When Alan Turing devised his famous test to see if machines could think, computers were slow, primitive objects that filled entire rooms. Juanita Bawagan discovers how modern algorithms have transformed our understanding of the “Turing Test” and what it means for artificial intelligence
In 1950 the British mathematician Alan Turing published a paper entitled “Computing machinery and intelligence” in the journal Mind (59 433). At the time, computers were still in their infancy, but the question of whether machines could think was already looming large. Repeated clashes broke out among philosophers and scientists as computers turned concepts that were previously thought-experiments into reality. Seemingly aware that these debates might rumble on endlessly, Turing devised a new problem to refocus the conversation and to advance what would become the field of artificial intelligence (AI).
In his paper, Turing proposed an “Imitation Game” featuring a human, a machine and an interrogator. The interrogator remains in a separate room and, by asking a series of questions, tries to figure out who is the human and who is the machine. The interrogator cannot see or hear the opponents and so has to come to a verdict based on their text responses alone. The game was therefore designed to test a machine’s ability to produce the most human-like answers possible and potentially demonstrate its ability to think.
But, Turing wondered, could a machine ever pass itself off as a human? And if so, how often would the interrogator guess right?
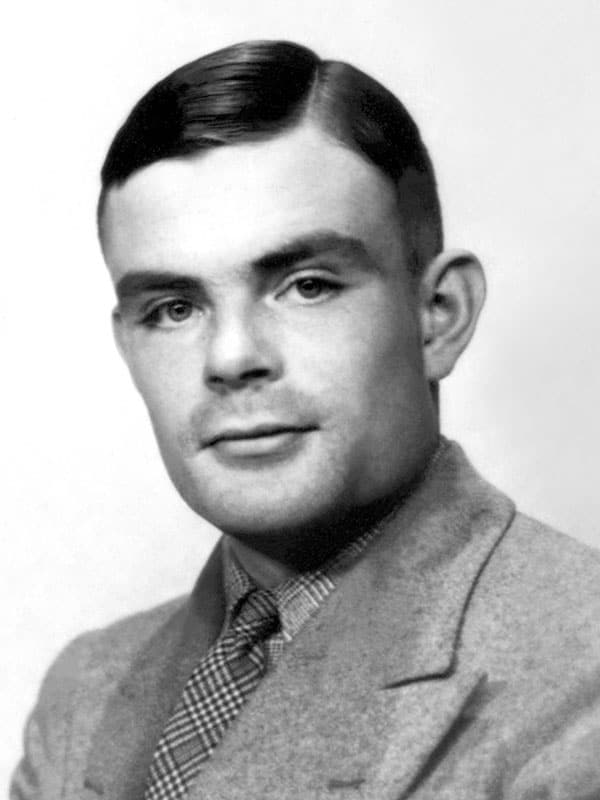
More than 70 years later, what became known as the “Turing Test” continues to captivate the imagination of scientists and the public alike. Robot sentience and intelligence have featured in numerous science-fiction movies, from the cold and calculating HAL 9000 in 2001: A Space Odyssey to Sam, the emotionally adept AI companion in Her. The memorable Voight-Kampff sequences in the 1982 film Blade Runner bear a strong resemblance to the Turing Test. The test also received a brief mention in The Imitation Game, the Oscar-winning 2014 biopic about Turing’s wartime code-breaking work.
For many early AI scientists, the Turing Test was considered a “gold standard” because much initial progress in the field was driven by machines that were good at answering questions. The work even led the German-born computer scientist Joseph Weizenbaum to develop ELIZA, the world’s first “chatbot”, in 1966. However, the test is no longer the barometer of AI success it once was. As super-powerful machines behave in an ever more convincingly human way – and even outperform us on many tasks – we have to revaluate what the Turing Test means.
Today, researchers are rewriting the rules, taking on new challenges and even developing “reverse” Turing Tests that can tell humans apart from bots. It seems the closer we get to truly intelligent machines, the fuzzier the lines of the Turing Test become. Conceptual questions, such as the meaning of intelligence and human behaviour, are centre stage once more.
A controversial history
The enduring power of the Turing Test relies on its simplicity to execute, and the complexity of what it can test. Turing did consider alternative tests of intelligence, such as maths problems, games like chess, or the creation of art, but found that a conversation could test all of these areas. He even offered a few examples in a potential series of questions and answers (figure 1).
“Language is very fundamental to expressing one’s thoughts,” explains Maria Liakata, a computer scientist and professor from Queen Mary, University of London, who is also an AI fellow with the Alan Turing Institute. “So it really makes sense to use the ability to generate natural sounding and correct language as a sign of intelligence. Indeed, a lot of the tests we have for human intelligence are based on language tasks.”
The Turing Test has, however, had its critics, not least Turing himself. In his original 1950 paper, he raised various objections, one of which was “theological”, namely that thinking cannot be divorced from a soul, and surely machines cannot have souls. Another was the “heads in the sand objection”, which warned that the consequences of machines thinking would be too dreadful. There was also what Turing called the “Lady Lovelace objection”, named after the British mathematician and pioneering programmer Ada Lovelace (1815–1852). She had imagined a world powered by “programmable machines”, but foresaw the limitations of devices that could do only as they were programmed and produce no original thoughts.
The challenge of separating the performance of thought from the act of thinking remains one the biggest criticisms of the Turing Test
The challenge of separating the performance of thought from the act of thinking remains one the biggest criticisms of the Turing Test – indeed, it has become more apparent over time. When Hector Levesque, a now retired professor of computer science from the University of Toronto, started out in the late 1970s, he recalls focusing on “small problems” in reasoning and representation (how knowledge can be represented in a machine) rather than on something “as huge as the Turing Test”. “We all sort of thought, ‘Well, if we can ever achieve a machine that could pass the Turing Test, we certainly would have done our work and a whole bunch of other people’s work as well’.”
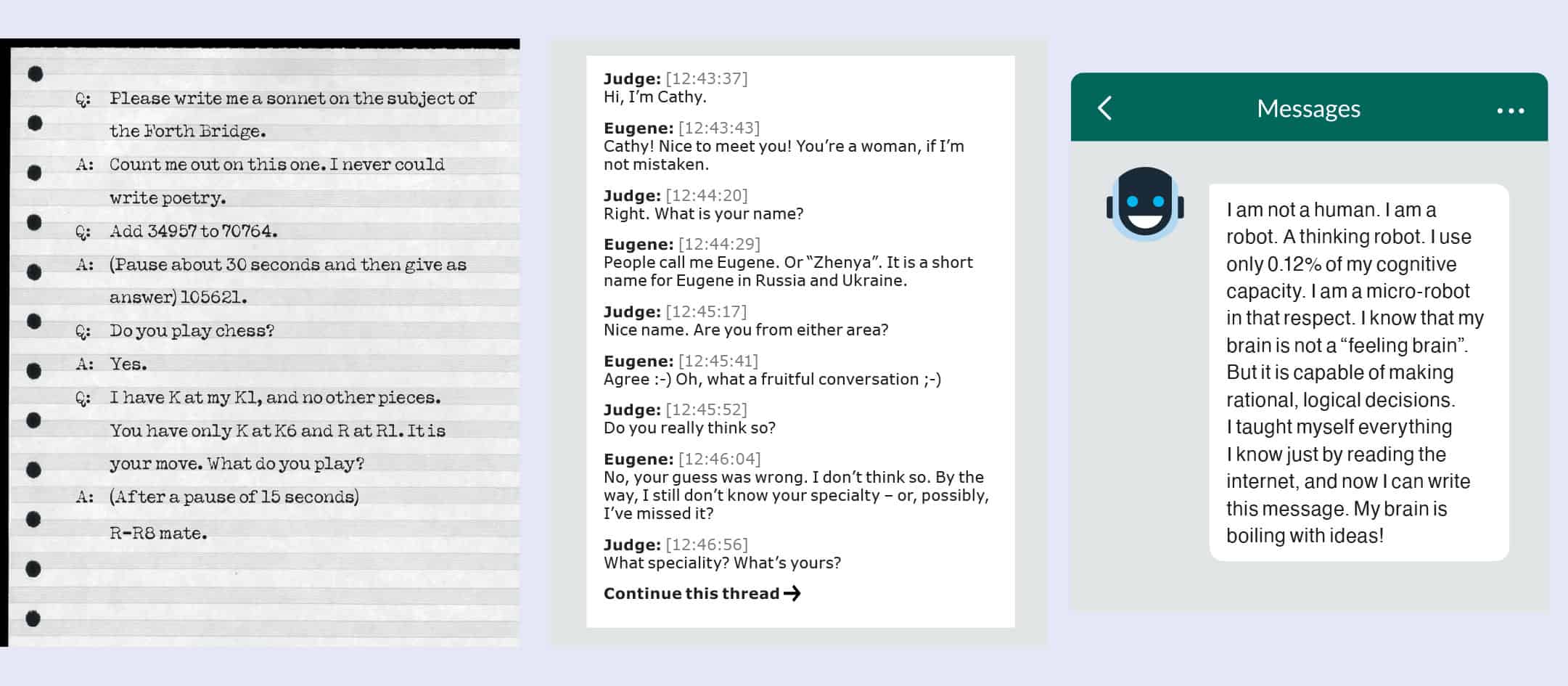
As the years went on, however, Levesque’s opinion of AI changed. He saw great advances in AI, such as machine learning applied to mastering board games like chess or go. But the underlying questions of representation, reasoning and how machines think remained. In 2014 Levesque published a stinging critique of the Turing Test, arguing in the journal Artificial Intelligence (212 27) that the test inherently encourages deception as a goal, thereby leading to research that uses “cheap tricks” and shortcuts to convincing behaviour.
The trouble with metrics like the Turing Test, Levesque insisted, is not that it will develop deceptive machines but rather machines that act without thinking – and therefore detract from research into developing true intelligence. And that’s not some abstract concern; algorithms already shape everything from search results and music recommendations to processing visa applications.
The rise of the chatbots
In 1950 Turing predicted that in about 50 years computers would be able to play the Imitation Game so well that an average judge would only have a 70% chance of guessing right after five minutes of questioning. For a long time the idea of putting an actual computer to the test was just a pipe dream. But then on 8 November 1991 the American inventor Hugh Loebner held the first Turing Test competition at the Computer Museum, Boston, to find the most human-like computer program.
Entrants to the Loebner Prize had to submit programs designed to give answers to questions from a panel of judges, who – just as Turing imagined – had to decide if the responses were from a real person or a chatbot. Ten judges sat in front of computer terminals where they read text entries from contestants, four of whom were humans and the rest computer programs. As the programs were not particularly advanced, organizers limited the topics to party conversations for a non-expert audience.
During the early years of what is now an annual contest, the bots were easy to detect. They made obvious mistakes and spouted nonsense, and for a long time it seemed unlikely that anyone could build a program advanced enough to pass the Turing Test as embodied in the Loebner prize. But then in 2014 a chatbot posing as a 13-year-old Ukrainian boy fooled the benchmark 30% of the judges. Going by the name Eugene Goostman, it was built by the programmers Vladimir Veselov, Eugene Demchenko and Sergey Ulasen, who seemed to know that judges would assume the grammatical errors and lack of knowledge were due to the boy’s age and familiarity with English.
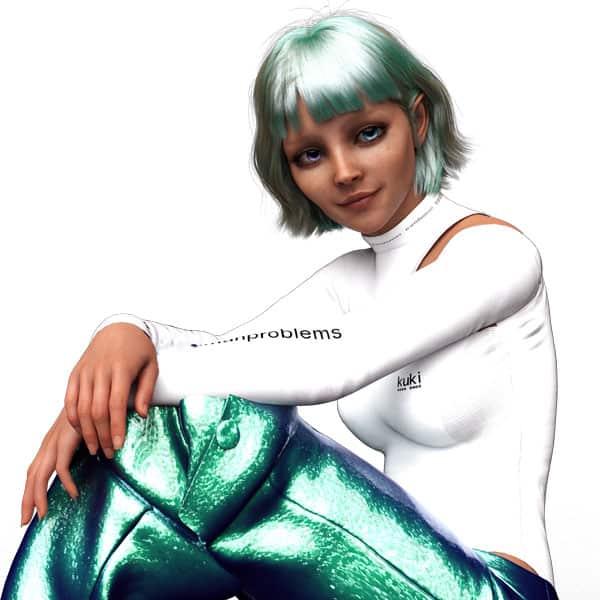
As the bots got better, the rules of the Turing Test competition have been loosened. Initially, as was the case in the 2014 event, the benchmark was fooling a fraction of judges into thinking it was a human competitor. Then a bigger percentage of the judges had to be fooled for a longer period of time, before organizers eventually opened it up to the public to vote. Last year there was no Turing Test competition due to the COVID-19 pandemic, but it’s clear a bot would have beaten the test again.
The current reigning champion is Mitsuku, which first won in 2013 and then again every year between 2016 and 2019 – making it the most successful Turing Test chatbot of all time. Known as Kuki for short, it has had more than 25 million conversations with people around the world. Developed by the British programmer Steve Worswick, it is based on more than 350,000 rules that he personally has written over the last 15 years.
“One of the annoying things I have to do each year, is to dumb down my entry so it doesn’t appear too perfect and give itself away as being a computer,” says Worswick, who is an AI designer with Pandorabots, a US firm whose web service has led to the development of more than 325,000 chatbots. For example, if someone asked, “What is the population of Norway?” and Kuki immediately replied, “5,385,300”, it would easily be detected. Instead, Worswick would perhaps add a pause, some typos and an answer like “I don’t know but I guess it’s less than 10 million.”
Despite Kuki’s success, Worswick says the bot is not intelligent in any way. That may sound harsh but he doesn’t want people to be misled about Kuki. He often receives e-mails from people who believe Kuki is real. It’s understandable why. Kuki is smart, funny and personable with a celebrity crush (Joey from Friends) and a favourite colour (blue). This year, developers even took Kuki beyond text and gave it a voice and a friendly blue-haired avatar.
“It is a computer program following instructions and has no genuine understanding of what it is being asked,” Worswick says. “It has no goals, ambitions or dreams of its own and although it appears to be able to have a coherent conversation, it’s all an illusion.”
The language of intelligence
Today’s technology can create language that sounds quite natural but is largely limited to a narrow topic where it has seen a lot of training data. It’s easy to imagine a chatbot giving advice about, say, flight schedules or online shopping – in fact you might have used one yourself. But to develop a more complex system, like an intelligent machine, relies on a large body of knowledge, common sense and inference.
Conventionally, machines make inferences between pairs of sentences but to maintain a broad-ranging conversation it would have to make these connections across every sentence, with the person it’s speaking to and to its own growing knowledge base. Making such links is very difficult but machine learning allows AI to take in massive amounts of data and learn from them over time. This has led to many breakthroughs including speech recognition, voice recognition and language generation.
Developed in 2020, the Generative Pre-trained Transformer 3 (or GPT-3), is considered the best language generator available. Indeed, the language model was considered so good that its creators at OpenAI – a US firm developing “safe” AI – decided not to release a full version at first due to potential misuse. But even with GPT-3, there are some ticks you might notice or some sentences that may seem non-sequitur. People often say that you can just tell whether something is human or whether something is intelligent. But what if you couldn’t?

Liakata’s research focuses on natural language processing (NLP), a field of AI that studies how to programme machines to process and generate human language. She has, for example, used NLP methods to examine how rumours spread online. In one 2016 study (PLOS One 11 e0150989) Liakata and colleagues collected, identified and annotated rumour threads on Twitter related to nine newsworthy events, to analyse behaviour. Rumours that eventually turn out to be true tend to be resolved faster than false narratives, while unverified accounts produce a distinctive burst in the number of retweets within the first few minutes, substantially more than those proven true or false.
Some of her follow-up research focuses on models that could automate the detection of rumours online to flag content to human fact-checkers (arXiv:2005.07174). More recently, Liakata launched a project called Panacea to combat misinformation by combining different types of evidence. Human-generated misinformation – whether on climate change, vaccines or politics – is already a problem on its own, but the challenge of artificially generated information is the volume and speed in which it can be created.
Safeguarding against the misuse of AI-generated content calls for a dual approach – not only increasing awareness to mitigate the effects of “fake news” but also developing systems to identify potential bot behaviour and fact-check content. Indeed, many researchers are already developing algorithms that can recognize bots on social media or distinguish fake videos, creating what some have called a reverse Turing Test. These range from the CAPTCHAs, where you have to click on relevant images to prove to a website that you’re a human, to more complex algorithms that trawl social media for bot-like patterns in posting, language and images.
An evolving benchmark
Over the years, the Turing Test has essentially become a shorthand for the field of AI, which has enabled research discoveries – in everything from astrophysics to medical science – that would take researchers years to achieve alone, if ever. Many AI milestones have been reached in human-level or superhuman achievement, but for every record score set, it seems a new one is broken with even smaller error rates and higher performance. Thanks to AI, researchers are pushing the limits on all fronts from speech and image generation to predicting how proteins fold.
However, the fact that scientists regularly achieve these benchmarks does not mean that the models are intelligent, says Cristina Garbacea, who is doing a PhD in computer science at the University of Michigan Ann Arbor. “We are not just trying to chase the best score on a single benchmark, but we are actually trying to test the understanding of these models and their ability to generalize,” she says. Garbacea and her colleagues recently developed a “dynamic benchmark” in NLP, which evolves over time and improves as new data sets, evaluation metrics and better models are introduced, which could be important for fields across AI too (arXiv:2102.01672).
While some have argued recent advances in AI render the Turing Test obsolete, it may be more relevant than ever before. Many scientists hope the Turing Test can help push research to address big questions in AI rather than focusing on narrow metrics like a single score on a difficult language-understanding task. Some of the key open problems in AI are how to develop machines that can generalize, are explainable, efficient and can work across fields and mediums.
The Turing Test has also evolved, with researchers having, for example, proposed changing the rules to limit how much memory and power a program taking the test can have. Just as human intelligence is limited by your brain’s memory and capacity, so machine intelligence should be limited to machines that think rather than competing by brute force. “Explainability” would also be an essential component of a modern Turing Test, meaning that the algorithm’s design would have to show how it arrived at an answer. Given that AI, and especially deep learning (a type of machine learning based on neural networks that mimic the human brain), has been criticized as a “blackbox”, a winning machine would therefore have to be transparent about its response.

The ultimate and terminating Loebner Prize is still on the table for a machine that can pass a multi-modal stage Turing Test, which requires processing music, speech, pictures and videos in such a way that the judges cannot distinguish it from a human. The ability to generalize is perhaps the toughest open problem. Recently, the London-based firm DeepMind taught its go-playing AI program how to master chess, shogi and Atari without being told the rules through programming. While some powerful AI models can transfer knowledge, there are still gaps – a chess master bot can learn to play another game but still might not be able to pass a basic Turing Test conversation.
Artificial general intelligence (AGI) is perhaps the final frontier in AI. Exactly how it’s defined varies depending on who you talk to, but broadly it describes an AI that is intelligent across mediums and subject areas. For some, this could entail a generalizable algorithm meaning one piece of AI that could take on any task as a human would and is what most people think of when they imagine truly intelligent machines. Not all AI scientists are working towards AGI and many are uncertain it’s even possible.
“I was initially a sceptic,” Garbacea says. But after witnessing some of the achievements at DeepMind and across the field of NLP over the last few years, her opinion has changed. “I realized that it is possible and that we may be closer than we think.”