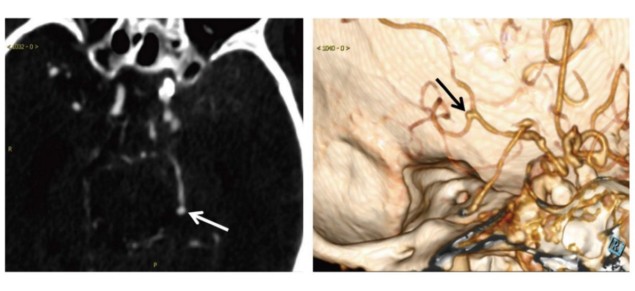
Researchers in China have developed a deep-learning-based algorithm that could help radiologists detect potentially life-threatening cerebral aneurysms on CT angiography images.
Cerebral aneurysms are weak spots in blood vessels in the brain, which can balloon out and fill with blood. If such a bulging aneurysm leaks or ruptures, it can cause serious symptoms and sometimes be fatal. The risk of rupture depends on the size, shape and location of the aneurysm, making detection and characterization of cerebral aneurysms vital.
CT angiography, which uses X-ray CT to visualize blood vessels following injection of contrast into the bloodstream, is usually the first-line imaging exam for detecting cerebral aneurysms. But this can be a challenging task: the complexity of intracranial vessels and the small size of cerebral aneurysms means that some may be missed during an initial assessment.
As such, the researchers propose that deep learning – a type of machine learning that’s increasingly used to develop algorithms for image recognition – could enhance radiologists’ performance and reduce the number of aneurysms that are initially overlooked.
“In our daily work we are always faced with cases in which some important lesions have been missed by the human eye,” says senior author Xi Long from Tongji Medical College‘s Union Hospital, in a press statement. “Cerebral aneurysms are among those small lesions that may be overlooked on the routine assessment of radiological images.”
Reporting their work in Radiology, Long and colleagues developed a detection algorithm based on a convolutional neural network. To train and assess their deep-learning algorithm, they used 1068 head CT angiography images with reported cerebral aneurysms, acquired by four different scanners at two hospitals. Half of these CT angiograms, which included 688 aneurysms ranging in size from 1.2 to 45.6 mm, were used to train the algorithm. The other half, including 649 aneurysms of 1.2 to 30.8 mm in size, formed the validation dataset.
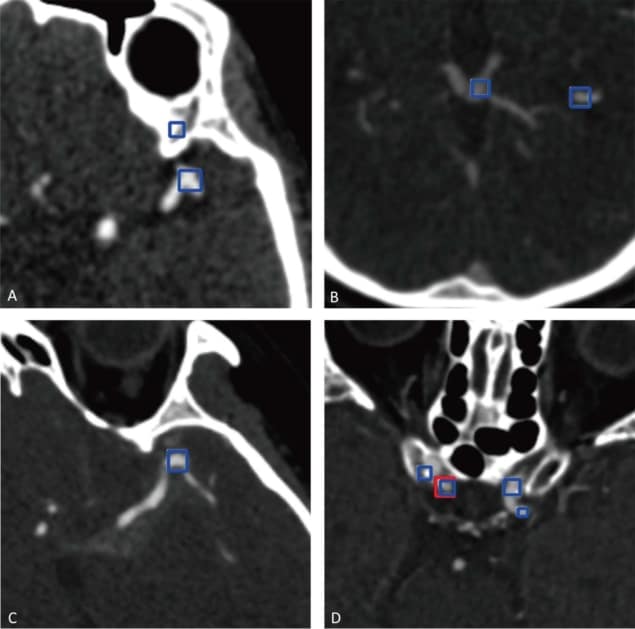
After training the algorithm, the team used it to detect cerebral aneurysms in the validation dataset. The algorithm demonstrated a maximum sensitivity of 97.5% for aneurysm detection, with a rate of 13.8 false-positive findings per case. These false-positives were observed in areas with bony structures, vessel bifurcations and curvatures, and calcified plaques. The authors note that most of these could easily be identified by radiologists.
The algorithm’s performance gradually improved in sensitivity with aneurysm size, reaching 100% in aneurysms 10 mm or larger. The lowest sensitivity was observed in aneurysms located on the anterior and posterior cerebral arteries, most of which were smaller than 3 mm. The algorithm also found eight new aneurysms, six of which were smaller than 3 mm, that had been overlooked by the human readers in the initial radiological reports.
The researchers next performed an external validation, using an additional 400 CT angiograms. Of these, 188 contained aneurysms (206, of 1 to 22 mm in size) and 212 were negative. The validation was performed by four radiologists with between one and seven years of experience in head CT angiography.
Each radiologist was randomly assigned 200 CT angiograms for interpretation with/without help from the algorithm. For each image, they recorded the type, number, location and size of any aneurysms, and the diagnosis time. Two weeks later, they interpreted the same samples again, without/with the algorithm.

Deep learning enables rapid detection of stroke-causing blockages
Assistance from the deep-learning algorithm improved the radiologists’ performance in detecting the cerebral aneurysms, increasing their overall sensitivity per lesion from 79.09% to 88.94%. The sensitivity per case was 81.63% and 91.86%, without and with the algorithm, respectively. In particular, performance was most improved for the two least experienced radiologists.
The researchers emphasize that the algorithm is intended to act as a supportive tool, providing the radiologist with a second opinion to improve their diagnostic accuracy, but not replacing the human reader.
“The deep-learning algorithm needs to be further validated on external imaging data,” Long tells Physics World. “Some improvements, such as decreasing the false positive rate, may be needed before it can be used clinically.”