is now prospectively collecting MR and PET images from patients with cardiac sarcoidosis, and Shade would like to use these images to see if the researchers’ combined model and classifier can correctly predict occurrence of arrythmia in these patients. She would also like to validate their modelling techniques and classifier performance in a multi-centre study.