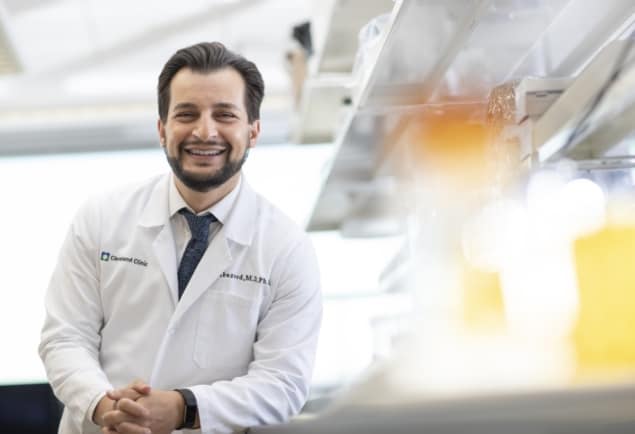
Radiotherapy is currently delivered using generic dose prescriptions that don’t account for individual tumour characteristics. To help personalize treatments, researchers from Cleveland Clinic and Siemens Healthineers have developed an artificial intelligence (AI) framework that uses a patient’s CT scans and electronic health records to generate an individualized radiation dose (Lancet Digital Health 10.1016/S2589-7500(19)30058-5).
The team built the AI framework using CT scans and health records from 944 lung cancer patients treated with stereotactic body radiotherapy. This high-dose treatment was introduced to achieve local tumour control while avoiding surgical morbidity in patients with early-stage lung cancer or lung metastases. But recent studies have noted high local failure rates in some patient subgroups. Personalized adjustment of radiotherapy dose could help mitigate such failures.
“While highly effective in many clinical settings, radiotherapy can greatly benefit from dose optimization capabilities,” explains lead author Mohamed Abazeed. “This framework will help physicians develop data-driven, personalized dosage schedules that can maximize the likelihood of treatment success and mitigate radiation side effects for patients.”
Deep learning framework
For their latest study, Abazeed and colleagues input pre-treatment lung CT images into Deep Profiler, a deep neural network that has radiomics incorporated into its training process. Deep Profiler analysed the scans, examining a multitude of deformable radiomics features, to create an image signature that predicts treatment outcomes.
The researchers used 849 patients in an internal study cohort (those treated at Cleveland Clinic main campus) to train and validate the AI framework. They found that radiotherapy failed at a significantly higher rate in patients with high image scores than in those with low scores; the three-year cumulative incidence of local failure was 20.3% in high-risk patients compared with 5.7% in the low-risk group.
The team validated the accuracy of their framework using an independent cohort of 95 patients who had received stereotactic lung radiotherapy at seven affiliate sites and were scanned using several types of CT scanner. The network was accurate in stratifying patients in this external cohort into high- and low-risk groups on the basis of data extracted from the internal study population; the two-year cumulative incidences of local failure were 9.5% and 39% in the low- and high-risk groups, respectively.
These results indicated the presence of image-distinct patient subpopulations with differential sensitivity to radiotherapy.
Individualizing the dose
Next, the researchers combined the image signatures from Deep Profiler with clinical variables from patient health records – such as biologically effective dose and histological subtype (adenocarcinoma and squamous cell carcinoma) – to derive iGray, a personalized radiation dose that minimizes the risk of local failure. They defined iGray as the patient-specific dose that reduces the probability of treatment failure to below 5% at 24 months.
The researchers note that iGray uses the clinically validated linear quadratic model, is empirically derived, with no assumptions made regarding individual tumour radiosensitivity, and is directly clinically actionable as it recommends a dose that can be achieved using several treatment schedules. In this study, iGray ranged from 21.1 to 277 Gy – a wider dose range than the actual delivered dose (39–180 Gy) – and recommended a dose reduction in 23.3% of patients. The results also showed that iGray can be safely delivered in the majority of cases.
“The development and validation of this image-based, deep-learning framework is exciting because not only is it the first to use medical images to inform radiation dose prescriptions, but it also has the potential to directly impact patient care,” says Abazeed. “This image-based information platform can provide the ability to individualize multiple cancer therapies but more immediately is a leap forward in radiation precision medicine.”