Abi Ramanan describes how hyperspectral imaging and machine learning could change the future of food production, drastically reducing waste and increasing consumer satisfaction
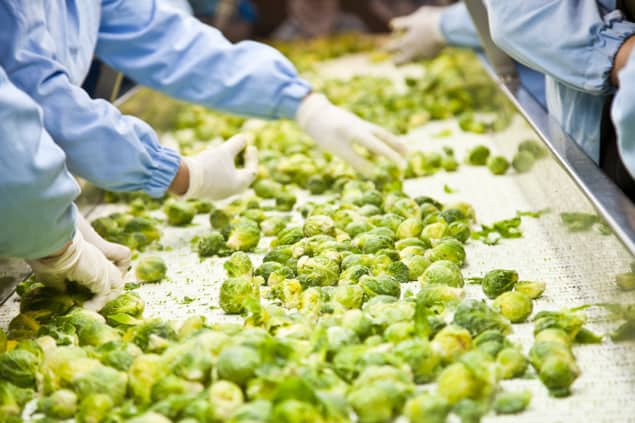
If wasted food were a country, it would be the world’s third-largest emitter of greenhouse gases. A staggering one-third of all food produced goes to waste, accounting for an estimated 8% of global emissions – more than any country except China and the US. As well as an environmental disaster, food waste is also bad for the economy, costing close to a trillion dollars annually.
There are many strategies for reducing food waste. They include better storage and transportation in countries with less advanced infrastructure, and a range of measures – including more consistent labelling; less rigorous cosmetic standards for food among retailers; and education campaigns aimed at consumers – in more developed regions. But there are also big savings to be made by reducing waste higher up in the supply chain, before the food reaches either consumers or retailers. In 2018, the Boston Consulting Group reported that supply-chain improvements, together with increased efficiency, could reduce loss and waste by $270bn.
To realize those benefits, food producers urgently need accurate, non-invasive tools that enable them to assess the quality of food in real time, in a way that integrates seamlessly with existing production-line systems. This is where a technology known as hyperspectral imaging comes in. In effect, this type of imaging combines spectroscopy with computer vision, making it possible to measure both spatial and spectral characteristics reliably and simultaneously. Each pixel in a hyperspectral image contains information about how a corresponding spatial pixel absorbs or reflects more than 100 wavelengths of light. The higher information per pixel ratio allows a larger volume of data to be obtained than is possible with traditional RGB cameras, or even with multispectral imaging, which typically records light at fewer than 10 wavelengths.
Hyperspectral imaging was originally developed for remote sensing applications, but as the price and size of sensors has decreased, it is now being deployed in industries as diverse as criminal forensics, pharmaceuticals and manuscript analysis. Although the food industry has been relatively slow to digitize, the time is now ripe (forgive the pun) for hyperspectral imaging to be rolled out across food-supply chains, too.
Finding things that don’t belong
In a food production context, hyperspectral imaging can provide valuable insights about food quality. For example, it enables us to classify the freshness of fish into discrete categories, because the fish’s chemical composition determines how much light it reflects at different wavelengths. This type of imaging can also yield better estimates of a product’s shelf life or ripeness, and information about whether there are any contaminants present. Previously, such information could typically only be acquired via a range of destructive tests (such as sample-based testing for pH, a shear force test, the laboratory oven method, the refractometer and many others) and by time-consuming, costly and imperfect visual inspections. Hyperspectral imaging could therefore transform the way food supply chains operate by facilitating a shift towards 100% product coverage, resulting in less waste, higher yields and increased revenues.
One of the food industry’s biggest challenges – and a key focus for ImpactVision, the company I co-founded – is keeping non-food objects out of production lines. Foreign-matter contamination is the third leading cause of recalls in both the US and the UK, after biological contamination and mislabelling. Such recalls can be prohibitively expensive: a 2011 study from the Food Marketing Institute and Grocery Manufacturers Association estimated that a single foreign-matter-related food recall can cost a major producer up to $10m. The number of recalls is also on the rise. Between 2015 to 2016, recalls related to foreign bodies jumped 60 percent in the US, and recalls in the EU also rose. In 2018 alone, US food companies were subject to 30 high-profile recalls, amounting to hundreds of thousands of pounds of wasted food.
Technologies for detecting foreign matter do exist, but they serve specific purposes. X-ray machines can detect dense objects, but they miss lighter ones such as certain plastics, paper, cardboard and insects. Magnets and metal detectors will not pick up many of the most common foreign-object culprits – plastics, paper, stones, textiles – at all. (They also miss some more emotive contaminants, such as the live frog that one Californian woman decided to keep as a pet after she found it in a bag of salad.) Whenever such objects get into the production line, stoppages in the line, lost batches and missed deliveries are the inevitable result – not to mention lower production yields and eroded customer confidence and brand credibility.
ImpactVision’s system overcomes these limitations by using hyperspectral imaging and machine learning to detect, identify and eliminate foreign objects. By gathering information about the spectral and spatial characteristics of foreign objects, we can discern differences in their spectral signatures, which vary considerably based on chemical composition. Our hyperspectral imaging cameras cover wavelengths from visible to the near-infrared (400-1700 nanometres). This gives the system the information it needs to identify insects, wood, plastic, and organic matter – all of which of which can be similar in texture, colour and shape to the food products themselves, and thus very hard for human eyes to distinguish. The system also uses advanced machine learning techniques to help recognize contaminants. High-speed learning models can provide rapid answers in real time, making it possible to detect foreign objects within the production line. More advanced convolutional neural networks and deep learning techniques can provide more detailed analysis and qualitative assessments.
From lab to food processing plant
The challenge of foreign-object detection varies somewhat across different food industry sectors. For example, ImpactVision is working with the largest sugar processor in Mexico, Beta San Miguel, to introduce our hyperspectral imaging technology into their operations. One of the hurdles we had to overcome in that case involved mitigating the effects of sugar dust on our system. We are also developing applications for products such as leafy greens and potatoes, where producers have strict wash-down requirements for their machinery, and for spices, where dust is a key issue. Another big challenge will be to detect foreign objects hidden underneath opaque foods such as raisins and other dried fruits.
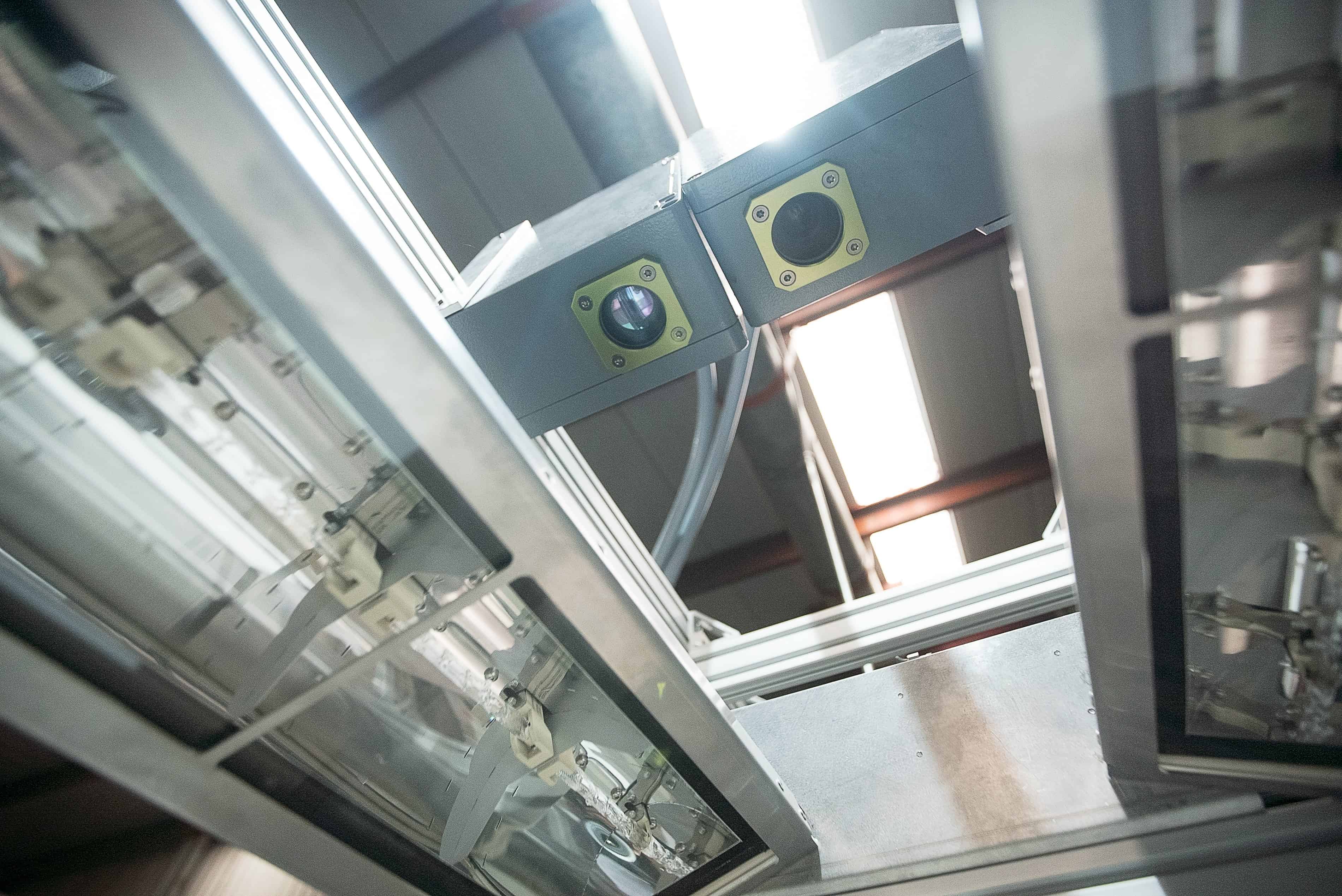
Irrespective of the type of foodstuff involved, however, there are some common hurdles associated with taking a relatively new, lab-based technology and turning it into a commercially viable product that delivers benefits for the food industry. One hurdle is that hyperspectral images are enormous. Whereas a full-resolution colour image has three pieces of data per pixel, a hyperspectral image may have over 200 spectral values associated with each pixel, meaning that a single image file takes up 1 GB of storage space. This means that image processing must happen locally in order to deliver real-time information on production lines that move at speeds of 0.75 m/s and up.
Another hurdle was that certain elements on our equipment, such as camera casings and enclosures, needed to conform to strict US Food and Drug Administration (FDA) regulations before we could use them in a real food-production facility. We have done initial integration work with a major manufacturer of programmable logic controllers, Allen-Bradley PLC, to ensure that our systems are fully integrated with the industrial automation systems used to control equipment on food-processing lines.
Replacing visual inspection and destructive tests
Margins in the food industry are slim, and manufacturers are often slow to adopt new technologies. For companies like ours, it is therefore important to develop solutions to the most urgent, expensive problems first. In March 2019, we conducted market research with produce companies based in California and other US states. Along with foreign-object detection, all the companies we interviewed ranked quality improvements among their highest priorities. The products we are currently developing, therefore, include systems that can predict the shelf life of leafy green vegetables and analyse the ripeness of avocados without needing to remove them from the production line.
For produce companies, an extra day of shelf-life – combined with a greater ability to predict and communicate how long that shelf-life is – would be a unique selling point and a way to differentiate their brands. For supermarkets, understanding shelf-life more accurately would enable them to adapt their sell-by dates accordingly. At the moment, grocery retailers manage their inventory on a first-in, first-out basis. More accurate shelf-life readings could change this (especially if integrated with other automation technologies), promoting efficient distribution and reducing waste both in-store and at home. Meal kit delivery companies are another interesting and rapidly growing market for shelf-life prediction. For these firms, a key factor driving the need for more information is a desire to increase product quality, so that they can deliver consistent produce to consumers and reduce the number of complaints.
As for avocados, the US avocado industry alone is worth $1.26bn in retail sales annually, and the price of avocados has more than doubled in the last year – meaning that waste is even more costly. For avocado processors, distributors and exporters, uneven ripening (which affects the product’s consistency as well as its taste) is a major source of waste and cost. Hyperspectral imaging is an attractive solution because it enables distributors and ripening facilities to obtain accurate readings of “dry matter” content (the easiest of the reasonably accurate fruit maturity tests) non-invasively for every avocado on the line. This means they can sort and ripen the fruit more precisely. As one avocado distributor noted when we spoke, “Everyone in the industry is dreaming of ways to evaluate maturity non-invasively and predict fruit quality more accurately.”
Universal quality assurance
In many ways, detecting foreign objects and evaluating avocado ripeness represents the tip of the iceberg for hyperspectral imaging in the food industry. Knowing the quality of 100% of products early in the supply chain will also influence the way decisions are made about their ultimate use. Potato processors, for example, might use hyperspectral imaging data to sort potatoes into those best suited for potato mash and other value-added products, and those that should be sold whole. For shipping and logistics providers (such as Maersk, our partner and investor), objective pre-export data on food quality could feed into models of how much damage is likely to occur during shipment. This could, in turn, have a dramatic influence on the way goods are stored and transported, ensuring that they reach market in optimum condition with as little waste as possible.
At all stages along the supply chain, from farm to fork, non-invasive, continuous quality data will help businesses promote food security and efficiency, while ensuring that all stakeholders have an objective, time-stamped record of quality. Ultimately, we believe that technological innovations – alongside effective regulation, consumer awareness campaigns and greater standardization in how quality is defined – are all part of the solution to the trillion-dollar problem of food waste.