To many physicists, “Tsallis entropy” has been a revolution in statistical mechanics. To others, it is merely a useful fitting technique. Jon Cartwright tries to make sense of this world of disorder.
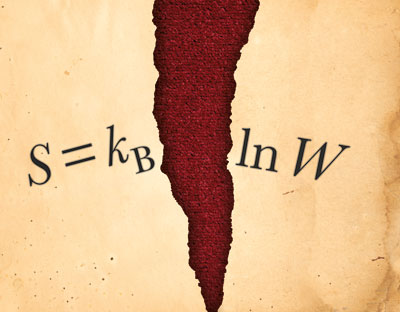
Physics may aim for simplicity, yet the world it describes is a mess. There is disorder wherever we look, from an ice cube melting to the eventual fate of the cosmos. Of course, physicists are well aware of that untidiness and have long used the concept of “entropy” as a measure of disorder. One of the pillars of physical science, entropy can be used to calculate the efficiency of heat engines, the direction of chemical reactions and how information is generated. It even offers an explanation for why time flows forwards, not backwards.
Our definition of entropy is expressed by one of the most famous formulae in physics, and dates back over a century to the work of the Austrian physicist Ludwig Boltzmann and the American chemist J Willard Gibbs. For more than 20 years, however, the Greek-born physicist Constantino Tsallis, who is based at the Brazilian Centre for Physics Research (CBPF) in Rio de Janeiro, has been arguing that entropy is in need of some refinement. The situation, according to Tsallis, is rather like Newtonian mechanics – a theory that works perfectly until speeds approach that of light, at which point Einstein’s special theory of relativity must take over.
Likewise, says Tsallis, entropy – as defined by Boltzmann and Gibbs – works perfectly, but only within certain limits. If a system is out of equilibrium or its component states depend strongly on one another, he believes an alternative definition should take over. Known as “Tsallis entropy” or “non-additive entropy”, it was first proposed by Tsallis himself in a 1988 paper (J. Stat. Phys. 52 479) that has gone on to become the most cited article written by a scientist (or group of scientists) based in Brazil. So far it has clocked more than 3200 citations, according to the Thomson Reuters Web of Science.
To many who study statistical mechanics, Tsallis entropy makes for a much broader view of how disorder arises in macroscopic systems. “Tsallis entropy provides a remarkable breakthrough in statistical mechanics, thermodynamics and related areas,” says applied mathematician Thanasis Fokas at the University of Cambridge in the UK. In fact, Fokas goes as far as saying that subsequent work motivated by Tsallis’s discovery has been “a new paradigm in theoretical physics”.
Tsallis entropy has, though, been divisive, with a significant number of physicists believing he has not uncovered anything more general at all. But the voices of these detractors are fast being lost in the crowd of support, with Tsallis’s original paper being applied to everything from magnetic resonance imaging to particle physics. So are these applications exploiting a truly revolutionary theory? Or to put it another way: is Tsallis to Boltzmann and Gibbs what Einstein was to Newton?
Old concept
Entropy as a physical property was introduced by the German physicist Rudolf Clausius in the mid-1860s to explain the maximum energy available for useful work in heat engines. Clausius was also the first to restate the second law of thermodynamics in terms of entropy, by saying that the entropy, or disorder, of an isolated system will always increase, and that the entropy of the universe will tend to a maximum. It was not until the work of Boltzmann in the late 1870s, however, that entropy became clearly defined according to the famous formula S = kB ln W. Here S is entropy, kBW is the number of microstates available to a system – in other words, the number of ways in which a system can be arranged on a microscopic level.
Boltzmann’s formula – so famous that it is carved on his gravestone in Vienna (as S = k log W) – shows that entropy increases logarithmically with the number of microstates. It also tends to class entropy as an “extensive” property – that is, a property, like volume or mass, whose value is proportional to the amount of matter in a system. Double the size of a system, for instance, and the entropy ought to double too – unlike an “intensive” property such as temperature, which remains the same no matter how large or small the system.
One example of entropy being extensive is a spread of N coins. Each coin has two states that can occur with equal probability – heads or tails – meaning that the total number of states for the coins, W, is 2N. That number can be entered into Boltzmann’s formula, but, given that an exponent inside a logarithm can be moved to the front of the same logarithm as a multiplier, the expression simplifies to S = NkB ln 2. In other words, the entropy is proportional to N, the number of coins, or matter, in the system; by Boltzmann’s definition, it is extensive.
Boltzmann’s formula is not, though, the final word on entropy. A more general Boltzmann–Gibbs formula is used to describe systems containing microstates that have different probabilities of occurring. In a piece of metal placed in a magnetic field, for example, the spins of the electrons inside are more likely to align parallel than antiparallel to the field lines. In this scenario, where one state (parallel alignment) has a much higher probability of occurring than the other (anti-parallel alignment), the entropy is lower than in a system of equally likely states; in other words, the alignment imposed by the magnetic field has made the system more ordered. Nonetheless, the entropy here is still extensive: double the electrons, double the entropy.
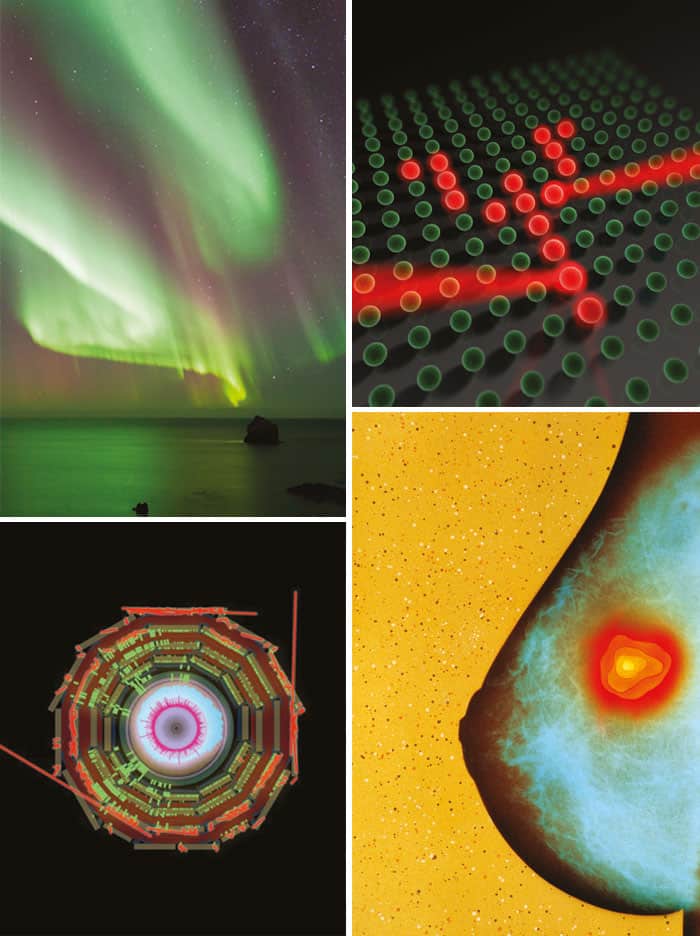
Unfortunately, it is not always possible to keep entropy extensive when calculating it with the Boltzmann–Gibbs formula, says Tsallis, and this, in his view, is the crucial point. He believes that entropy is extensive not just some of the time, but all of the time; indeed, he believes that entropy’s extensivity is mandated by the laws of thermodynamics. Calculations must always keep entropy extensive, he says – and if they ever suggest otherwise, those calculations must change. “Thermodynamics, in the opinion of nearly every physicist, is the only theory that will never be withdrawn,” Tsallis insists. “The demands of thermodynamics must be taken very seriously. So if Boltzmann–Gibbs entropy does not do the job, you must change it so it does do the job.” For Tsallis, thermodynamics is a pillar of physics and must not be tampered with at any cost.
As to why thermodynamics restricts entropy to being extensive, he says, there are two main arguments. One is a complex technical argument from large deviations theory, a subset of probability theory. But another, simpler, argument is based on intuition. Thermodynamic functions depend on one or more variables, which for most systems can be either intensive or extensive. However, it is possible to switch a function that depends on an intensive variable to a version that depends on a corresponding extensive variable, and also vice versa, by using a mathematical “Legendre transformation”. For instance, a Legendre transformation can switch a function for energy that depends on temperature to one that depends on entropy – and since temperature is an intensive variable, this implies that entropy must be correspondingly extensive. “The Legendre transformation is the basic mathematical ingredient that makes thermodynamics work,” says Tsallis. “And you quickly see that entropy must be in the extensive class.”
Systems in which the Boltzmann–Gibbs formula does not keep entropy extensive include those that are out of equilibrium, or where the probability of a certain microstate occurring depends strongly on the occurrence of another microstate – in other words, when the elements of a system are “strongly correlated”.
As an example of such correlation in statistics, Tsallis gives linguistics. Take four words almost at random, for example “one”, “many”, “child” and “children”, and you might expect to find, via probability theory, 4 x 4 = 16 possibilities for two-word phrases. As it happens, many of these possibilities are not permitted – you cannot say “one children” or “child many”. There are, in fact, only two syntactically correct possibilities: “one child” and “many children”. Grammar produces strong correlations between certain words, and so greatly reduces the number of allowed possibilities, or entropy.
There are other obvious examples in the physical world of strong correlations affecting entropy. In the presence of a whirlpool, for instance, water mole-cules do not take any path, but only those that give the overall resemblance of a vortex, because the mole-cules’ motions are correlated. And it turns out that in any system with strong correlations, the number of possible microstates, W, no longer increases exponentially with the number of elements, N, as it does in the coin example where W = 2N; instead, it might, say, follow a power of N such as W = N2.
This is a problem for the Boltzmann–Gibbs expression of entropy, says Tsallis, because mathematically N can no longer be taken outside the logarithm as a multiplier. The formula is now written as S = kB ln N2, which simplifies to S = 2kB ln N. In other words, entropy is no longer proportional to N; it is forced to be non-extensive. “If you keep using Boltzmann–Gibbs entropy, you are going to violate extensivity,” says Tsallis. “And I don’t want that.”
A cloudy idea
None of this was clear to Tsallis back in 1985. At that time he was at a meeting in Mexico City about statistical mechanics, when the study of fractals was becoming fashionable. Fractals are shapes that can be broken down into parts, each of which retains the statistical character of the whole, and are found throughout nature in, for example, lightning bolts, clouds, coastlines and snowflakes. Look closely at one of the arms of a snowflake, for instance, and it is possible to discern features that resemble the snowflake’s overall shape.
A mathematical generalization of a fractal is a “multifractal”, which describes such hierarchical structures using probabilities raised to a power, q (that is, pq). Tsallis describes how, during a coffee break at the meeting in Mexico City, he stayed behind in a room where another professor was explaining this concept to a student. “I couldn’t hear them,” he recalls, “but I knew they were talking about multifractals because of their writing on the blackboard – probability to the power q. And suddenly it came to my mind that that could be used to generalize Boltzmann–Gibbs entropy.”
Tsallis believes he instantly thought of entropy because the famous Boltzmann–Gibbs formula was always somewhere in his mind, “as it is for every statistical mechanist in the world”. But having written down a new formula, he did not know what, if anything, he had discovered. For two years he mulled over its implications, until a workshop in Maceió, Brazil, where he discussed it with two physicist colleagues, Evaldo Curado of CBPF and Hans Herrmann, who is now at ETH Zurich in Switzerland. “They were very stimulating, both of them,” Tsallis says.
From the discussion with Curado and Herrmann as well as with others around that time, Tsallis realized that his expression for entropy could be used to preserve the property’s extensive nature in cases when the Boltzmann–Gibbs formula makes it non-extensive – that is, in systems with strong correlations. Leaving Maceió, on a plane back to Rio, he performed calculations to convince himself that his formula worked, and then looked upon it with admiration. “I found it very cute, very pretty,” he recalls.
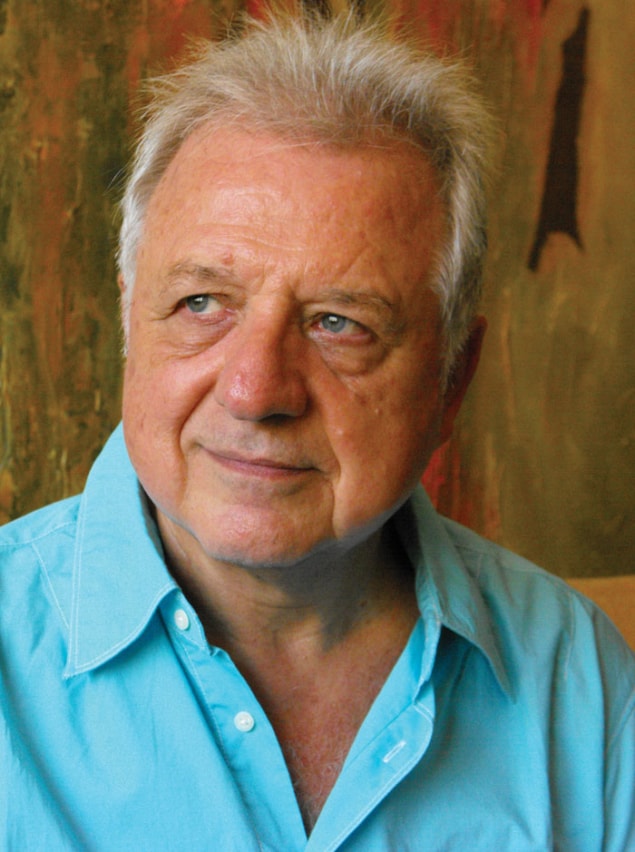
The new expression, called by him non-additive entropy and by others Tsallis entropy, derives its merit from the exponent, q, of the probability (see “Tsallis entropy defined” below). When the correlations in a system are weak or non-existent, q tends to one and the expression reduces to the standard Boltzmann–Gibbs formula. However, when the correlations in a system are strong, q becomes more or less than one to “bias” the probabilities of certain microstates occurring. The parameter q, which is now called the Tsallis index by proponents of the theory, is therefore a way of characterizing a system’s correlations – particularly how strong they are.
Three years after his formulation of non-additive entropy, in 1988, Tsallis published his Journal of Statistical Physics paper on the topic. For five years, few scientists outside Brazil were aware of it, but then its popularity skyrocketed – possibly due to research showing how non-additive entropy could be used in astrophysics to describe the distribution functions of self-gravitating gaseous-sphere models, known as stellar polytropes. Since then it has been used to describe, for example, fluctuations of the magnetic field in the solar wind, cold atoms in optical lattices, and particle debris generated at both the Large Hadron Collider at CERN in Switzerland and at the Relativistic Heavy Ion Collider at the Brookhaven National Laboratory in the US. In these cases, unlike Boltzmann–Gibbs entropy, Tsallis entropy is claimed to describe much more accurately the distribution of elements in the microstates; in the case of the LHC, these elements are the momenta of hadrons. More recently, Tsallis entropy has been the basis for a swathe of medical physics applications.
Defenders and detractors
Many people – notably the US physicist Murray Gell-Mann, who won the 1969 Nobel Prize for Physics for his theoretical work on elementary particles – agree that Tsallis entropy is a true generalization of Boltzmann–Gibbs entropy. But there are many detractors too, among whom the principal charge is that the Tsallis index q is a mere “fitting parameter” for systems that are not well enough understood.
Naturally, Tsallis disagrees. If the fitting-parameter accusation were true, he says, it would not be possible to obtain q from first principles – as he did in 2008, together with quantum physicist Filippo Caruso, who was then at the Scuola Normale Superiore di Pisa in Italy. Tsallis and Caruso showed that q could be calculated from first principles for part of a long, 1D chain of particle spins in a transverse magnetic field at absolute zero. The value of q, which was not equal to one, reflected the fact that quantum effects forced some of the spins to form strong correlations (Phys. Rev. E 78 021102).
This calculation required a knowledge of the exact microscopic dynamics, which is not, however, always possible. In situations where the dynamics are not known, says Tsallis, then q indeed has to be obtained from fitting experimental data, but he claims that doing so is no different to how other accepted theories are employed in practice.
As an example, Tsallis cites the orbit of Mars, which could be calculated from first principles – but only if both the distribution of all the other planets at a given moment, and the initial conditions of masses and velocities, were all known. Clearly, he says, that is impossible. “For the specific orbit, astronomers collect a lot of data with their telescopes, and then fit that data with the elliptic form that comes out of Newton’s law [of gravitation], and then you have the specific orbit of Mars,” he adds. “Well, here, it’s totally analogous. In principle, we would always like to be able to calculate q purely from mechanics, but it’s very hard, so q often has to be obtained from fitting.”
Mathematical physicist Henrik Jensen at Imperial College London takes a more nuanced view. He says that, for many years, proponents of Tsallis statistics did in fact make their case by calling attention to its greater ability to fit to data. But this, he says, is no longer true. “In the last couple of years work…has demonstrated that one might arrive at Tsallis statistics from very general assumptions about how complex correlated systems behave,” he adds.
That the Tsallis index is merely a fitting parameter is not the only criticism, however. In 2003 physicist Michael Nauenberg at the University of California, Santa Cruz claimed that Tsallis statistics is, for various technical reasons, incompatible with the zeroth law of thermodynamics, which states that two systems at different temperatures placed in thermal contact will reach thermal equilibrium at some intermediary temperature (Phys. Rev. E 67 036114). “Boltzmann–Gibbs statistics leads to this law, but Tsallis statistics violates it,” says Nauenberg. Why that should be the case is a rather technical argument, but he claims that if a thermometer were made from a substance whose entropy could only be described with Tsallis statistics, it would not be able to measure the temperature of ordinary matter.
“Tsallis statistics is a purely ad hoc generalization of Boltzmann–Gibbs statistics,” Nauenberg continues. “But since the appearance of Tsallis’s paper, applications of the new statistics have been made, without any justification whatsoever, to virtually every system under the Sun. As a fitting technique it may have some merits, but it is not a valid generalization of Boltzmann–Gibbs statistics.”
Eugene Stanley, a statistical and econophysicist at Boston University in the US, believes Nauenberg’s criticism is misplaced. He says that the zeroth law of thermodynamics is an “important and quite subtle” point that is still being explored for systems with strong correlations. “I suspect that many people don’t have a clear idea about a very deep question such as the extended validity of the zeroth principle of thermodynamics. Up to now, everything seems consistent with the possibility that the zeroth principle also holds for [Tsallis] systems, which violate Boltzmann–Gibbs statistical mechanics.”
Certainly, not everyone is convinced by the new theory of entropy, and the debates look set to continue. But on the wall of his office, Tsallis has posters of both Einstein and Boltzmann – perhaps in the subconscious hope that he will one day be known for overturning conventional statistical mechanics, as Einstein’s special theory of relativity overturned classical mechanics.
“Any physicist is supposed to know that classical mechanics works only when the masses are not too small and not too fast,” says Tsallis. “If they’re very small, you have to use quantum mechanics, and if they’re very fast, you have to use relativity.” But with statistical physics being one of the pillars of contemporary physics – and an obligatory subject in physics degree courses all over the world – he feels that students should be taught its limitations. “They should learn where Boltzmann–Gibbs statistics works, and where it doesn’t.”
If Tsallis’s ideas hold sway, that equation on Boltzmann’s gravestone may soon need updating.
Tsallis entropy defined
Standard Boltzmann entropy, where the probabilities of all microstates are equal, is given by the classic equation S = kB lnW, where S is entropy, kB is the Boltzmann constant and W is the total number of microstates in the system.
If the system has lots of different microstates, i, each with its own probability pi of occurring, this equation can be written as the Boltzmann–Gibbs entropy S = –kB pi lnpi.
Tsallis entropy, Sq, is claimed to be useful in cases where there are strong correlations between the different microstates in a system. It is defined as
where q is a measure of how strong the correlations are. The value of q is either more or less than one in such systems – effectively to bias the probabilities of certain microstates occurring – but in the limit where q approaches 1, Tsallis entropy reduces to the usual Boltzmann–Gibbs entropy. The parameter q is called the Tsallis index by proponents of the theory.
Medical applications of Tsallis entropy
In recent years, one of the most active fields in which Tsallis statistics has been applied is medical physics. In 2010, for instance, medical physicist Luiz Murta-Junior and colleagues at the University of São Paulo in Brazil applied Tsallis statistics to magnetic resonance imaging (MRI), to help them to delineate different types of tissue in the brain. A loss in the brain’s grey matter, for example, can be the cause of neurodegenerative diseases such as multiple sclerosis, which is why doctors turn to MRI to see how much grey matter there is relative to other tissues.
In any MRI scan, different tissues appear as different shades of grey, but each of these shades is actually made up from pixels with a range of different luminosities. The trick therefore is to work out the top and bottom thresholds in luminosity for each tissue – for instance, grey matter may contain pixels with luminosities between 20 and 90 on an eight-bit scale. This range corresponds to a certain value of entropy, since the greater the spread of luminosity values the greater the “disorder”. If there are just two different tissues in an MRI scan – grey matter and white matter – a scientist can analyse the image to determine the distribution of each tissue using an algorithm that adjusts two entropy variables until their total is a maximum.
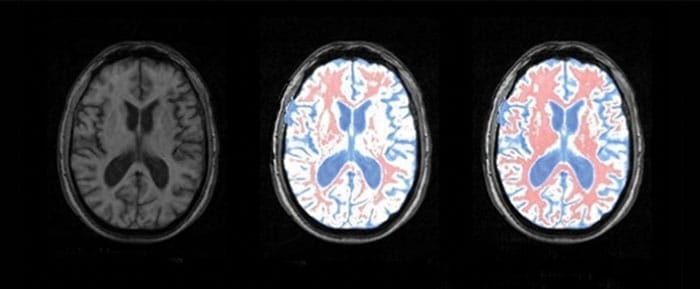
An algorithm based on Boltzmann–Gibbs entropy, and typical extensions of it, can do this. But according to Murta-Junior and colleagues, Boltzmann–Gibbs entropy does not allow for long-range correlations between pixels, which can arise in regions with complex, fractal-like shapes. The São Paulo researchers therefore turned to Tsallis entropy, and found that it could delineate grey matter from white matter and cerebrospinal fluid much more precisely (Braz. J. Med. Biol. Res. 43 77). “By accurately segmenting tissues in the brain, neurologists can diagnose the loss of grey matter earlier, and patients can be treated sooner with much better results,” says Murta-Junior.
In the same year as the São Paulo group’s research, electrical engineers at the Indian Institute of Technology Kanpur used Tsallis statistics to improve the detection in mammograms of mineral deposits known as microcalcifications, which are sometimes a sign of breast cancer. And in 2012 computer scientists at the Changchun University of Science and Technology in China again used Tsallis entropy with MRI, this time as an aid for image-guided surgery. This suggests that the debates about the fundamental validity of Tsallis statistics are scarcely deterring those wishing to make use of it.