
Whole-body positron emission tomography/computed tomography (PET/CT) is a diagnostic imaging technique that can detect the spread of cancer or monitor a tumour’s response to treatment. But manual delineation of the multiple lesions often observed in whole-body images is a time-consuming task that’s subject to inter-reader variability. For clinical use, what’s really needed is an efficient, fully automated method for detection and characterization of cancer from PET/CT images, enabling rapid diagnosis and treatment.
Deep learning can be used to automatically extract key features from image data, but the models need to be trained on large annotated datasets, which may not be readily available. To remove this reliance on labelled data, a team headed up by Kevin Leung from Johns Hopkins University School of Medicine is using deep transfer learning – a technique that employs knowledge from a pre-existing model to address a new task – to detect six different types of cancer, imaged with two different radiotracers, on whole-body PET/CT scans.
“Deep learning models are also often developed for specific radiotracers,” explained Leung, who presented the findings at this week’s 2024 SNMMI Annual Meeting in Toronto. “And given the wide range of radiotracers available for nuclear medicine, there’s a need to develop generalizable approaches for automated PET/CT tumour quantification in order to optimize early detection and treatment.”
The new approach uses deep transfer learning to jointly optimize a 3D nnU-Net backbone (a deep learning-based segmentation method) across two PET/CT datasets, in order to learn to generalize the tumour segmentation task. The model then automatically extracts radiomic features and quantitative imaging measures from the predicted segmentations, and uses these extracted features to assess patient risk, estimate survival and predict treatment response.
“In addition to performing cancer prognosis, the approach provides a framework that will help improve patient outcomes and survival by identifying robust predictive biomarkers, characterizing tumour subtypes, and enabling the early detection and treatment of cancer,” says Leung in a press statement.
For their study, Leung and colleagues examined data from a total of 1019 patients. For training and cross validation, they used PET scans (with limited tumour annotation) from 270 patients with prostate cancer imaged with the PSMA-based tracer 18F-DCFPyL, as well as scans (with complete annotations) from 501 patients with lung cancer, melanoma or lymphoma imaged with the metabolic tracer 18F-FDG.
For external testing, they used PSMA PET scans of 138 patients with prostate cancer and FDG PET scans from 74 patients with head-and-neck cancer and 36 with breast cancer (none containing annotations). The automated segmentation approach yielded median true positive rates ranging from 0.75 to 0.87, and median Dice similarity coefficients ranging from 0.73 to 0.83, indicating fairly accurate segmentation performance.
Leung shared the findings of the three prognostic models that the researchers developed. For risk stratification of prostate cancer, they used whole-body imaging measures extracted from the tumour predictions to build a model that predict patients as low to high risk. Comparison with classifications based on initial prostate-specific antigen (PSA) levels showed that the risk model had an overall accuracy of 0.83.
“Patients predicted as being high risk also had significantly higher follow-up PSA levels and shorter PSA doubling times compared to low- and intermediate-risk patients, indicating further disease progression for those patients,” Leung explained.
In a similar manner, the researchers used imaging measures from FDG PET scans of patients with head-and-neck cancer to calculate risk scores. They found that the risk score was significantly associated with overall survival, with patients assigned a higher risk score having the shortest median overall survival.
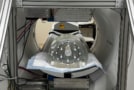
Innovative devices ramp the resolution of PET imaging
Lastly, the team created models to predict the response of patients with breast cancer to neoadjuvant chemotherapy, using imaging measures extracted from pre- and post-therapy FDG PET scans. A classifier using only pre-therapy imaging measures predicted pathological complete response with an accuracy of 0.72, while models using both pre- and post-therapy measures exhibited an accuracy of 0.84, highlighting the feasibility of using the model for response prediction.
“The approach may be able to help reduce physician workload by providing automated whole-body tumour segmentations, as well as automatically quantifying prognostic biomarkers,” Leung concluded, noting that the AI tool could also play a role in tracking changes in tumour volume in response to therapy.