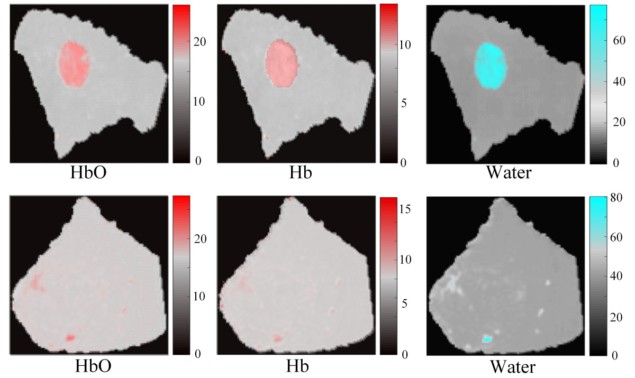
An international research team has configured the deep-learning-based Z-net algorithm to generate MRI-guided near-infrared spectral tomography (NIRST) images directly from measured optical signals and MRI data. According to its developers, from Dartmouth College, Beijing University of Technology and the University of Birmingham, the new algorithm shows potential to improve the detection and diagnosis of breast cancer. Reporting their findings in Optica, they note that the algorithm’s ability to learn from data in near-real time overcomes a major hurdle in multimodality imaging. Z-Net also can distinguish between malignant and benign tumours using data from non-contrast MRI-guided NIRST breast exams.
NIRST is a diagnostic imaging technique that characterizes soft tissue optical properties in the spectral range of 600–1000 nm for early detection of cancer. The complexity and time required to reconstruct diagnostic quality NIRST images, however, has made the technology impractical for day-to-day clinical use.
Incorporating structural information from simultaneous MR imaging can significantly improve the obtained tissue function images. The researchers explain that the process of MRI guidance in NIRST has been time consuming, however, due to the need for tissue-type segmentation and forward diffuse modelling of light propagation in tissue. As such, they propose using an artificial intelligence algorithm to perform near-real-time image reconstruction of MRI-guided NIRST, without the need for complex light propagation modelling.
To create the Z-Net-based algorithm, the researchers used diffuse optical signals and MR images as inputs to the neural network, and simultaneously recovered the concentrations of oxy-haemoglobin, deoxy-haemoglobin and water from 20,000 sets of computer-generated simulations. After training the algorithm, they confirmed that eliminating diffuse light propagation modelling and MRI segmentation did not degrade the quality of the resulting images.

To test the algorithm’s clinical relevance, the researchers applied Z-Net to patient data obtained by their MRI-guided NIRST system. Principal investigator Keith Paulsen, of Dartmouth’s Thayer School of Engineering, explains that the MRI exam and NIRST data acquisition were carried out simultaneously for two women with suspicious undiagnosed abnormalities at the time of their imaging exams. One patient was later diagnosed with invasive ductal carcinoma and the other with a benign fibroadenoma. Z-Net was able to characterize and distinguish malignant from benign tissue, using only tissue haemoglobin concentration and water images.
“Since Z-Net can be expanded by adding other parameters, such as oxygen saturation, lipids and scattering properties into the network, the diagnostic power for breast cancer detection may be increased even further as multispectral systems for tissue spectroscopy are advanced,” the team writes.
Lead author Jinchao Feng, of the Beijing Key Laboratory of Computational Intelligence and Intelligent System, points out that the Z-Net algorithm reduces the time needed to generate a new image to several seconds. “Another advantage is that the algorithm can be trained with data generated by computer simulations rather than from actual patient exams, which can expedite training when in vivo data is insufficient or unavailable,” Feng explains. “These capabilities will allow Z-Net’s adaptation for use with other cancers and diseases for which multimodality imaging data are available.”
“Although Z-Net training sets were based on 3D simulations, data from a single MRI slice was input to the network, and thus, the network output was a 2D image of chromophore concentrations,” Paulsen tells Physics World.
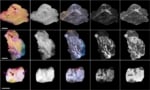
Optical imaging could reduce recall surgery for breast cancer patients
The researchers are developing a new NIR/NIRST imaging system with a breast interface that has many more sources and detectors that cover the entire breast volume. They are also working on an improved Z-Net algorithm that receives the 3D MR image volume (many slices of the MR image) and returns the 3D NIRST images.
“We hope that 3D NIRST images of chromophore concentrations can be learned by Z-Net and recovered from patient data to improve MRI diagnostic accuracy and performance,” Paulsen adds. The team is now planning a clinical study, which will involve about 40 women who have breast abnormalities, in the near future.