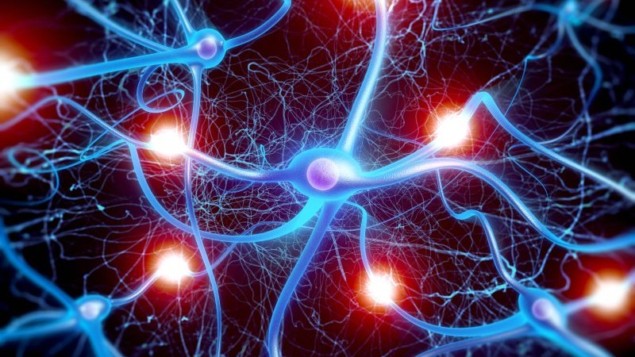
Using a strategy that mimics the encoding of information in our brains, a trio of researchers in China has proposed a new platform for artificial intelligence (AI) that could be far more robust than existing architectures. The approach, which has yet to be implemented in the lab, exploits the inevitable non-uniformity of artificial neurons that are a result of defects in real magnetic materials.
The research was done by Zhe Yuan, Ya Qiao and Yajun Zhang at the Center for Advanced Quantum Studies and Department of Physics at Beijing Normal University.
So far, the latest advances in artificial intelligence (AI) have mainly been achieved using conventional digital-computer hardware. However, it is becoming apparent that conventional silicon devices are not ideal for AI and researchers are developing neuromorphic architectures that mimic the structure and function of the human brain. These systems promise to boost both the computing speed and energy efficiency of AI systems.
Mimicking synapses
The underlying architecture of some neuromorphic computing systems involves tiny memory storage devices – which stand in for the neurons in our brains. These are linked together by memristors – which change their resistance in response to the current flowing through them. This allows them to mimic the synapses responsible for conveying electrical signals between neurons. These signals change in strength over time as information is learned and lost.
One challenge in creating neuromorphic computing systems with existing fabrication and micromachining techniques is that it can be difficult to ensure uniformity of the component devices. This means that their performance can vary widely. If this variation is too great, it can severely limit the accuracy of the overall system.
However, Yuan points out that this non-uniformity is not necessarily a problem – so long as the right approach is taken. “We certainly do not have identical cells in our brain, and there are also random processes in the brains’ neural dynamics,” he says. “Nevertheless, people can still perform precise tasks of cognition and movement without much difficulty.”
Population coding
In their study, Yuan and colleagues explored a promising strategy for reproducing this natural variation. Called “population coding”, this approach represents information in the collective activity of a population of neurons, rather than in individual cells.
As Yuan explains, this scenario actually mimics the brain’s function more closely than systems of identical neurons. “In the brain, information about positions, directions, colours, and other continuous variables are usually encoded by a group of cells,” he says. “In this way, the information is hardly influenced by noise of individual cells.”
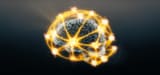
Quantum memristor paves the way for neuromorphic quantum computing
By implementing population coding, the researchers calculated how the memristor synapses linking artificial neurons can be implemented using domain walls. These are structures found in ferromagnetic materials that mark the boundaries between regions of different magnetic orientation. The location of a domain wall in a material can be shifted by passing an electrical current through the material – forming the basis for a domain-wall synapse memristor.
The trio has calculated that by using such domain-wall memristors, the non-uniformity of component devices can be an advantage. “We demonstrate that even employing these highly nonuniform devices, applying a population coding strategy can significantly improve the performance of a neural network,” Yuan explains.
The research suggests that using population coding to exploit domain-wall synapses offers a route towards more robust neuromorphic computing systems, with performances comparable to those of more traditional digital computers.
Yuan and team also believe that their approach can also be applied to other types of neuromorphic components including resistive, phase-change, and ferroelectric devices.
The research is described in New Journal of Physics.