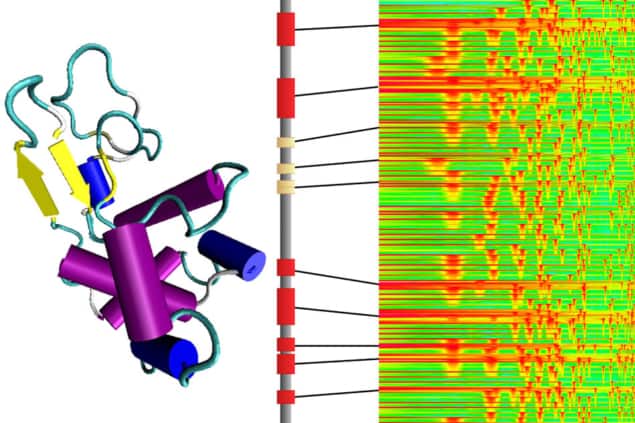
Ever wondered what Beethoven and bone have in common? According to researchers at Massachusetts Institute of Technology (MIT) in the US they both gain from being composed of structures over a range of scales be that notes, chords and melodies or amino acids, proteins and collagen matrices. Taking the analogy further Markus Buehler and colleagues have translated the vibrations of amino acids and the longer-range structures of the proteins they form into a musical framework. Machine learning algorithms trained on this musically transcribed protein data could devise fresh amino acid music based on musical principals learnt from the training data set, which the researchers then translate back into protein structures.
“What we’ve been trying to do in a lot of different ways is to find ways of predicting a protein’s functionality from its sequence and that’s a really difficult thing to do,” Buehler tells Physics World. For several years Markus Buehler and his group at MIT have studied materials including spiders’ webs and nacre to identify the hierarchical structures behind their impressive mechanical properties. He explains that current approaches to relating protein structure and function generally rely on long computational-resource-hungry molecular dynamics simulations to solve equations approximating the quantum mechanical interactions at the molecular scale in order to determine how the protein folds, and how it functions. “One of the directions we have pursued is to think how we look at materials, and we realized that when we look at materials at the molecular scale, the atoms and molecules continuously vibrate. So we thought that maybe there’s a way of capturing the spectrum of vibrations at the nanoscale and building a model from that.”
Based on the vibrational frequencies of amino acids the researchers developed an amino acid musical scale. They then encoded secondary structures that govern the way the proteins fold into other musical components such as rhythm and volume. Just as pictorial representations of data can make it easier to recognize patterns and trends, presenting the algorithm with a musical representation of the data may help identify relationships that have so far remained elusive, and already the results are promising.
“Based on a training [data] set, we can now come up with proteins that nature has not invented before,” says Buehler. “We’ve also found that some of the proteins that our AI method can generate are proteins that nature has invented, but they were not part of the training sets – it’s really fascinating that the method can sort of discover things that evolution has already discovered on its own. And this leads to the application of this work, that we’re now able to optimize protein sequences using this method because we can ask the AI to generate a large number of candidates, which we can then further categorize.”
Musical inspiration
Training a machine learning algorithm on an amino acid orchestra to discover new useful materials may seem a neat if unorthodox idea, but successfully putting it into practice has built on over a decade of work exploring the function and properties of hierarchical structures and the translation of theoretical models into different frameworks. As well as this unique catalogue of expertise in Buehler’s group, developments in AI have been crucial to making use of the approach.
Unlike classical western music, where each scale is made up of 12 notes separated by semitones, the amino acid scale has 20 tones, and sounds very different. Using the musical representation of proteins to reveal insights into the relationships between protein structures at different levels and their functions, requires not just a familiarization with this strange sounding music but the ability to ignore the principals of classical music.

Art and science light up
“AI was a way of overcoming that limitation, to have a neutral model that has never heard any music but basically learned only from the data that was provided,” says Buehler. “Because one of the problems you have with a human brain, is that if you want to compose a new protein you tend to want to make it sound like the music you’ve heard before – this is sort of how composition works we’re trying to create or explore ideas, themes, chord progressions and so on that you have maybe heard before. Of course, in this case it doesn’t work, because these core progressions and melodies and successions of notes, might not at all relate to anything that’s important for protein.” While the system has already identified real and potentially realisable proteins, future work will require improved data sets to train the algorithms with the aim of identifying protein structures with specific folding and functional properties.
Unifying trends
Buehler is also optimistic about other sectors that could benefit from a musical representation of data. “Generally, I think there’s a very interesting fundamental insight that one can possibly get from this kind of work. An extension of this work would be the idea that many of these hierarchical systems actually show striking similarities in the way they’re built.” He describes the way materials like spider silk, bone, and nacre, or language or music share similarities in the way they are constructed to give rise to various functions from simple building blocks. “One of the general things I think that we could explore is whether we have discovered certain features or ways systems can be designed better in other representations.”
You can hear compositions from these musical representations on soundcloud or download an app to play with them yourself. Full details of the work applied to protein research are reported in ACS Nano.
- This article was edited Thursday 4th July to include the audio clip