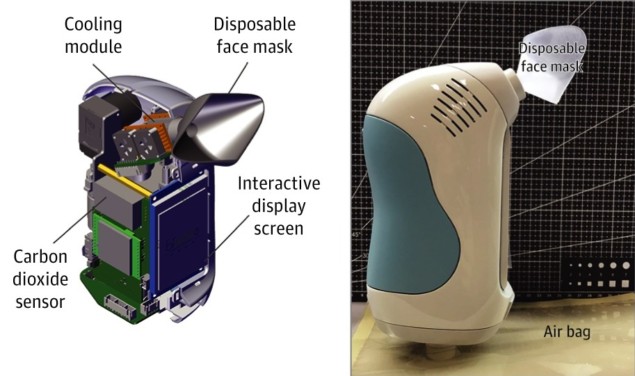
Breathe in, breathe out. Breathe in, breathe out.
This reflexive action that is indispensable to life may also be a way to detect cancer – each breath we exhale contains thousands of molecules that provide information about our health.
Detecting lung cancer
Lung cancer was the leading cause of cancer-related deaths worldwide last year. And because symptoms often don’t appear until the disease is advanced, detecting lung cancer early is critical.
Currently, doctors screen people who are at high risk of getting lung cancer using X-ray or low-dose computed tomography (CT) images. Though research suggests that low-dose CT may reduce lung cancer-related mortality by 20%, with recent studies confirming this result, low-dose CT has some risks, including frequent false positives that suggest a person has lung cancer when they do not.
Enter exhaled breath tests, which can identify more than 1000 substances in exhaled breath.
Mantang Qiu, a thoracic surgeon and researcher, and his colleagues at Peking University People’s Hospital combined high-pressure photon ionization time-of-flight mass spectrometry (TOFMS) and a support vector machine algorithm to classify patients as having lung cancer or not only using patients’ exhaled breath. They report the results of their research in a JAMA Network Open paper.
Accelerating electrons to analyse exhaled breath
Unlike other breath tests that try to detect cancer, high-pressure photon ionization TOFMS is sensitive and fast and detects exhaled breath directly, without any pre-processing.
The technique “can sniff the ‘fingerprint’ of each breath sample and tell which one is from lung cancer,” explains Qiu.
In the high-pressure photon ionization TOFMS system (manufactured by Shenzhen Breax Biological Technology Company Ltd), a vacuum ultraviolet lamp bombards an exhaled breath sample with electrons, ionizing some of the exhaled breath molecules. Then, an electric field accelerates the ions toward a detector, which measures the time it took the ions to reach the detector. With these time-of-flight data, the ions are separated according to their mass-to-charge ratio. Researchers can then use mass spectra created using the time-of-flight data to identify molecules and chemical compounds in each exhaled breath sample.
Detecting lung cancer with high-pressure photon ionization TOFMS
Qiu and his colleagues collected exhaled breath samples from 139 patients who had lung cancer and 289 people who did not, according to CT and low-dose CT imaging scans, respectively. Those people with lung cancer then had surgery to biopsy, remove and study their tumours. Low-dose CT images from people without lung cancer were reviewed first by an artificial intelligence program and then confirmed by a radiologist. People with benign pulmonary nodules in their lungs were not included in the study. Room air was also collected to ensure that environmental effects were not seen in the results.
The researchers processed all exhaled breath samples using high-pressure photon ionization TOFMS. Then, they put the results of the high-pressure photon ionization TOFMS studies and each participant’s lung cancer status (lung cancer or not) into a support vector machine – a supervised learning model that analyses and classifies data.
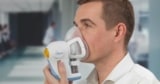
Clinical trial to develop breath test for multiple cancers
The researchers’ support vector machine performed well: it was accurate, always detected lung cancer, and estimated how likely people without disease could be correctly identified in a validation dataset. These results suggest that high-pressure photon ionization TOFMS and the support vector machine could be used to non-invasively and quickly identify people who have lung cancer without requiring CT machines or other equipment.
“This is the first step toward using high-pressure photon ionization TOFMS for lung cancer detection,” says Qiu.
The researchers now are working to identify specific molecules from exhaled breath that are unique to people who have lung cancer. They also want to integrate high-pressure photon ionization TOFMS into current clinical workflows.