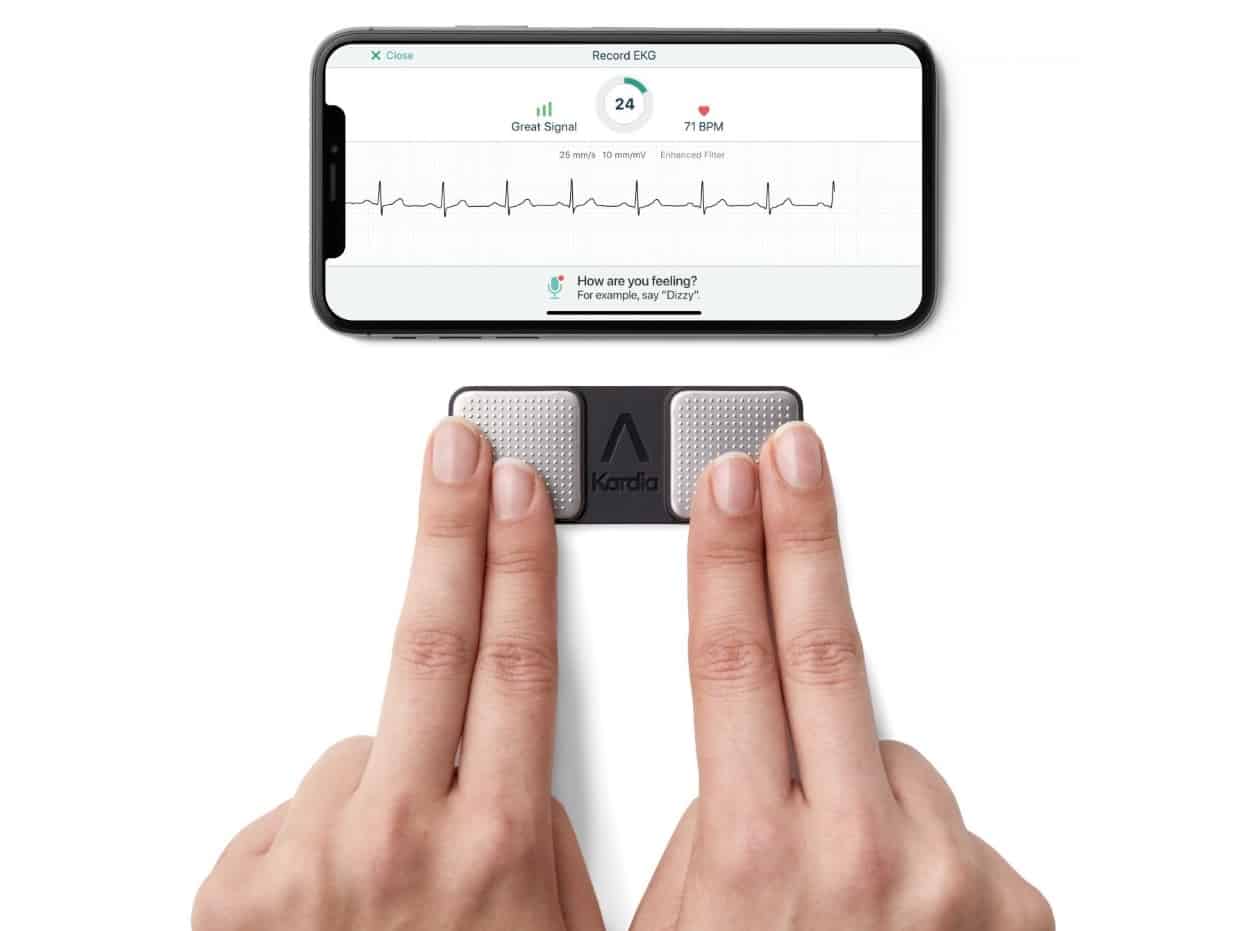
A new artificial intelligence algorithm could soon enable patients to automatically diagnose and monitor a common and potentially fatal heart disorder at home, using a small device that plugs into a smartphone (Heart Rhythm 10.1016/j.hrthm.2018.06.037).
Atrial fibrillation (AF) is a heart rhythm disorder that significantly increases a person’s risk of stroke. It is thought to affect around 1 million people in the UK, but often remains undiagnosed. To diagnose and monitor the condition, and inform treatment options, patients usually undergo regular 12-lead electrocardiograms (ECGs), performed using bulky equipment at hospitals or doctors’ surgeries, which are analysed by the patient’s physician.
ECG results, however, can be unreliable at diagnosing patients with AF, as attacks are often transient and may not be present at the time of the procedure. As such, subjective descriptions of symptoms are also important in making a diagnosis.
The Kardia Mobile Cardiac Monitor (KMCM) is a small, simple device that allows AF patients to regularly monitor their condition themselves at home. It contains two electrodes, which record a 30 second ECG trace from the patient’s fingers that can be analysed using a smartphone app. The device is already in use for patients to collect ECG data at home and transmit these for review by their doctor. Used in this way, the device has been successful in monitoring patients who are undergoing or have recently received treatment.
Researchers at the Cleveland Clinic and Marshall University have now investigated the effectiveness of the KMCM paired with the new algorithm. The study included 52 AF patients, who used the KMCM themselves to obtain an ECG trace. Immediately before this, each patient underwent a conventional 12-lead ECG. This was analysed by physicians to provide a baseline with which to evaluate the automated system. Because the condition is variable, only some of the patients were experiencing AF at the time of the ECG.
The KMCM was able to identify 71.2% of AF cases given to it (the sensitivity). It had very high specificity: 94.1% of those categorized as AF were correctly labelled. The lower sensitivity was because many traces could not be categorized, usually because the heart rate was too high or too low, or because of excessive noise. When these unclassified traces were excluded, sensitivity rose to 96.6%.
While the high proportion of unclassified readings means the system still requires oversight from experienced doctors, group leader Khaldoun Tarakji sees value in the device as a useful tool alongside current monitoring techniques.
“Patients can use an automated atrial fibrillation detection as a basis for pursuing additional medical follow-up, and clinicians may use it to develop treatment plans supported by more objective data rather than relying only on symptoms,” he comments. “As physicians, we should not view these products as threats, but rather as an opportunity to provide better and more effective care for our patients.”