
Language decoding involves recording a person’s brain activity and using this to predict the words that they were hearing, saying or thinking. Ultimately, such a system could help restore communication to those who are unable to physically speak.
That’s the goal of researchers at The University of Texas at Austin, who have created a decoder that reconstructs continuous language from non-invasive functional MRI (fMRI) data. “Eventually we hope that this technology could help people who have lost the ability to speak, due to injuries like strokes or diseases like ALS,” explained first author Jerry Tang at a press briefing.
Current systems for decoding speech from brain recordings are based on brain–computer interfaces that require invasive neurosurgery to implant. Previous non-invasive approaches, meanwhile, typically only decode single words or short phrases. The new fMRI-based model, described in Nature Neuroscience, can decode continuous language for extended periods of time.
“We were kind of shocked that this worked as well as it does,” said Alexander Huth, who co-led the study with Tang. “It was a long time coming and it was exciting when it finally did work.”
Extensive training
The key to this success, explained Huth, lies in the creation of bigger and better training datasets, combined with use of a neural network-based language model for feature extraction.
To train the decoder, the researchers recorded fMRI data from volunteers while they listened to 16 h of narrated stories. They then used these fMRI datasets to create user-specific decoding models. Each model was trained by extracting semantic features that capture the meaning of phrases, and modelling how these features influence brain response.
The researchers then tested the individual decoders on a participant’s brain responses as they listened to new stories. Based on the resulting fMRI data, the decoder outputs words as predictions of what the user is hearing. They found that the decoder could generate intelligible word sequences that captured the meanings of the new stories, as well as reproducing some exact words and phrases.
They note that the system does not precisely replicate the original words, but rather recovers the gist, for example, interpreting “I don’t have my driver’s license yet” as “she has not even started to learn to drive”.
The decoder creates these paraphrases due to way that fMRI works, by recording the blood-oxygen-level-dependent (BOLD) signal. “Functional MRI does not measure the firing of neurons, it measures changes in blood flow and blood oxygenation in the brain, which are a noisy, sluggish proxy for neural activity,” Huth explained.
Following an impulse of neural activity, the BOLD signal rises and falls over approximately 10 s, thus it is not responding to a single word but a few seconds of activity. This means that each brain image can be affected by over 20 words. “We can’t recover the exact words with this approach because we always see words mashed together. But we are able to disentangle that and we can recover the overall idea,” Huth added.

The team also ran the decoder while users imagined telling stories or watched silent movies. In both cases it successfully recovered the gist of what they were imagining or seeing. When subjects watched silent movies, for example, the decoded sequences accurately described events from the films. Comparing the decoded word sequences to descriptions of the films for the visually impaired revealed that that they were significantly more similar than expected by chance.
Privacy protection
Finally, the researchers addressed the issue of privacy and potential misuse of the technology. “We believe that nobody’s brain should be decoded without their co-operation,” stated Tang. A privacy analysis revealed that a decoder trained for one individual did not work when used with another person, and that a participant must willingly participate in the decoder training.
Users were also able to “sabotage” the decoding by performing a separate task while listening to a story in the scanner. Tasks such as naming animals or telling a story silently in their head prevented the decoder from recovering information about the story that they were listening to. “Of course, this could all change as technology gets better, so we believe it’s important to keep researching the privacy implications of brain decoding and enact policies that protect a person’s mental privacy,” said Tang.
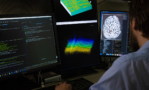
Brain implant allows person with complete paralysis to communicate
Another potential obstacle with the use of fMRI for language decoding is its reliance on access to an MRI scanner, making it unsuitable for practical applications outside of the lab.
“We think of this work as a proof-of-concept that we can decode language from non-invasive recordings,” explained Tang. “Moving forward, we want to see if our approach works with recordings from cheaper or more portable devices like electroencephalography, magnetoencephalography and functional near-infrared spectroscopy (fNIRS).”
Of these three, fNIRS is the most similar to fMRI, measuring the same BOLD signal in the brain but with lower spatial resolution. To assess its potential, the researchers simulated fNIRS data by blurring fMRI data. They found that the decoding performance degraded, but not to zero, and suggest that it should be plausible to apply their model to fNIRS data. “We are testing this out and are very excited about this approach in particular,” said Huth.