
The vast quantity of data generated by global monitoring initiatives and large-scale research facilities present both new opportunities and challenges for scientists. Results that can be captured in minutes may take years to fully understand.
To help researchers review and analyse this growing volume of information, cloud-based platforms are now being developed to combine distributed access with shared high-power computing resources. These tools are opening the door to massively collaborative projects, including citizen science, and are providing a manageable route for making publicly-funded research available to the wider world.
Catherine Jones, based at the STFC’s Rutherford Appleton Laboratory in Oxfordshire, UK, leads the software engineering group at the Ada Lovelace Centre – an integrated, cross-disciplinary, data intensive science centre supporting national facilities such as synchrotrons and high-power lasers.
In her role, Jones is closely involved with providing researchers with access to tools and data over the cloud – an approach known as Data Analysis as a Service. “Traditionally, researchers using our facilities would have taken the data with them, but as data volumes increase you need to look at other solutions,” she says.
Our experiences encourage us to think about simple and easy pathways and not to make our solutions overly complicated
Using internal cloud facilities at the STFC, Jones and her colleagues offer researchers access to virtual machines designed to simplify working with large amounts of scientific results. “The virtual machines are aimed at a specific scientific technique,” Jones explains. “Whenever a user spins one up, they have access to their data and to the routines that they’ll need for that analysis, together with the right amount of computing resource.”
The cloud-based tools require testing and documentation to make sure that the platform meets not just the researchers’ immediate needs, but also provides a robust solution long term. In other words, a product that can be serviced, supported and transferred.
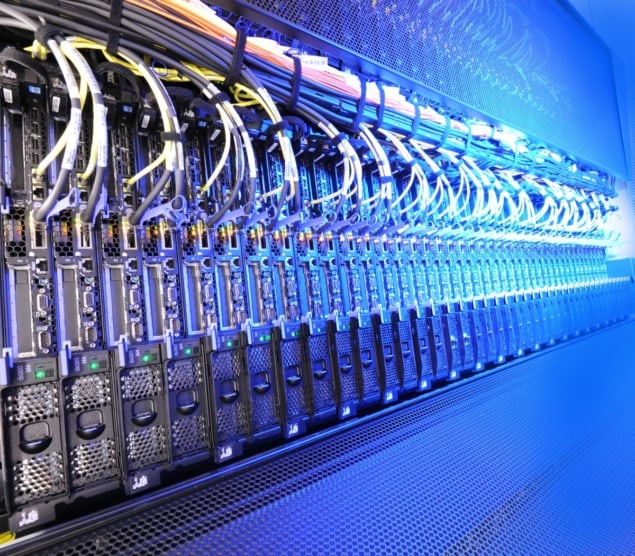
Currently, the system supports scientists conducting research using one specific experimental technique at the STFC’s ISIS neutron spallation facility, with plans to roll it out further. It’s a model that could be applied across different research communities, although each one will have its own specific needs. Detailed requirements gathering is essential to understand the machine learning and AI needs across multiple labs.
The benefits of a cloud-based approach to data analysis include streamlined administration and maintenance. For example, the use of virtual machines makes it easier to roll out software upgrades and apply version control so that scientific models can be re-run, and their results reproduced in the future.
There are advantages too when it comes to configuring the work environment. “It’s easier to match the computing resources to the analysis, as a cloud setup is more flexible,” says Jones. “It’s a more elastic resourcing mechanism.” The hope here is that researchers will gain more time to spend on the analysis, with less to worry about in terms of the hardware under the hood.
Different fields, different requirements
As Jones points out, different scientific fields can have different requirements when it comes to dealing with the demands of big data. John Watkins, who is head of environmental informatics at the Centre for Ecology & Hydrology (CEH), gives an example.
“With particle physics, the challenges are likely to be more in terms of data volume and the analytics of a particular data flow,” he says. “However, with environmental science you are often assessing a very broad variety of data. This needs to be pulled from multiple sources and can be very, very different in nature.”
Watkins’ colleague, Mike Brown – who is head of application development at CEH – refers to the so-called Vs of big data (a list that includes volume, variety, velocity, and veracity) to emphasize the multiple challenges associated with providing scientists with easy access to data and analytical tools.
It’s not just about providing easy-to-use interfaces, it’s also about enabling the dialogue between researchers with a shared aim
A key objective for Brown and Watkins is to connect environmental scientists who understand the data with experts in numerical techniques who are developing cutting-edge analytical methods. Once again, the solution has been to provide collaborative facilities in the cloud – this time through a project known as DataLabs, funded by NERC.
“It’s not just about providing easy-to-use interfaces, it’s also about enabling the dialogue between researchers with a shared aim,” Watkins comments. “The provision of collaborative tools such as Jupyter Notebooks or R-Shiny apps are a way of achieving this over time.”
To break down the DataLabs project into user stories, an approach that helped the team to capture the key features of the platform and quickly pilot its ideas, Watkins and Brown worked with experts at Tessella. “The aim in the first 12 months was to build a proof-of-concept to show that all the different elements could work together and would be useful for the community,” says Jamie Downing, a project manager at Tessella who has been supporting the programme’s core partners.
Today, the group has the essential elements in place from end-to-end, and the first case studies show that Data Labs has got off to a flying start. As an example, researchers are now using the cloud-based environment to run much more detailed CEH land-cover models. The leap in performance (a jump from 1 km to 25 m resolution), coupled with significantly reduced execution time, is a huge improvement on what was possible under the previous physical workstation-based approach.
Digital twin
Other fields get to benefit too. The experience in developing DataLabs has provided a springboard for rolling out similarly collaborative platforms such as solutions supporting the Data and Analytics Facility for National Infrastructure (DAFNI). This is a project that aims to integrate advanced research models with established national systems for modelling critical infrastructure.
“Led by Oxford University and funded by the EPSRC, the initiative aspires over the next 10 years to be able to model the UK at a household level, 50 years into the future,” explains Nick Cook, a senior analyst at Tessella. Here, the firm is involved in conceptualizing DAFNI’s capabilities and implementation roadmap.
One of the project’s early goals is to create a “digital twin” of a UK city such as Exeter – in other words, to virtually describe a city with a population of several hundred thousand people together with its transport infrastructure, utility services and environmental context. This digital twin would, for example, help planners to decide where to invest in new road or rail networks, and to identify the best sites for housing, schools and doctors’ surgeries.
Cook cautions that such a hyperscale systems approach will succeed only if it performs in a reliable, repeatable and provenanced way. “When users deliver their findings, they need to be able to justify how the results that have been generated in an analogous way to applying scientific best practices of high energy physics or life science research – engendering a sense of trust in their outcomes to perhaps skeptical or hostile audiences,” he emphasizes.
DAFNI is looking very closely at what DataLabs is doing as a way of providing the interface and the virtual research spaces within its own cloud. Both proposals share requirements to store the results in a traceable way that preserves the integrity of the data and protects it against tampering, inadvertent corruption, or malicious use. It’s an area that could one day see digital ledgers, or block chains, playing an important role – particularly when dealing with the sensitive nature of critical national infrastructures.
More food for thought
As well as supporting collaborative number crunching, cloud-based big science solutions make it much easier to reach out and share knowledge and expertise – for example, through webinars and workshops.
Today, more and more of us have experience of operating in the cloud, collaborating on projects at work, and watching movies and sharing photos at home. Popular online platforms have become easier to use and more personalized to our requirements. But as expectations rise, so can our demands in terms of what an interface can do and the features we’d like to see.
“It can be a challenge when you don’t have the resources of giants like Google, but it’s all to the good as our experiences encourage us to think about simple and easy pathways and not to make our solutions overly complicated,” says Jones.
Goodbye USB
Summing up, the days of doing an experiment and being able to carry the full data set back to your PC on a USB stick are over. And while it’s unlikely to surprise many that cloud storage and online data access has risen to the challenge, the devil is in the detail. Get it right and platforms can do so much more for the scientific community – providing scalable computing resource, simplifying maintenance and upgrades, and enabling multidisciplinary collaboration to spur on research progress.
Read more in “Artificial intelligence and cloud computing: the future for scientific research”, available for download from the Tessella website.