Chris Lucas is a research engineer in the AI research team at Babylon Health. His work covers the research and application of a wide range of machine-learning techniques
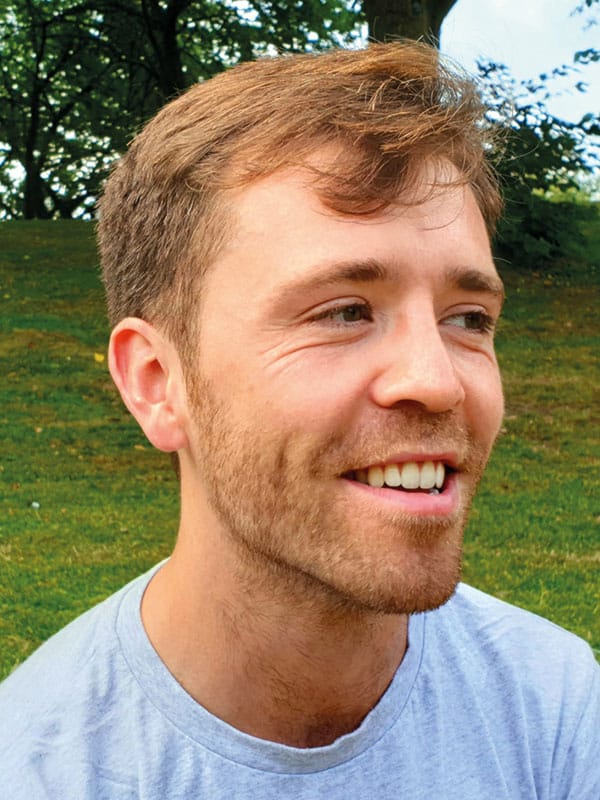
What sparked your initial interest in physics?
I suppose it started at school. I remember the older kids warning us how difficult and boring physics classes would be at secondary school, so I was massively relieved to find the complete opposite was true. We were very lucky to have an inspirational teacher who was passionate about the subject and really pushed to share his enthusiasm. Lively experiments and demos were common. One in particular sticks in my memory: building a 5 m long capacitor from a roll of cling-film sandwiched between two rolls of tin foil, both attached to a high-voltage power supply. I remember our teacher casually informing us at the last minute, “Oh yes, don’t touch it after I’ve turned it on…you’ll definitely die.” How could I not be excited?
After years of being encouraged to believe you had to go to university to get a specific job, I’d decided that I should get a degree in engineering. But after multiple university open days, I couldn’t shake the feeling that engineering wasn’t for me. If I was going to do something for four years, then I decided it’d better be something I found interesting and exciting. The decision to study physics at Bristol is still to this day the best move of my life. If you’d told me all those years ago that it would lead to me working on the Large Hadron Collider (LHC), one of the most famous and complex physics experiments in history, I probably would have just laughed.
Did you ever consider a permanent academic career after your PhD in particle physics?
I really loved the work I did as a PhD student on the CMS experiment at the LHC, searching for elusive supersymmetric particles. Working as part of a worldwide collaboration, at the frontier of knowledge, was incredibly exciting, so naturally I considered continuing on the path through academia. The work I’d done at CERN on both data analysis and experimental hardware could have led me down a variety of paths, but I think for me the biggest draw was the prospect of teaching. I’ve always loved sharing my passions with others and could easily see myself as a physics lecturer. Unfortunately, in my field the path to lectureship looked very uncertain, with few roles ever becoming available. Facing this, and the prospect of the long shutdown at the LHC, I made the decision to see what life was like “on the other side”. I was very fortunate to have met and worked with people who had experience in the tech world. Following some lengthy, and occasionally even constructive pub chats, I decided to take the plunge and move to London in search of the table-tennis playing, silicon-roundabout dream.
How did your interest in data science and software engineering emerge?
Most of the work during my PhD involved writing code, largely for performing some fairly heavy-duty statistical analyses on huge amounts of data. I’d done lots of maths and statistics courses throughout my undergrad and postgrad, but only ever basic programming. We basically learnt to code on the job, and so I never quite felt I was doing things “properly”. I felt I had a fairly good grasp of the statistical side of things, and so instead decided to improve my engineering skills. My first role was as a junior software engineer at a small tech start-up where I learnt a lot very quickly, especially how to write reliable and efficient code. I loved my time there, but after a couple years in the role I realized I was missing the excitement and cutting-edge nature of scientific research, and so was lucky enough to be approached for my current position at Babylon Health. With Babylon I’m finally able to combine both my scientific and engineering backgrounds, all within a supportive, flexible and impactful research environment.

Once a physicist: Paul Bate
What does your current role as a research engineer in machine learning involve?
At Babylon Health I work in the research team. We’re very fortunate to be afforded quite a high level of autonomy, much like in academia. Within this research environment we develop machine-learning approaches to tackle the many challenges associated with digitally delivering healthcare. More specifically, my role encompasses everything from researching and implementing a wide range of machine-learning techniques, building research tools for our group and getting involved in the delivery of this code into the production systems our company currently offers.
I think the thing I love the most about my position is that I’m able to continually learn and grow in such an interesting and dynamic field. Over the last few months I’ve been studying and experimenting with many exciting new fields of research, such as adversarial learning where you train models by having them compete; creating models that can imagine and reason about counterfactual worlds; and developing statistical methods for safeguarding user privacy in a dataset. The cherry on the top being that when we attempt to use these techniques, we do so to positively impact people’s lives by improving the access, affordability and quality of healthcare for everyone.
How has your physics background been helpful in your work, if at all?
I definitely find my physics background a huge help in my day-to-day work. I suppose there are the obvious qualities like analytical thinking and being comfortable with maths, but ultimately the biggest benefit is the capacity for technical problem-solving. As physicists we’re basically trained to approach a complex problem, break it down into digestible components and apply whatever techniques are necessary to tackle them. It’s interesting to find how many ex-physicists there are now working in the machine-learning field. I keep spotting names I recognize at conferences, or finding an increasing number of machine-learning papers emerging with references to classical thermodynamics, gauge-equivariance or quantum mechanics. It’s really encouraging to see that so many physicists are able to take their knowledge and training to help advance and progress the field of machine learning, and ultimately artificial intelligence, in such a positive way.
Any advice for today’s students?
I’m probably echoing what a lot of people have said already, but my main advice would be not to stress if you’re unsure of what direction you want to take with your studies or beyond. A degree in physics is one of the most versatile and sought-after qualifications someone can bring to the table.