
Glioblastoma is the most common primary malignant brain tumour. It is aggressive and rapidly fatal, with a median survival time of only 15 months after diagnosis. Glioblastoma cells’ genetic profiles are extremely heterogenous, which is one reason for the generally poor prognosis. It also means that disease progression and response to treatment can vary greatly between patients.
A group of researchers from Case Western Reserve University has now developed a model that links radiomics features to tumour biology. This could greatly improve prognostic accuracy and help in the design of patient-specific treatments for glioblastoma (Clin. Cancer Res. 10.1158/1078-0432.CCR-19-2556).
Gene expression as a prognostic tool
In recent years, researchers have identified several characteristic genetic markers in glioblastoma that influence the disease prognosis. These markers can be used as predictors of a patient’s survival time or even to predict which treatment a patient is most likely to respond well to. Discovering a tumour’s gene profile requires a biopsy, however – an invasive procedure that carries risks of its own.
MRI provides a non-invasive way of analysing a tumour and its surroundings. Using radiomics methods, one can extract image information, so-called “features”, that are hard or impossible to detect by the naked eye. The group, led by Pallavi Tiwari, has developed a prognostic model based on pre-treatment MRI radiomics features – and, importantly, connected the features used in this model to biological processes in the tumour. In this way, the researchers not only show that their model can predict survival, but they also explain why it does.
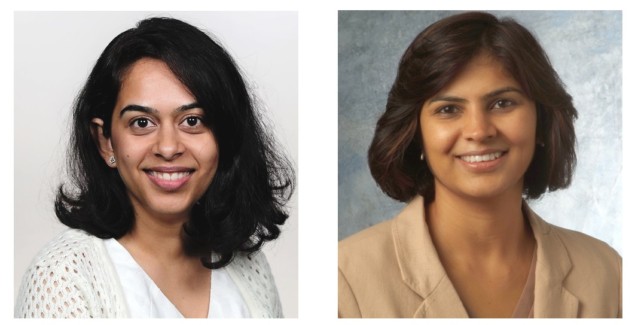
Tiwari and colleagues built their model using pre-treatment MR image sets of 130 patients. For each of these, they extracted 2850 radiomics features and finally identified 25 as being especially prognostic of a patient’s progression-free survival. These features describe image properties such as “wavy, ripple and spot-like patterns”, the authors explain, or the sphericity of the oedema (swelling) surrounding the tumour. They combined these features into a model to calculate a radiomic risk score (RRS) and validated the model’s prognostic ability in an independent patient cohort.
Tiwari’s team then showed that these radiomics features correlate with certain gene expressions in the tumour. These genes control biological processes such as the rate of cell division or the growth of new blood vessels to transport nutrients to the tumour. In this way, the researchers established a direct connection between the radiomics features and the biological processes leading to disease progression.
Towards personalized treatments
Despite being the most common primary brain cancer, glioblastoma remains a relatively rare disease. It would likely take a prohibitively long time to collect enough patients for a study such as this in a single institution. Tiwari and her colleagues circumvented this issue by using open-source data sets from The Cancer Imaging Archive and the Ivy Glioblastoma Atlas Project, in addition to data from their collaborating institution, the Cleveland Clinic. Data pooling efforts such as these are essential for building increasingly stronger predictive disease models.
In the future, the group will work on extending the image correlates found to be prognostic of overall survival and demonstrate that the RRS model can be predictive of response to chemotherapy in particular, says Tiwari. “This could have huge clinical implications in terms of identifying glioblastoma patients who may not respond favourably to chemotherapy and may be more suited for genomically-driven treatments to improve their quality of life as well as overall survival times,” she explains.