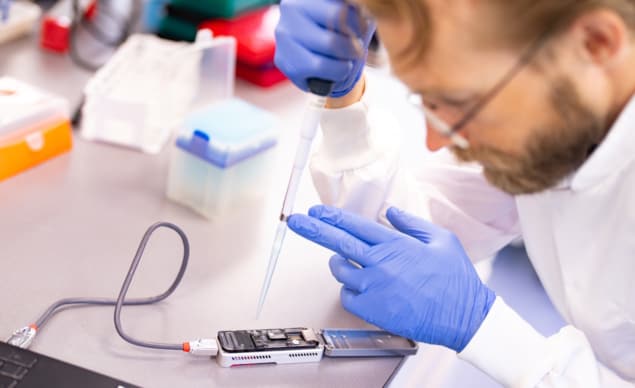
For a neurosurgeon, removal of cancerous tissue in the brain is a fine balancing act between maximizing the amount of tumour removed to extend a patient’s survival and minimizing the risk of permanent neurological damage. A new tool that combines rapid DNA sequencing and artificial intelligence to classify central nervous system (CNS) tumours during brain cancer surgery is enabling neurosurgeons to make better decisions about the extent of tumour resection that will most benefit the patient.
Surgeons have limited knowledge of the tumour type prior to surgery. As surgery begins, sections of tumour tissue are removed for immediate histological assessment. But the DNA sequencing for histological and molecular analysis by a pathologist typically requires a week to provide a definitive diagnosis.
By comparison, the new tool – named Sturgeon by its multi-institutional team of developers in the Netherlands – can make an accurate diagnosis within 90 min for most CNS tumours. And once they know the tumour type and aggressiveness, neurosurgeons can modify their surgical strategy in the operating room as the tumour classification warrants.
“During surgery, a small remnant of tumour tissue is sometimes deliberately left behind to prevent neurological damage,” explains paediatric neurosurgeon Eelco Hoving in a press statement. “But if it later turns out, for example, that the tumour is very aggressive, a second surgery may still be necessary to remove that last remnant. This can be avoided now because we will already know during the first surgery what type of tumour we are dealing with.”
Reporting their findings in Nature, the researchers – from UMC Utrecht, Amsterdam UMC and the Princess Máxima Center for Pediatric Oncology – explain how they created, trained and tested the tool. They also describe its use during 25 surgeries, where Sturgeon accurately classified 72% of tumours in less than 45 min.
Sturgeon works by using rapid nanopore sequencing, a technology that helps to read DNA in real time, to obtain a sparse methylation profile during surgery. Methylation patterns are DNA modifications that are highly distinctive of an individual tumour type, enabling molecular subclassification of CNS tumours. The neural network classifier is patient agnostic, which means that it does not require patient-specific model training, and takes just a few seconds to run on a laptop computer.
Due to the limited availability of nanopore-based methylation datasets, Bastiaan Tops, Jeroen de Ridder and colleagues developed a strategy to generate realistic training data from standard array-based methylation profiles. Sturgeon uses these data to upsample the number of training samples available, simulating thousands of unique nanopore sequencing experiments from each tumour methylation profile. Ultimately, the final Sturgeon models were trained on 36.8 million simulated nanopore runs and validated on an addition 4.2 million.
The researchers initially trained Sturgeon to perform CNS tumour classification and applied them to sparse nanopore sequencing data in 50 CNS tumour samples and a publicly available data set of sequenced CNS samples. The model correctly classified 45 out of the 50 tumour samples, within 40 min of starting sequencing, with similar results for the public data set.
To specifically validate Sturgeon’s performance in diagnosing paediatric CNS tumours, the team obtained 94 methylation profiles from paediatric patients who had a CNS tumour resection and used these to simulate nanopore sequencing experiments. For cases with a clear diagnosis, Sturgeon correctly classified (at a 0.8 confidence threshold) 95.3% of 34,000 simulated samples within 25 min, and 97.1% within 50 min.
“These results suggest that a conclusive diagnosis can be reached within 25–50 min of simulated sequencing for the vast majority of paediatric cases that can be classified…with a very low error rate,” they write.
The team also demonstrated the use of Sturgeon during 20 paediatric surgeries at the Princess Máxima Center and five adult surgeries at Amsterdam UMC. For this clinical feasibility study, samples obtained for histological assessment were split, with one part used for intraoperative sequencing and the other for histological assessment. The researchers report that Sturgeon correctly diagnosed 18 out of the 25 tumours in less than 45 min of sequencing, with a total diagnostic turnaround time of less than 90 min.

Multispectral infrared imaging improves guidance of cancer surgery
One limitation of Sturgeon is that only performs well in samples that are sufficiently represented in training data, which do not include rare types of CNS tumour. Sturgeon also does not perform as well when analysing samples containing less than 50% of abnormal cells. Additionally, large tissue samples (about 5 mm3), are needed to provide sufficient DNA concentration.
The researchers tell Physics World that further future developments of this method will include application to other tumour types, such as sarcoma or leukaemia, as well as prospective validation to demonstrate patient benefit and studies in a much larger patient population.