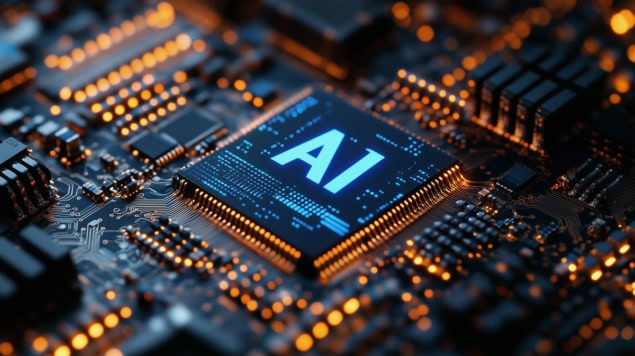
The rising popularity of generative artificial intelligence (GAI), and in particular large language models such as ChatGPT, could produce a significant surge in electronic waste, according to new analyses by researchers in Israel and China. Without mitigation measures, the researchers warn that this stream of e-waste could reach 2.5 million tons (2.2 billion kg) annually by 2030, and potentially even more.
“Geopolitical factors, such as restrictions on semiconductor imports, and the trend for rapid server turnover for operational cost saving, could further exacerbate e-waste generation,” says study team member Asaf Tzachor, who studies existential risks at Reichman University in Herzliya, Israel.
GAI or Gen AI is a form of artificial intelligence that creates new content, such as text, images, music, or videos using patterns it has learned from existing data. Some of the principles that make this pattern-based learning possible were developed by the physicist John Hopfield, who shared the 2024 Nobel Prize for Physics with computer scientist and AI pioneer Geoffrey Hinton. Perhaps the best-known example of Gen AI is ChatGPT (the “GPT” stands for “generative pre-trained transformer”), which is an example of a Large Language Model (LLM).
While the potential benefits of LLMs are significant, they come at a price. Notably, they require so much energy to train and operate that some major players in the field, including Google and ChatGPT developer OpenAI, are exploring the possibility of building new nuclear reactors for this purpose.
Quantifying and evaluating Gen AI’s e-waste problem
Energy use is not the only environmental challenge associated with Gen AI, however. The amount of e-waste it produces – including printed circuit boards and batteries that can contain toxic materials such as lead and chromium – is also a potential issue. “While the benefits of AI are well-documented, the sustainability aspects, and particularly e-waste generation, have been largely overlooked,” Tzachor says.
Tzachor and his colleagues decided to address what they describe as a “significant knowledge gap” regarding how GAI contributes to e-waste. Led by sustainability scientist Peng Wang at the Institute of Urban Environment, Chinese Academy of Sciences, they developed a computational power-drive, material flow analysis (CP-MFA) framework to quantify and evaluate the e-waste it produces. This involved modelling the computational resources required for training and deploying LLMs, explains Tzachor, and translating these resources into material flows and e-waste projections.
“We considered various future scenarios of GAI development, ranging from the most aggressive to the most conservative growth,” he tells Physics World. “We also incorporated factors such as geopolitical restrictions and server lifecycle turnover.”
Using this CP-MFA framework, the researchers estimate that the total amount of Gen AI-related e-waste produced between 2023 and 2030 could reach the level of 5 million tons in a “worst-case” scenario where AI finds the most widespread applications.
A range of mitigation measures
That worst-case scenario is far from inevitable, however. Writing in Nature Computational Science, the researchers also modelled the effectiveness of different e-waste management strategies. Among the strategies they studied were increasing the lifespan of existing computing infrastructures through regular maintenance and upgrades; reusing or remanufacturing key components; and improving recycling processes to recover valuable materials in a so-called “circular economy”.
Taken together, these strategies could reduce e-waste generation by up to 86%, according to the team’s calculations. Investing in more energy-efficient technologies and optimizing AI algorithms could also significantly reduce the computational demands of LLMs, Tzachor adds, and would reduce the need to update hardware so frequently.
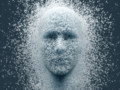
Fighting algorithmic bias in artificial intelligence
Another mitigation strategy would be to design AI infrastructure in a way that uses modular components, which Tzachor says are easier to upgrade and recycle. “Encouraging policies that promote sustainable manufacturing practices, responsible e-waste disposal and extended producer responsibility programmes can also play a key role in reducing e-waste,” he explains.
As well as helping policymakers create regulations that support sustainable AI development and effective e-waste management, the study should also encourage AI developers and hardware manufacturers to adopt circular economy principles, says Tzachor. “On the academic side, it could serve as a foundation for future research aimed at exploring the environmental impacts of AI applications other than LLMs and developing more comprehensive sustainability frameworks in general.”