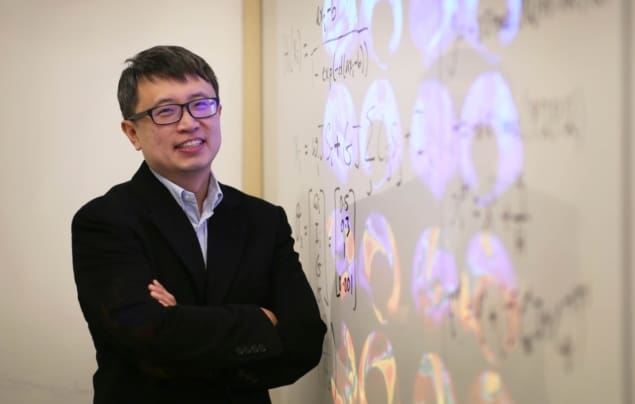
A research collaboration headed up at the National University of Singapore (NUS) has successfully employed machine learning to investigate the cellular architecture of the human brain. The approach uses functional MRI (fMRI) data to automatically estimate brain parameters, enabling neuroscientists to infer the cellular properties of different brain regions without having to surgically probe the brain. The researchers say that their technique could potentially be used to assess treatment of neurological disorders or develop new therapies (Science Advances 10.1126/sciadv.aat7854).
“The underlying pathways of many diseases occur at the cellular level, and many pharmaceuticals operate at the microscale level,” explains team leader Thomas Yeo. “To know what really happens at the innermost levels of the human brain, it is crucial for us to develop methods that can delve into the depths of the brain non-invasively.”
Currently, most human brain studies employ non-invasive approaches such as MRI, which limits examination of the brain at a cellular level. To bridge this gap between non-invasive imaging and cellular insight, researchers around the world have used biophysical brain models to simulate brain activity. However, many of these models rely on overly simplistic assumptions, such as assuming that all brain regions have the same cellular properties, which is known to be incorrect.
Yeo and his team, working with researchers from Universitat Pompeu Fabra, Universitat Barcelona and UMC Utrecht, analysed imaging data from 452 participants of the Human Connectome Project. In contrast to previous modelling work, they allowed each brain region to have distinct cellular properties and exploited machine learning algorithms to automatically estimate the model parameters.
“Our approach achieves a much better fit with real data,” says first author Peng Wang. “Furthermore, we discovered that the micro-scale model parameters estimated by the machine learning algorithm reflect how the brain processes information.”
The researchers found that brain regions involved in sensory perception, such as vision, hearing and touch, exhibited cellular properties opposite to those from brain regions involved in internal thought and memories. The spatial pattern of the human brain’s cellular architecture closely reflects how the brain hierarchically processes information from the surroundings. This form of hierarchical processing is a key feature of both the human brain and recent advances in artificial intelligence.
“Our study suggests that the processing hierarchy of the brain is supported by micro-scale differentiation among its regions, which may provide further clues for breakthroughs in artificial intelligence,” says Yeo.
Moving forward, the NUS researchers plan to apply their approach to examine the brain data of individual participants, to better understand how individual variation in the brain’s cellular architecture may relate to differences in cognitive abilities. They hope that these latest results will provide a step towards the development of individualized treatment plans with specific drugs or brain stimulation strategies.