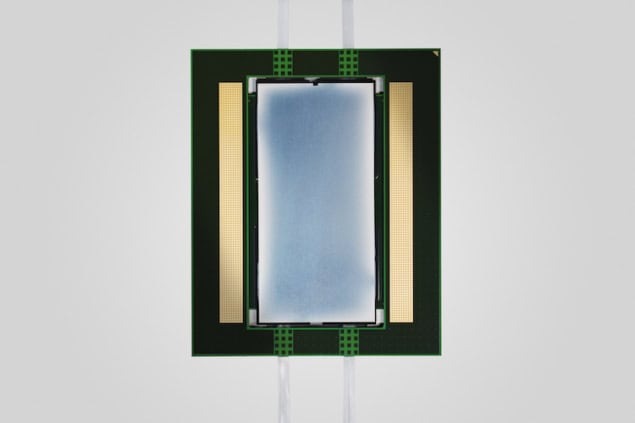
Researchers in Singapore and the US have independently developed two new types of photonic computer chips that match existing purely electronic chips in terms of their raw performance. The chips, which can be integrated with conventional silicon electronics, could find use in energy-hungry technologies such as artificial intelligence (AI).
For nearly 60 years, the development of electronic computers proceeded according to two rules of thumb: Moore’s law (which states that the number of transistors in an integrated circuit doubles every two years) and Dennard scaling (which says that as the size of transistors decreases, their power density will stay constant). However, both rules have begun to fail, even as AI systems such as large language models, reinforcement learning and convolutional neural networks are becoming more complex. Consequently, electronic computers are struggling to keep up.
Light-based computation, which exploits photons instead of electrons, is a promising alternative because it can perform multiplication and accumulation (MAC) much more quickly and efficiently than electronic devices. These operations are crucial for AI, and especially for neural networks. However, while photonic systems such as photonic accelerators and processors have made considerable progress in performing linear algebra operations such as matrix multiplication, integrating them into conventional electronics hardware has proved difficult.
A hybrid photonic-electronic system
The Singapore device was made by researchers at the photonic computing firm Lightelligence and is called PACE, for Photonic Arithmetic Computing Engine. It is a hybrid photonic-electronic system made up of more than 16 000 photonic components integrated on a single silicon chip and performs matrix MAC on 64-entry binary vectors.
“The input vector data elements start in electronic form and are encoded as binary intensities of light (dark or light) and fed into a 64 x 64 array of optical weight modulators that then perform multiply and summing operations to accumulate the results,” explains Maurice Steinman, Lightelligence’s senior vice president and general manager for product strategy. “The result vectors are then converted back to the electronic domain where each element is compared to its corresponding programmable 8-bit threshold, producing new binary vectors that subsequently re-circulate optically through the system.”
The process repeats until the resultant vectors reach “convergence” with settled values, Steinman tells Physics World. Each recurrent step requires only a few nanoseconds and the entire process completes quickly.
The Lightelligence device, which the team describe in Nature, can solve complex computational problems known as max-cut/optimization problems that are important for applications in areas such as logistics. Notably, its greatly reduced minimum latency – a key measure of computation speed – means it can solve a type of problem known as an Ising model in just five nanoseconds. This makes it 500 times faster than today’s best graphical-processing-unit-based systems at this task.
High level of integration achieved
Independently, researchers led by Nicholas Harris at Lightmatter in Mountain View, California, have fabricated the first photonic processor capable of executing state-of-the-art neural network tasks such as classification, segmentation and running reinforcement learning algorithms. Lightmatter’s design consists of six chips in a single package with high-speed interconnects between vertically aligned photonic tensor cores (PTCs) and control dies. The team’s processor integrates four 128 x 128 PTCs, with each PTC occupying an area of 14 x 24.96 mm. It contains all the photonic components and analogue mixed-signal circuits required to operate and members of the team say that the current architecture could be scaled to 512 x 512 computing units in a single die.
The result is a device that can perform 65.5 trillion adaptive block floating-point 35 (ABFP) 16-bit operations per second with just 78 W of electrical power and 1.6 W of optical power. Writing in Nature, the researchers claim that this represents the highest level of integration achieved in photonic processing.

Optical chipmaker focuses on high-performance computing
The team also showed that the Lightmatter processor can implement complex AI models such as the neural network ResNet (used for image processing) and the natural language processing model BERT (short for Bidirectional Encoder Representations from Transformers) – all with an accuracy rivalling that of standard electronic processors. It can also compute reinforcement learning algorithms such as DeepMind’s Atari. Harris and colleagues have already applied their device to several real-world AI applications, such as generating literary texts and classifying film reviews, and they say that their photonic processor marks an essential step in post-transistor computing.
Both teams fabricated their photonic and electronic chips using standard complementary metal-oxide-semiconductor (CMOS) processing techniques. This means that existing infrastructures could be exploited to scale up their manufacture. Another advantage: both systems were fully integrated in a standard chip interface – a first.
Given these results, Steinman says he expects to see innovations emerging from algorithm developers who seek to exploit the unique advantages of photonic computing, including low latency. “This could benefit the exploration of new computing models, system architectures and applications based on large-scale integrated photonics circuits.”