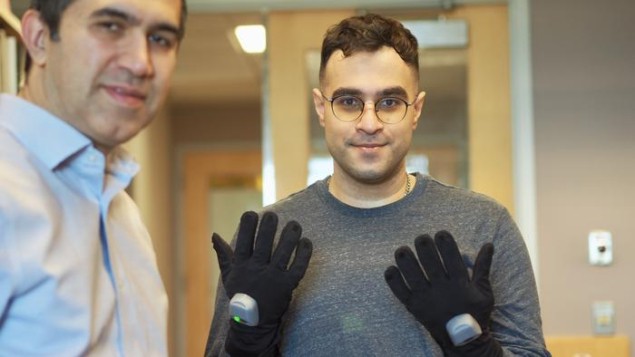
A smart glove that tracks finger, hand, and wrist movements with unprecedented accuracy has been developed by researchers at Canada’s University of British Columbia (UBC) and Texavie Technologies. The washable device is embedded with individual sensor fibres that respond to tiny changes in the material’s stretch and pressure. The sensors wirelessly transmit this information into a machine learning algorithm that estimates the hand’s fine scale movements almost immediately.
In addition to remotely capturing dynamics and providing information about how hands interact with objects for robotics and virtual reality, the glove offers a tool for assessing stroke and other patients’ hand movements and grasping forces. Such assessments can help patients to receive feedback on what movements they need to focus on to improve their hand mobility function.
In the new design, created by Peyman Servati and his team, numerous custom-made fibre sensors are sewn into the glove’s stretchable fabric at points overlying the finger joints, fingertips, wrist, and palm. Movements in the joints, or pressure caused by the hand interacting with an object, create stretches in the fabric. The sensors can detect stretches as low as 0.005% and as high as 155% of their original length. All of these sensors, linked via stretchy connectors to a wireless processing board on the back of the glove, feed data into an algorithm that estimates the joint angles with an accuracy of 1.4°. The output is a 3D image of the hand’s shape that dynamically follows the glove wearer’s movements.
Weaving good yarns
Servati and his colleagues developed special fibres called helical sensor yarns, which improve the performance accuracy of materials used in wearable textile sensors. These stretchable yarns consist of an elastic core wrapped with metal-coated nanofibres in helical form. A polymer matrix and elastomer shell bind the structure together, providing durability, dynamic range, and tensile strength. External stretching/pressure-releasing cycles change the contact area of the bound-together metallic nanofibres, resulting in changes in their electrical resistance. These yarns were sewn between two layers of nylon-polyester-spandex to make the smart gloves.
Using motion-capture camera systems, the researchers collected more than three million frames of hand movement by five participants with different hand sizes. They were wearing smart gloves that were marked with visible labels at 16 points. The participants grasped objects, switched between gestures, and randomly moved their fingers. A neural-network architecture mapped visible images to simultaneously collected sensor data, resulting in a machine learning model that estimated hand joint angles and tactile information from the strain data measured by the sensor yarns.
“Capturing dexterous hand and finger movements with accuracy is a very hard task. Current camera-based systems are costly and have issues with limited field of view,” says Servati. The glove is the most accurate design in the market for estimating the angles of fingers and wrist during movement with minimal delay. It matches the accuracy of gold-standard camera equipment.
Subjects wearing the glove also tested how the glove performed at capturing specific movements related to everyday tasks. The device was able to detect words “typed” by multi-finger movements on a random surface with 98% accuracy; it estimated 100 static and dynamic gestures adapted from American Sign Language with 95% accuracy. It also detected 34 objects – including mugs, glasses, baseballs, and tennis balls – from the hand’s grasp shape and forces with 98% accuracy.
Rinse, wash, repeat
One use of the glove could be to aid stroke and patients who have lost partial hand mobility. Working with clinical experts including Janice Eng, who specializes in stroke rehabilitation at the UBC Department of Medicine, Servati and his team found that many patients require an accurate way to assess their hand movements and grasp forces. Performing these assessments remotely and modifying exercise routines or evaluating compliance could also help patients with Parkinson’s and other hand mobility issues.
“This is very hard to do even in clinic, and nothing exists to do it accurately and remotely,” says Servati.

Sensor-covered glove could lead to better prosthetic hands
Wearable devices are attractive for clinical tasks, but many designs lack the reliability, accuracy, and washability that are needed for practical use. After repeated soaking and stirring in water and detergents, and after undergoing repeated machine laundering cycles, Servati’s glove experienced less than 10% change in sensor performance.
“It is really exciting to develop this technology in a durable and washable form that can create a major leap in human-computer interaction and the possibility to accurately represent interaction with objects without the need for camera,” says Servati.
Subramanian Sundaram, a researcher in Boston University’s Biological Design Center, who was not involved in the study, says that studying how these fibres’ functionality changes under daily-use conditions is the “right direction to focus on” for creating reliable textiles that people can use repeatedly. Although the quantitative error estimates of joint angles are important considerations for potential medical applications, he believes that such applications are still a long way off. “The key challenge, not unique to this work, is determining the specific settings where this type of technology is critically needed,” he says.
The work is described in Nature Machine Intelligence.