Available to watch now, IOP Publishing, in sponsorship with Sun Nuclear Corporation, explores the current state-of-the-art deep-learning development in CT image generation
Want to learn more on this subject?
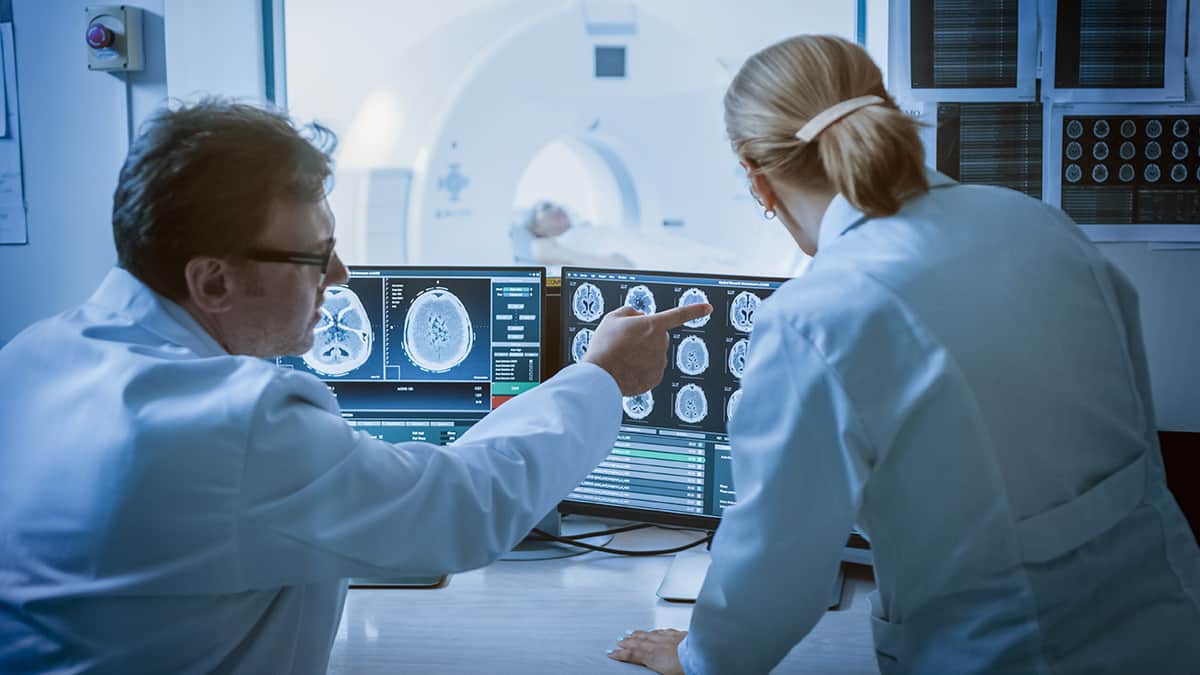
Conventional model-based image reconstruction (MBIR) for X-ray CT is often formulated as an optimization problem, the solution of which is the unknown image to be reconstructed.
Research in the past few years has shifted to replace components of these conventional MBIR methods by deep neural network models. Such integration can provide both improved image quality and certain interpretability of the deep learning architecture.
Jingyan Xu will present some existing approaches combining deep learning and MBIR, and discuss their strengths, weaknesses, and possible future extensions.
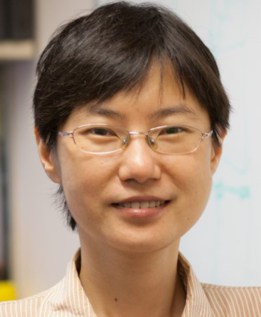
Jingyan Xu obtained her PhD in electrical engineering from Stanford University. She is currently an assistant professor in the Department of Radiology at Johns Hopkins University. Her area of expertise lies in developing model-based image reconstruction methods and task-based image-quality evaluation for X-ray CT. More recently, she has been working on synergistic integration of deep learning and model-based reconstruction for CT image generation.
Speaker relationship with IOP Publishing
Co-author of a recently published Physics in Medicine & Biology topical review, Convex optimization algorithms in medical image reconstruction—in the age of AI.
Want to learn more on this subject?
Why not watch one of our other webinars that we held during AI in Medical Physics Week.