Rachel Brazil investigates how physicists are trying to predict the dynamics of shifting opinions using laws that describe the physical world
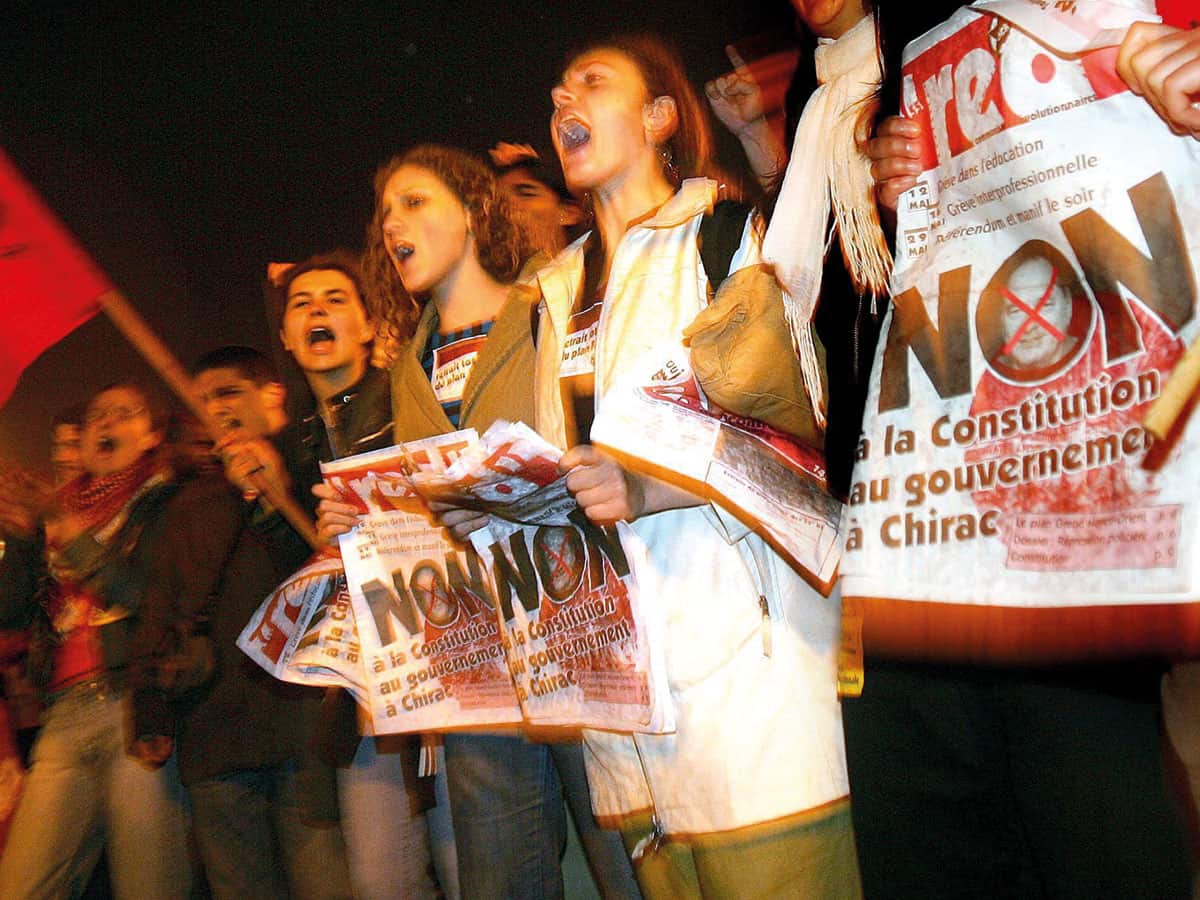
In August 2016 the French theoretical physicist Serge Galam published a paper explaining Donald Trump’s unexpected victory in that year’s US Republican primary election. His model also suggested that Trump could win the November presidential election – a view not then supported by analysts or polls (International Journal of Modern Physics B 31 1742015). Galam, who is seen by some as the father of the emerging field of “sociophysics”, is convinced that the dynamics of opinions obey discoverable universal quantitative laws and can be modelled in the same way that scientists model the physical world.
Now based at the Paris Institute of Political Studies in France, Galam has spent most of his career in physics departments studying disordered systems. As a student in the 1970s, he began trying to apply statistical physics to social models, but initially this work was treated with suspicion by other physicists. “People were very hostile,” Galam recalls. “Until today, I don’t really understand why.” His faculty head even confiscated Galam’s first paper in the area to try and prevent him publishing it.
Galam’s track record in predicting political surprises goes back further than Trump. In 2005, foreshadowing Brexit, he correctly predicted the “No” result in that year’s French referendum on establishing a European Constitution (55% of voters rejected the treaty on a turnout of 69%). Galam warned before the vote that “even starting from a huge initial majority of people in favour of the European Union, an open and free debate would lead to the creation of a huge majority hostile to the European Union”. He says these same dynamics were at play with Brexit.
Physics models
Over the last 15 years many physicists have become interested in using physics models to analyse trends in public opinions. Indeed, there is now a growing sociophysics community, which is ultimately trying to explain and predict why political outcomes sometimes seem to come out of nowhere.
The growing sociophysics community is trying to explain and predict why political outcomes sometimes seem to come out of nowhere
One of those involved is Daniel Stein, a physicist from New York University in the US whose research focuses primarily on randomness and disorder in magnetic materials. He is working with social psychologist Mirta Galesic from the Santa Fe Institute in New Mexico to develop what Galesic describes as “cognitively enriched models from statistical physics”. As is the case for many sociophysics models, Stein and Galesic are using the mathematical modelling of ferromagnetism as the basis of their work – specifically, the Ising model.
The Ising model is typically depicted as a 2D lattice with – in the case of magnetic materials – a particle at each point on the grid. Each particle can be in one of just two states: spin “up” or spin “down”. The spins like to line up in parallel with their neighbours because doing so lowers the overall energy of the system. However, this action competes against thermal noise, which injects fluctuations that tend to destroy order. At low temperatures, long-range ordering is established, but above a critical temperature the system becomes disordered. Turns out this basic model works for many physical systems and has gone way beyond magnetism. “It is probably one of the most intensively used models in physics,” Stein points out. In sociophysics, for example, it is used as a simple model of opinion dynamics, where networks of people are interacting and influencing each other.
Another model that has been applied to sociophysics is percolation theory, which is used in disparate fields from physics to epidemiology. One of those to follow this route is Hernan Makse – a physicist from the City College of New York. He has a background in the oil industry where percolation theory is used to understand how water flows when it’s pumped into rocks to extract oil. “This is a typical percolation problem because you are solving a problem of connectivity – in this case it is the percolation of water through a porous media, which is the rock,” he says. In a similar vein, Makse now uses percolation models to describe the diffusion of information and its impact on people’s opinions.
Modelling opinion
So can these models really have any relevance to human behaviour and how we form our opinions? Stein admits that social systems are far more complex than individual atoms or spins, but there are commonalities. “It’s not just the properties of the agent themselves but the interactions between the agents and this is where the statistical mechanics comes in,” he explains. “That’s because the physical mechanics is all about the interactions of individual particles and spins and external fields.” Just as with atomic spins, the way in which human opinions change is linked to our surrounding networks and can be modelled as any collective phenomena with interacting “agents”, be they people or atoms.
Opinion-dynamics models look at interactions within networks and how these are likely to cause change – usually between two opposing opinions such as voting for a left or right wing political party. The models create successive rounds of “updates” with opinions shifting after each round. “There is of course a fine line between using a model as a framework to guide your exploration and interpreting this too literally,” says Galesic. “They shouldn’t be taken as a direct description of humans and human society – of course humans are not particles living in crystal lattices!” But the models do seem to produce patterns of opinion spread that resemble the real world. Galesic continues, “These very simple models have found a way to derive these patterns using only a few parameters.”
When Galesic and Stein use a form of the Ising model, opinions are modelled as up or down spins that can change in line with their coupling to surrounding spins – the network of people to which they are exposed. The likelihood of a spin change will also depend on intrinsic predispositions such as political leanings that affect the likelihood of changing beliefs. “Every particle can have a different internal magnetic field, which in our case is an analogy for all their [prior] beliefs and values,” says Galesic. She explains the psychology behind this: “If believing in anthropogenic climate change is an up or down spin, my internal magnetic field would be my political orientation – so if I am a Republican I would be less likely to accept such a scientific fact at all.”
To test their theoretical framework, Galesic and Stein used opinion data on politics and health collected from 80 students in an undergraduate dorm at the Massachusetts Institute of Technology (MIT) (Physica A 519 275). One aspect they were looking to test was the mechanisms by which agents – the students – changed their opinions between successive surveys during 2008 and 2009. In sociophysics models there are different ways this can be modelled algorithmically, one of the most common being the “majority rule” where a person adopts the views of the majority within their local network. Alternatively, with the “expert rule”, people follow a particular individual whose opinion they value. Galesic and Stein showed that it’s not possible to use one rule in all circumstances and that how people behave is context-dependent. So in their study both rules were being used, depending on the kinds of questions being asked. “Things like playing sports once a week, eating salads versus unhealthy foods – we found that the majority rule worked best. So if your friends were going to exercise, you might too,” says Stein. But when asked about scientific questions, people are more likely to follow someone they consider an expert.
The types of networks modelled also make a difference. In physics it is common to use a Euclidean lattice (a 2D array of points), but that is not realistic for social networks. One model often used instead is the small-world network, which creates clusters and results in strangers being closely linked. In the MIT study, Galesic and Stein constructed real social networks from survey responses and by using Bluetooth and WiFi signals that indicated participants’ actual proximity. In fact, this Bluetooth network turned out to provide the most accurate model – even though it didn’t always match people’s own ideas of their networks.
Phase transitions and tipping points
The crucial aspect of the models borrowed from physics is their ability to identify phase transitions – when a system shifts from one phase of collective organization to another. A common example is how water changes state as a function of temperature and pressure. “These transitions are what we see in societies as well,” says Makse. However, the phase changes are not caused by temperature or pressure, but by changing distributions of opinions, which can be caused by various societal factors.
With colleagues from Brazil, Makse has looked for these kinds of phase transitions in real public opinion taken from over 10 years of data from the Pew Research Center in the US, on a range of political issues from immigration to abortion. The researchers were particularly interested in looking at how opinion becomes more extreme and if the numbers of people holding extreme opinions can cause “tipping points”, which – once reached – cause nonlinear changes and even greater take-up of extreme views across the whole population.
The conditions for a society to occupy moderate, incipient extremist, or extremist phases varies with the fraction of those who hold extreme views
Makse and his colleagues have created “social phase diagrams” that show the conditions for a society to occupy moderate, incipient extremist, or extremist phases (where a majority hold extremist views), and how this varies with the fraction of those who held extreme views. One example of such a transition was in opinions on how people rated the economic situation in various countries including France, Italy, Greece and Spain. Rather than overall views changing linearly as the proportion of those who considered the situation to be “very bad” increased, a point arose where the relationship between overall opinion and the proportion of those expressing extreme opinions was no longer linear. Instead, overall opinion on the economic situation became more negative than expected (figure 1). This occurred just after the 2009 European sovereign debt crisis (Sci. Rep. 5 10032).
1 A social tipping point
Outcomes of 260 polls in 59 countries, where f is the fraction of people sharing an opinion (rating the economic situation in their country as either “bad” or “very bad”), and fe is the fraction sharing an extreme opinion (rating the economy only as “very bad”). The dotted line depicts the linear behaviour expected for a non-interactive group. The polls in Spain are highlighted with black squares to demonstrate time evolution. There is a clear tipping point where fe diverges from expectations in 2009.
A surprising finding was that to reach the tipping point, in some cases only 20% of a population need initially hold extreme views to sway others and eventually create an extreme majority. This seemed difficult to understand from both a physical and psychological perspective until the researchers factored in differences in people’s natures, similar to Galesic’s “internal field” concept. Specifically, they were able to model the onset of cascades of extreme views when they included “stubbornness” – clearly some people are less likely to change their minds than others, which becomes a crucial ingredient to the model. “We played with these thresholds to see how many stubborn people we needed in the model so that [people’s] ideas do not change,” says Makse, “All sorts of nice dynamics come up.”
Similarly, Galam’s model of opinion dynamics also incorporates stubbornness on each competing side, and is consequently able to predict the value of the corresponding tipping point with any initial proportion of opinions in a population. As few as 2% more stubborn agents on one side puts the tipping point at a very low value of around 17%, which leads to the unfortunate conclusion that to win a public debate, what matters is not convincing a majority of people from the start, but finding a way to increase the proportion of stubborn agents on your side (Physica A 389 3619).
Majority rules yielding minority spreading
Galam has spent most of his career explaining the kind of unexpected results that seem to periodically arise in politics and public debate. He ascribes this to “minority opinion spreading”, where an initial vocal minority turns a majority towards its position. Rather than being a response to a specific political issue, Galam says this is a sociological phenomenon that can be modelled along a similar path used in statistical physics to reach global behaviour from local interactions.
Galam’s model uses a majority-rule-based approach, with repeated local small group discussions occurring within the network. Think of these as the real-life lunches, coffee breaks and dinner parties where people discuss the news. During these encounters, people change their views to follow the local majority, unless faced with an equal opinion split within the group. In the latter case, people are likely to support the position that offers the least change or is in tune with their unconscious prejudices (Eur. Phys. J. B 25 403). As a result, over a number of updates and continuing debates, opinions shift to support the status quo or the opinion in tune with the activated prejudices.
An opinion that starts in the minority can quickly spread as long as it is above a base threshold. Galam suggests this threshold is just above 23% for groups of four people. In other words, although some groups of four start with no-one holding the minority view, over a series of updates a person will encounter split groups, causing a switch to the minority position. And after each update, the proportion of people holding the minority view will increase. Getting to a final point happens remarkably quickly. “In all cases the number of updates is less than 10,” Galam says. However, transposing that to real time would need an understanding of the speed of each update, which will increase, for example, as an election comes closer. One update could be between a few days and one to two weeks.
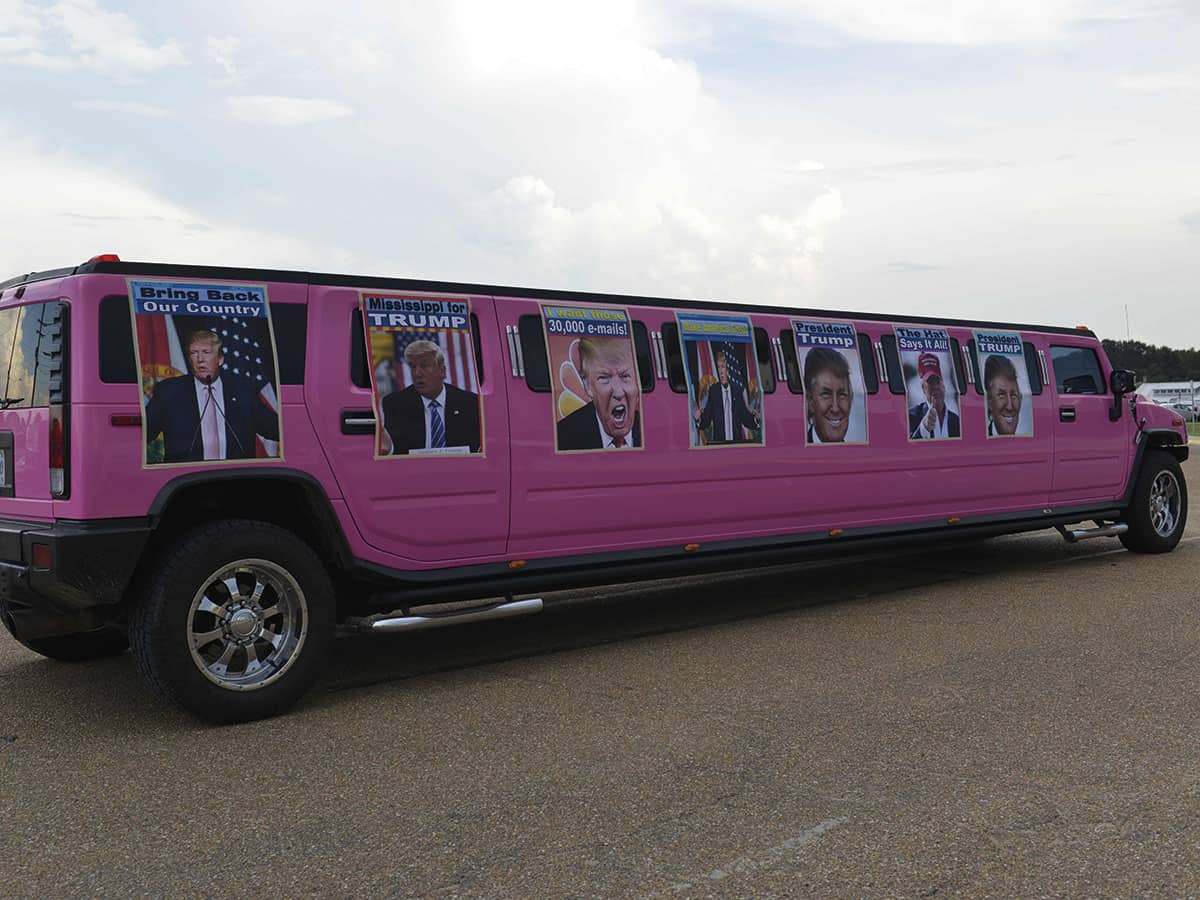
In the case of the 2016 US presidential elections, Galam says the prevailing factor was peoples’ “frozen prejudices”. He argues that Trump’s outrageous statements, though initially seen as repellent by most voters, managed to activate their hidden or unconscious prejudices. First, many Trump supporters shifted to Hillary Clinton, rejecting his statements with great outrage, leading to a decrease in support. But the initial outrage led to more public debates with an automatic increase in the number of local ties. At those points, “it’s like flipping a coin, but with a coin biased along the leading prejudice”, Galam says. Then many voters started to swing in favour of Trump.
However, the model does require a minimum support for Trump above the corresponding tipping point value. “To stay above the threshold, it is thus a combination of being able to modify the unconscious reality by activating old frozen prejudices, at the price of losing support, but still preserving a nucleus of people who are consciously OK with the prejudice,” explains Galam. Although the threshold value can be as low as 17%, the second requirement was not satisfied everywhere, which explains why Trump did not win in all US states.
Opinion polls, big data and statistical models
If Galesic has a criticism of past work in sociophysics, it is that many models were designed in isolation without reference to empirical measurements of real social groups. She carried out her own longitudinal survey of US public opinion to test her model, surveying 94 people in four waves in 2016. Participants were asked about their beliefs on guns, terrorism and vaccinations, and the survey followed how their views changed over the four waves. Galesic factored in information on peoples’ networks and their initial values to create a quantitative understanding of the likelihood that they would change their opinion. The resulting model could reproduce the real-world pattern of belief changes found in the survey.
For both the 2016 US and 2017 French presidential elections, Galesic asked interviewees not only who they would vote for, but also the voting intentions of other individuals in their social circle. Using this information calculated the likelihood that any individual would change their mind. Adding this information did seem to improve the accuracy of predictions (Nature Human Behaviour 2 187) and Galesic also used this approach for the 2018 US mid-term elections, predicting a 9% Democratic lead over the Republicans. This turned out to be very close to the actual Democratic lead of 8.6% and a better prediction than just asking people about themselves (12%).
Physicists and computer scientists are also looking at social-media data, which is being used together with machine learning to make predictions about people’s opinions and voting intentions. This might in fact provide quicker and cheaper information than opinion polls. Prior to the 2016 US presidential elections, for example, Makse analysed over 170 million Tweets, using a combination of network analysis, natural language processing and machine learning (Scientific Reports 8 8673). The results proved to be remarkably similar to the New York Times National Polling Average – an aggregate of hundreds of independent polls, but 10 days ahead – without the lag-time needed to complete a survey.
This approach does have its limitations says Makse. “You are just making statistical inferences, but you do not know why – it’s a black box, you won’t learn anything about society. If you want to actually change the situation, you need to do that with physics and understand the mechanism.” As he adds: “For a physicist it is more fun to find the fundamental laws behind those predictions.”
If you want to actually change the situation, you need to do that with physics and understand the mechanism
Galam agrees that statistical physics can provide unique insights, particularly when it comes to nonlinear effects and “phase changes”. “Big data will not show you a sudden change that’s rapid before it occurs because it is not there beforehand – you need a theory to do that.” Galam sees the unique power of sociophysics as its ability to predict the sort of nonlinear behaviour that opinion polling will never predict and that often triggers large political or social changes. “I think we will be able one day to predict on solid ground, co-operative phenomena like an election, or a social movement over an event timescale of a few weeks or a few months,” he says.
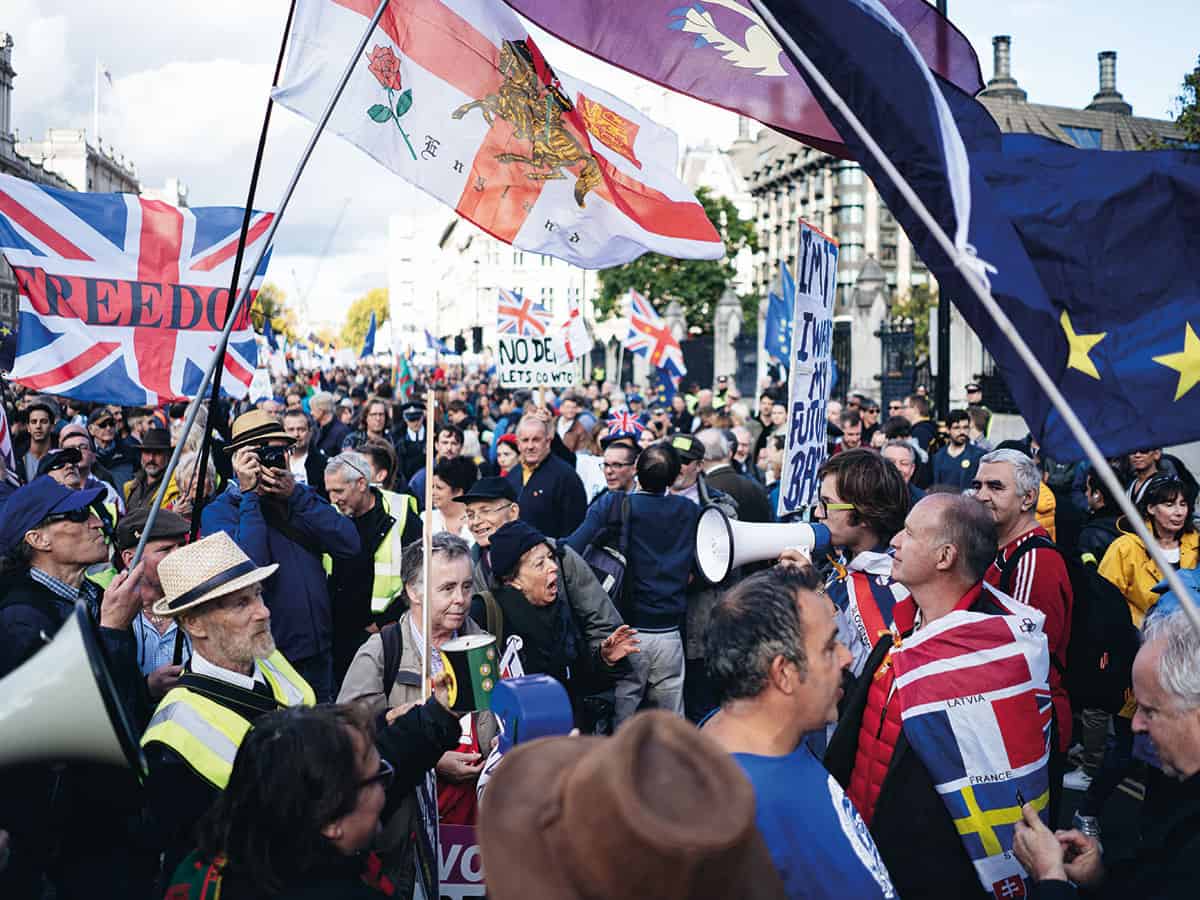
With Taksu Cheon, a physicist from Kochi University of Technology in Japan, Galam has succeeded in incorporating all his models into one unique update equation. “We now have all the parameters together so I have a kind of universal formula,” says Galam. “It gives me – for any proportions of inflexible individuals, contrarians, distribution of prejudices and for any size discussion groups – the relevant tipping point, which will eventually drive the final outcome, so this is a very strong step forward.” All possible opinion dynamics outcomes are now included within a phase diagram embedded in a parameter space of six dimensions (arXiv:1901.09622).
And while social circle perceptions have improved prediction, Galesic says they are a step away from creating a model that could predict how each individual surveyed is likely to behave. “That’s the ultimate frontier in social science, to try to predict human behaviour,” she suggests. But if this was ever possible, what does it tell us about our own free will? Perhaps our opinions are not formed as freely as we think. “We are taking in dynamics that we don’t see,” says Galam. “Only by identifying our hidden determinisms will tomorrow allow us to reach more free will, so this is why I think it’s very important to push in the sociophysics direction.”
As for Galesic, she thinks this kind of research can actually help us to have more freedom. “If these models show that our social contacts and our pre-existing moral values influence how likely it is that we will believe a potentially useful piece of information, then we should periodically re-examine who we socialize with and what we believe in.”