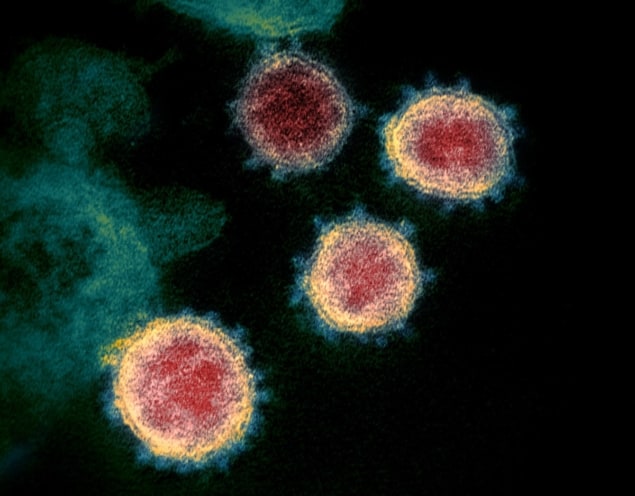
Models of disease spread inform governments on when and how to ease the measures currently in place to contain COVID-19. But physicist Susanna Manrubia, an expert in modelling biological phenomena at the Spanish National Centre for Biotechnology in Madrid, is alarmed by the precise predictions reported by some models. In response, she and her colleagues have highlighted the uncertainties in predicting the peak and end of the pandemic in a e-print currently under peer review.
“We are really worried about the limitations of modelling and thought media predictions were getting out of hand,” explained Manrubia.
The e-print is available on Cornell University’s arXiv server. In it, Manrubia and colleagues describe how they used a simple model to expose COVID-19 forecasting uncertainties, and demonstrate that uncertainty is intrinsic to a pandemic’s exponential growth pattern. They conclude that more realistic weather-like forecasts, transparent about uncertainties, should be used in portraying COVID-19 predictions to the public and governing authorities. “Only probabilistic prediction is feasible and reliable,” states Manrubia.
David Dowdy, a clinician who researches infectious disease epidemiology at Johns Hopkins University in the US, and Samir Bhatt, an expert in modelling infectious diseases from Imperial College London, were not involved in the study, but agree that presenting uncertainty is vital. “With COVID, I don’t feel like any model should be making projections more than a few weeks in advance,” says Dowdy. “There are too many unknown factors beyond that. And if you’re going to do it, you have to do it in a way that suggests the great uncertainty.”
Modelling disease spread
Many approaches used to estimate the future stages of infectious disease spread — and to quantify the impact of social distancing measures aimed at “flattening the curve” — are based on simple models. These models include limited mechanistic detail, simply simulating the exponential growth of an epidemic using a set of differential equations, with the population described as being in three distinct categories – susceptible, infectious or recovered (SIR). There are various iterations of these SIR models, incorporating different categories such as quarantined or infected but asymptomatic.
To highlight the limitations of such epidemic modelling, Manrubia and colleagues selected Spain’s COVID-19 epidemic as a case study and applied a variant of the SIR model called SCIR that includes a category describing the reversible confinement of susceptible individuals. The team used a Bayesian approach to probabilistically fit the data – training parameters (factors defining the outbreak such as infectivity) according to expected distributions. These priors were then filtered and fitted to the data.
The result was a posterior distribution of parameter values that accurately recovered the daily recorded data in Spain – in the period 28 February to 29 March.
Sensitive to small variations
“We could recover data of the past nicely but see a sensitivity to small variation in the parameters that causes a spread of trajectories,” says Manrubia. Many of these possibilities indicated a reduction in active COVID-19 cases – “flattening the curve” – but there was also bending towards continued exponential growth of Spain’s epidemic. “There, your prediction is somehow lost in the sense that, you can only say probabilistically speaking, whether there will be a peak in three days or not.”
Attempts to add precision into these models often involves including more categories, but Manrubia points out that this practice adds parameters, so multiplying potential for variation.
Bad data is often blamed for a prediction’s uncertainty, but Manrubia and colleagues argue that it’s not just about the data. They illustrate this by directly integrating the SCIR model to generate synthetic data, and then fitting the same model to this “perfect data set”. A spread in future trajectories was still observed, and the team explained this is due to intrinsic dynamics of model’s with exponential growth in the variables, such as an epidemic.
“Forecasting the future is very challenging. Even with perfect data, it doesn’t give information about who is going to get infected in the future,” says Bhatt. He points out that inherent unpredictability in predicting the future isn’t a new finding in itself, but acknowledges the value in highlighting uncertainties in the current crisis.
Dowdy is also in favour of this issue being broadcast to a wider audience. “[Uncertainty in forecasts] is an issue that is well-known in the scientific community, but not well-known in the lay community.”
Presenting the probabilities
Manrubia is frustrated by models that extrapolate from the single parameter value best fitting past data, so failing to show that many other predictions are possible. “We are trying to get more care from those doing models, and get the message up to the authorities because there is so much noise they need to be aware of.”

COVID-19: how physics is helping the fight against the pandemic
“It’s fine to be wrong (forecasting models are by definition going to be wrong because they are making assumptions), it’s the communication that is absolutely essential,” says Bhatt. “Some scientists need to be transparent about the limitations of models and what they tell us, and this paper nicely discusses that.”
Manrubia hopes this crisis will spur the global epidemiology community to reliably integrate data across the globe. “SIR models are really simple – they’ve been around for a century – come on. I’m certain that we can do better.”