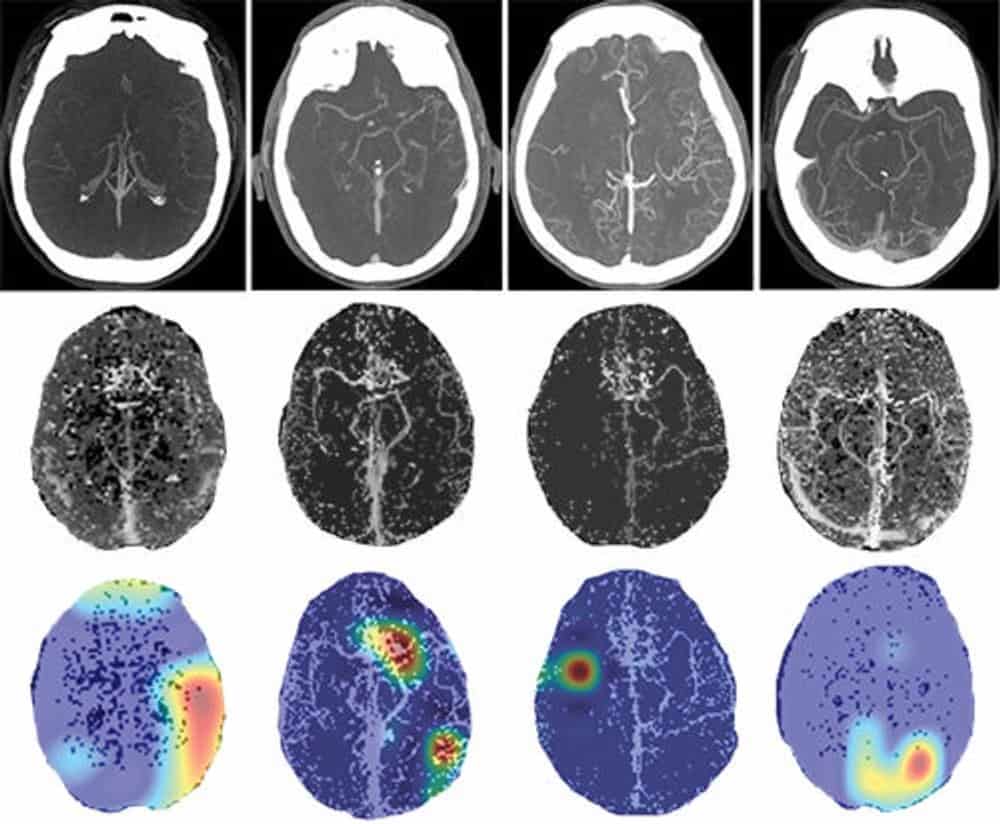
Strokes are life-threatening medical emergencies where urgent treatment is essential. They occur when part of the brain is cut off from its normal blood supply. The most common type of stroke (accounting for almost 85% of all cases) is an ischemic stroke, which is caused by a clot interrupting the supply of blood to the brain. Large vessel occlusion (LVO) strokes occur when such a blockage is found in one of the major arteries of the brain. As LVO strokes are more severe, they require immediate diagnosis and opening of the blocked artery as fast as possible.
In clinical practice, the most common method used to detect LVOs is an imaging modality called CT angiography. This method provides clinicians with a detailed, 3D image of the blood vessels in the patient’s brain. A newer CT technique, multiphase CT angiography, provides more information than its single-phase counterpart through the acquisition of cerebral angiograms in three distinct phases: peak arterial (phase 1), peak venous (phase 2) and late venous (phase 3). The main advantage of this method comes from its potential to detect any lag in filling of vessels, thus allowing the clinicians to perform a time-resolved assessment.
A group of researchers led by Ryan McTaggart from Brown University has developed a tool with the potential to quickly identify and prioritize LVO patients in an emergency setting. To achieve this, they built and trained a convolutional neural network capable of classifying the presence of LVOs on CT angiographies. This is the first study that uses deep learning to identify LVOs in both anterior and posterior arteries using multiphase CT angiography images. Their results are summarized in Radiology.
A deep-learning model that can classify LVOs…
To train, validate and test their model, the researchers used a dataset of 540 subjects with multiphase CT angiography exams. Of these, 270 patients had confirmed presence of an LVO, while the other 270 were LVO-negative. Each CT scan underwent a series of pre-processing steps.
First, the researchers standardized their scans through isotropic resampling (voxel resolution of 1 mm3), image resizing (500 x 500 pixels) and intensity normalizing (between 0 and 1). Then, they employed a vessel segmentation algorithm to increase the images’ signal-to-noise ratio. Finally, to further enhance the blood vessels, they selected 40 most-cranial axial slices from each subject and produced a single 2D image through a technique called maximal intensity projection.
To evaluate the diagnostic performance of the proposed deep-learning model, the group decided to experiment with seven training strategies. In each strategy, the team used a different subset of the multiphase CT angiography data: each phase alone, or various combinations (phases 1 and 2, phases 2 and 3, phases 1 and 3, and all three phases together).
… achieves high diagnostic performance
The group used a dataset of 62 patients (31 LVO-positive and 31 LVO-negative) to test their seven strategies. The model performed best when using all three phases as the input, where it achieved a sensitivity of 100% (31 out of 31) and a specificity of 77% (24 out of 31). Moreover, combining the peak arterial (phase 1) and late venous (phase 3), or the peak venous (phase 2) and late venous (phase 3), resulted in significantly better models than using just single-phase CT angiography.

The model gave good predictions across patient demographics, multiple institutions and scanners. Also, it detected both anterior and posterior circulation occlusions. “[Our model] could function as a tool to prioritize the review of patients with potential LVO by radiologists and clinicians in the emergency setting,” the researchers conclude. For future work, the group aims to evaluate the model’s clinical utility by testing it in a real-time emergency setting.